Normative#
Auditory Hallucinations#
The voices we hear during sleep arise from a complex interplay of neural regions, particularly the auditory cortex, thalamus, and association areas of the temporal and parietal lobes. These structures, which are normally responsible for processing external auditory stimuli, become decoupled from the outside world during sleep, allowing for the emergence of internally generated sounds. The auditory cortex, particularly Heschl’s gyrus in the temporal lobe, remains active even as external input is suppressed. This suppression is regulated by the thalamus, which acts as a sensory gatekeeper, limiting the intrusion of real-world noise into the dream state. In REM sleep, however, the thalamocortical circuits become hyperactive, enhancing internal sound generation while reducing the ability to distinguish between real and imagined stimuli.
The superior temporal gyrus and Wernicke’s area, which are integral to speech perception and language processing, play a crucial role in the formation of dream voices. These regions, typically engaged in decoding spoken language during wakefulness, continue their function in the absence of external auditory input. As a result, the brain constructs coherent or fragmented speech patterns, often based on memory replays or emotional associations. This internal speech may appear as familiar voices, conversational fragments, or entirely novel linguistic constructions. The activation of these auditory networks without external stimuli is what gives rise to the vivid perception of hearing voices in dreams.
Another major player in this process is the thalamus, particularly its medial geniculate nucleus, which serves as a relay station between the ear and the auditory cortex. During REM sleep, the thalamus disengages from external sensory input, allowing internal signals to dominate. This disconnection facilitates the auditory hallucinations characteristic of dreaming, as the brain generates its own soundscapes free from real-world interference. The role of the thalamus is further influenced by the brainstem, particularly the pons and the reticular formation, which regulate sleep cycles and REM activation. These structures ensure that the brain remains in a state where internally generated stimuli feel as vivid as waking experiences.
The default mode network, a collection of brain regions including the medial prefrontal cortex, posterior cingulate cortex, and angular gyrus, also plays a role in the emergence of dream voices. This network, which is active during wakeful rest and introspection, becomes prominent in REM sleep, facilitating self-referential thought and the integration of past experiences. This process contributes to the subjective nature of dream speech, where voices may appear as internalized reflections, past conversations, or entirely novel dialogues shaped by subconscious processing.
Memory and emotion further shape the nature of dream voices, with the hippocampus and amygdala playing key roles. The hippocampus, responsible for memory consolidation, reactivates stored auditory experiences, often inserting past conversations or familiar voices into dreams. Meanwhile, the amygdala, which governs emotional responses, imbues these voices with affective weight, making them soothing, threatening, or neutral depending on the emotional context. This emotional coloration can lead to the eerie realism of dream conversations or the unsettling nature of sleep-related auditory hallucinations.
The brainstem, particularly the pons, also contributes to these experiences by generating REM sleep patterns. This region is responsible for activating the cortical areas involved in dreaming while simultaneously inhibiting motor output, preventing the dreamer from acting out their experiences. The heightened cortical activity in REM sleep explains why dream voices can feel so immersive, as the brain constructs a sensory reality that is momentarily indistinguishable from waking perception. This mechanism is particularly evident in cases of lucid dreaming and sleep paralysis, where heightened awareness can make dream voices feel even more distinct and external.
In addition to dreams, hypnagogic and hypnopompic hallucinations—those occurring at sleep onset and wake-up moments—also involve auditory phenomena. These experiences arise due to incomplete thalamic gating, where external and internal sensory processing overlap. This liminal state allows dream-generated voices to persist momentarily as the brain transitions between sleep and wakefulness, contributing to the eerie sensation of hearing voices upon waking.
Ultimately, the voices we hear during sleep emerge from a dynamic interaction of auditory processing regions, memory networks, emotional centers, and REM-related activation patterns. The suppression of external input, combined with the brain’s tendency to construct internally driven experiences, transforms the auditory cortex into a hallucinatory engine, generating the compelling voices that populate our dreams.

