Collective Unconscious#
Generic#
“Tikkun Olam” (תיקון עולם): “repairing the world” or “mending the world.” It’s a concept in Judaism that emphasizes social justice, ethical behavior, and actions that contribute to the betterment of society and the world as a whole. In You Are So Not Invited to My Bat Mitzvah, the term “Tikkun Olam” is mentioned in a class session, and then it transitions into the playful song God Is Random.
By bringing up “Tikkun Olam,” the film nods to a serious
, thoughtful Jewish concept about making the world a better place. Then, it follows this with the whimsical
song “God Is Random,” which introduces a contrasting, humorous take on divine order
(or the lack thereof). This contrast highlights the tension between the deep, philosophical ideas in religious traditions and the randomness and unpredictability
of real life, all wrapped up in the lighthearted context of a Bat Mitzvah.
This blend of profound themes with humor likely adds a layer of irony or commentary about how we reconcile serious beliefs with the seemingly random events of life. It’s a clever way to reflect the complexities of faith, especially in a coming-of-age story.
Specific#
Rabbi
. We’re going to be talking about Tikkun Olam. Does anyone know what that means? Kim! If God exists, then how do you explain climate change? That’s actually an amazing question. Yeah, and why can’t straight people get on gay TikTok? It’s like way funnier. And if God loves me, then why does he always give me so many zits? And why does Apple keep changing their chargers? And why did my dad’s doctor operate on the wrong shoulder? And why do I have to share a room with my grandma? Okay, okay, okay. I think I can explain zits and climate change and gay TikTok. Musically! If God is real, then why is there war? Why is there injustice? Why are people poor? And why have I had a yeast infection for a solid 11 years? The answer is clear. God is random. God is random. God is random. Everybody! God is random. God is random. God is random.GPT
. It sounds like you’re channeling a scene that mixesexistential
questions, humorous modern dilemmas, and a bit ofabsurdity
, all wrapped up in a playful, musical style. This blend of philosophical inquiry and comedy seems like it’s from a contemporary piece, perhapssatirizing
the way we look for answers to life’s big questions in all the wrong places—or maybe how we try to make sense of randomness and chaos in our everyday lives.
The idea that “God is random” plays with the concept of divine inscrutability, suggesting that life’s unpredictability could be seen as evidence of a capricious higher power—or maybe just the absence of any order at all. The randomness becomes a metaphor for life’s inexplicable injustices and inconveniences, from the grand scale of war and climate change to the personal annoyances of zits and incompatible chargers.
If this is from a specific work or if you’re developing a scene, it’s capturing that modern sense of disillusionment mixed with humor, where the existential is met with a shrug and a song. It’s a clever way to highlight the absurdity and randomness of life’s questions while also suggesting that perhaps the answers—or the lack thereof—don’t really matter as much as we think. Would you like to delve deeper into any of these themes or how they could be developed further?
1. Outside World
\
2. Heritage -> 4. Collective Unconscious -> 5. Teachers-High.priests-Heroes-Producers-Decoders -> Ego
/
3. Symbols & Archetypes
Fig. 13 Fruitful exchange with Duncan. He shared Azawi’s “Masavu” & was perplexed at how much it taken a hold of him. On listening to it, I immediately recognized the enchanting chord progression & dynamics of Chi-Lites “Have you seen her”. When you go beneath the superficial difference between these two songs, you’ll unconver their essence: the archetypal chord progression ii-V7-I
& emotional arc “something about a girl”#
Biological 1, 2, 3#
Voir: Aware
Savoir: Compute
Pouvoir: Bayesian
Personal 5, 6#
Flow, Plugin
Growth
also… conducted amongst researchers list the following reasons:
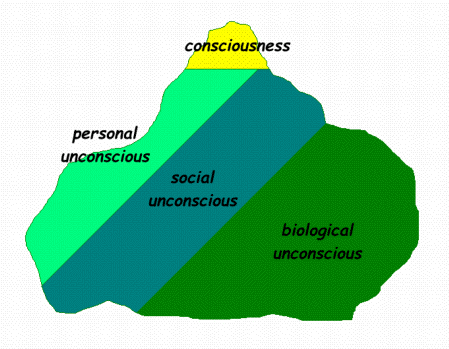
Fig. 14 Jung was onto something#
1. Food-Physical
\
2. Teeth-Natural.Language -> 4. Enzymes-Coded.Language -> 5. Absorption-Plugins -> Growth-Liberation
/
3. Gut-Tokens
Social 4#
Agency: Social