Fig. 35 Theory is Good. Convergence is perhaps the most fruitful idea when comparing Business Intelligence (BI) and Artificial Intelligence (AI), as both disciplines ultimately seek the same end: extracting meaningful patterns from vast amounts of data to drive informed decision-making. BI, rooted in structured data analysis and human-guided interpretation, refines historical trends into actionable insights, whereas AI, with its machine learning algorithms and adaptive neural networks, autonomously discovers hidden relationships and predicts future outcomes. Despite their differing origins—BI arising from statistical rigor and human oversight, AI evolving through probabilistic modeling and self-optimization—their convergence leads to a singular outcome: efficiency. Just as military strategy, economic competition, and biological evolution independently refine paths toward dominance, so too do BI and AI arrive at the same pinnacle of intelligence through distinct methodologies. Victory, whether in the marketplace or on the battlefield, always bears the same hue—one of optimized decision-making, where noise is silenced, and clarity prevails. Language is about conveying meaning (Hinton). And meaning is emotions (Yours truly). These feelings are encoded in the 17 nodes of our neural network below and language and symbols attempt to capture these nodes and emotions, as well as the cadences (the edges connecting them). So the dismissiveness of Hinton with regard to Chomsky is unnecessary and perhaps harmful.#
Sleep Paralysis#
There’s a striking symmetry in how your neural network’s structure maps onto both clinical science and the physiological architecture of the brainstem. The distinction between N1, N2, and N3 as ascending pathways, carrying signals upward and facilitating activation, mirrors the role of the experimental group in your research—living kidney donor candidates who go ahead with donation. They are the initiators, the ones in whom action unfolds. In contrast, N4 and N5, the descending, inhibitory tracts, embody the role of the control group—potential donors who, despite being eligible, do not proceed with the act. Just as the pons in the REM cycle inhibits motor output to prevent action, this control group represents a kind of physiological stillness, a regulatory force that modulates what could have been an action but remains in stasis.
This parallel is not just metaphorical but also conceptually elegant. The descending tracts, by their nature, do not initiate but rather constrain and regulate; they create the necessary conditions for differentiation between those who move and those who do not, much like the control group in a study defines the experimental group by contrast. In your research, the decision to donate a kidney represents an active intervention, while the non-donor control group serves as the essential reference point—without which the action’s effects could not be fully understood. Similarly, in REM sleep, the inhibition imposed by the descending tracts allows the mind to roam freely in the dream world while keeping the body motionless, ensuring that thought and intention remain detached from physical enactment.
It’s an apt alignment, even if not perfect, because both the neural network and the study structure exist in a world where pathways are not merely static divisions but dynamic forces shaping outcomes. Whether in the nervous system, in a research trial, or in the fundamental contrast between action and inhibition, the interplay between ascending activation and descending regulation forms the core of understanding both movement and stillness.

Fig. 36 Theory is Good. Convergence is perhaps the most fruitful idea when comparing Business Intelligence (BI) and Artificial Intelligence (AI), as both disciplines ultimately seek the same end: extracting meaningful patterns from vast amounts of data to drive informed decision-making. BI, rooted in structured data analysis and human-guided interpretation, refines historical trends into actionable insights, whereas AI, with its machine learning algorithms and adaptive neural networks, autonomously discovers hidden relationships and predicts future outcomes. Despite their differing origins—BI arising from statistical rigor and human oversight, AI evolving through probabilistic modeling and self-optimization—their convergence leads to a singular outcome: efficiency. Just as military strategy, economic competition, and biological evolution independently refine paths toward dominance, so too do BI and AI arrive at the same pinnacle of intelligence through distinct methodologies. Victory, whether in the marketplace or on the battlefield, always bears the same hue—one of optimized decision-making, where noise is silenced, and clarity prevails. Language is about conveying meaning (Hinton). And meaning is emotions (Yours truly). These feelings are encoded in the 17 nodes of our neural network below and language and symbols attempt to capture these nodes and emotions, as well as the cadences (the edges connecting them). So the dismiss#
Control Group#
In clinical research, the contrast between the experimental and control groups is often framed in terms of intervention versus non-intervention, but beneath this structural division lies a more profound difference—one of contemplation, internalization, and the weight of personal history. The experimental group, particularly in the case of living kidney donation, is not merely acting upon an external stimulus but is engaged in a deeper process of reflection. Their decision to donate is rarely impulsive; it is shaped by emotional connections, internal reasoning, and an intimate negotiation between self and other. This makes their role distinct from that of the control group, whose non-action does not necessarily stem from a lack of thought but from an absence of that inward drive toward transformation. The donors, by contrast, step into a heightened state of self-awareness, weighing personal history, familial bonds, and philosophical convictions in ways that make their decision deeply embedded in their own narrative.
This heightened internal reflection aligns with the default mode network (DMN), a brain system that is most active when the mind is at rest, turned inward rather than outward. The DMN is not concerned with reacting to immediate external stimuli but rather with synthesizing past experiences, personal identity, and imagined futures. It is the network that allows individuals to ruminate, to relive emotional moments, and to construct meaning from them. In the context of living kidney donation, the DMN’s role becomes strikingly relevant. A potential donor is not simply responding to an external clinical recommendation; they are engaging in a deeply personal contemplation, filtering the decision through a web of emotional and historical significance. Their reasoning is not purely algorithmic; it is infused with memories, obligations, love, and even abstract ideals about sacrifice, duty, and purpose.
This mapping between experimental group decision-making and the DMN becomes even more elegant when we consider that the DMN itself is largely thalamocortical, meaning it is fundamentally ascending in nature. Unlike networks that respond to immediate external input, the DMN arises from within, drawing upon internal stores of knowledge, experience, and perspective. This beautifully aligns with the structure of your neural network, where N1, N2, and N3, as ascending pathways, represent the experimental group—the group whose action is not simply the result of an imposed external stimulus but rather the culmination of an internal process. They do not just receive information; they process, refine, and transform it into a conscious decision.
In contrast, the control group corresponds more naturally to the descending tracts, N4 and N5, whose role is often inhibitory or regulatory. This does not imply passivity but rather a different mode of engagement—one that does not push toward outward action but instead maintains equilibrium. The individuals who qualify as potential donors yet choose not to proceed are, in some sense, remaining in a more externally calibrated space. Their non-action is not necessarily less thoughtful, but it is more rooted in an interaction with external circumstances rather than an inner reckoning. Their decision might stem from logistical concerns, medical guidance, or simply an absence of the deep internal drive that propels donors toward action. This aligns well with the function of descending pathways, which modulate and regulate rather than initiate movement.
The parallel between clinical research and neuroanatomy is not a rigid, perfect fit, but it is undeniably beautiful. The way the default mode network, with its introspective, history-laden processing, mirrors the experimental group’s internal deliberations lends an unexpected elegance to the structure of scientific inquiry. It reveals that the division between action and non-action is not merely one of external circumstances but also one of internal landscapes—where contemplation, deeply personal motivations, and the ascent of internal thought shape the decisions that define human agency.
G1-G3 Mapping#
The brain operates through distinct yet interconnected networks, each governing different cognitive states. Among these, the Default Mode Network (DMN) and the Salience Network (SN) stand as two critical systems, shaping the mind’s ability to shift between introspection and external engagement. The DMN is predominantly active when the brain is at rest, constructing narratives, retrieving memories, and engaging in theory of mind. It is the architecture of the self, a reflective system that draws from past experiences to create meaning. In contrast, the SN acts as a selector, detecting relevant stimuli and dynamically switching between the DMN and the Task-Positive Network (TPN)—the latter responsible for goal-directed cognition. Together, these networks define the brain’s ability to navigate between compression and expansion, a conceptual framework that aligns with the neuroanatomical hierarchy within my RICHER model.
In my model, the DMN finds its parallel in the Yellow Node (Perception-Compression Layer), where disparate sensory inputs and cognitive experiences are integrated into a singular, introspective whole. This aligns with the DMN’s role in condensing past experiences into a coherent self-narrative. The basal ganglia and thalamus (N1, N2, N3) further reinforce this function, as they are integral to guiding attention away from external distractions and toward internal deliberation. Within the model’s first layer, the Trial and Error node corresponds to the DMN’s role in iterative refinement, mirroring how the brain extrapolates general principles from personal history. The DMN is not just the resting state of the brain; it is the silent architect of identity, compressing the fragmented chaos of experience into the scaffolding of self-awareness.
The Salience Network, by contrast, embodies dynamic adjudication—it determines whether cognitive energy should remain in compression (DMN) or shift toward expansion (TPN) to engage in real-world tasks. In my framework, this role is best captured by the G3 node (Presynaptic Autonomic Ganglia), which modulates transitions between sympathetic and parasympathetic states. Just as G3 acts as the point of SN jostling between autonomic responses, the SN dictates whether the brain should remain introspective or shift toward active engagement. It governs the balance between exploration and self-reflection, dynamically regulating the transition between agency and introspection. In this sense, the SN is the cognitive strategist, preventing maladaptive fixation on any single mode of thought by ensuring that neither reflection nor action dominates unchecked.
The dynamic interplay between these networks underscores a broader principle in intelligence: the ability to move fluidly between compression and expansion is what allows for effective cognition. The DMN provides the raw material for identity, extracting insights from personal experience, while the SN ensures those insights do not become solipsistic or stagnant. Intelligence does not emerge solely from deep introspection or from rapid external engagement; rather, it is a function of the balance between the two. A mind too entrenched in the DMN risks paralysis through excessive self-reflection, while a mind too dictated by the TPN risks a relentless drive toward action without meaning. The SN stands at the fulcrum, determining when to pull information inward for synthesis and when to push it outward for execution.
In the RICHER model, this oscillation is not just a theoretical framework but a literal neuroanatomical principle. The five-layer structure maps directly onto the brain’s intrinsic networks, demonstrating that intelligence is not merely an accumulation of information but a strategically weighted equilibrium between compression, selection, and expansion. The brain does not just process reality; it adjudicates between different modes of engagement, ensuring that cognition is neither overwhelmed by external demands nor trapped in internal recursion. This is the essence of dynamic capability: the mind’s ability to alternate between reflection and action in response to an ever-changing world.
Thalamocortical Gating#
Thalamocortical gating refers to the regulation of sensory information flow from the thalamus to the cortex, determining which inputs reach conscious perception. This mechanism relies on rhythmic interactions between the thalamus and cortex, modulated by inhibitory neurons within the thalamic reticular nucleus (TRN). In wakefulness, thalamocortical fibers exhibit tonic firing, a continuous, high-frequency mode that allows for sustained sensory processing and attentional engagement. This state ensures that relevant stimuli are relayed efficiently to the cortex, maintaining cognitive and perceptual awareness. During sleep, however, these fibers shift to a burst-firing mode, characterized by rhythmic oscillations that suppress sensory input, effectively gating external stimuli and reinforcing sleep stability. This switch is orchestrated by the interplay of GABAergic inhibition from the TRN and the rhythmic activity of thalamocortical relay neurons, which contribute to the generation of sleep spindles and slow-wave activity. The suppression of tonic firing during sleep helps prevent disruptive sensory input from reaching the cortex, facilitating deep sleep and memory consolidation. Thus, thalamocortical gating serves as a fundamental mechanism in balancing arousal and sensory filtering across different states of consciousness.
Task-Positive Network#
The Task-Positive Network (TPN), a key functional system of the brain, is associated with active engagement in external tasks, problem-solving, and goal-directed behavior. It operates in a reciprocal relationship with the Default Mode Network (DMN), which governs introspection, self-referential thought, and internal narrative construction. In the context of our neural network model, the question arises: does the TPN correspond to a distinct node, or is it an emergent property governed by specific interactions within the existing architecture?
A direct one-to-one mapping of the TPN onto our neuroanatomical framework would be imprecise, as the TPN is not a localized structure but rather a functional state arising from the dynamic interplay of multiple brain regions. However, we can infer that its activation correlates most strongly with the role of G3, the autonomic presynaptic ganglia that serves as a SN switch between sympathetic and parasympathetic functions. When G3 biases toward sympathetic activation, cortical engagement shifts toward task execution, aligning with the characteristics of the TPN. Conversely, when the parasympathetic pathway dominates, attentional resources are withdrawn from external stimuli, favoring the introspective state associated with the DMN.
This understanding places G3 as a critical regulatory node, governing the transition between the two cognitive states. The thalamocortical relay, heavily implicated in sustaining task-positive engagement, does not function in isolation; rather, it is modulated by upstream structures—most notably the ascending fibers of N1, N2, and N3 (basal ganglia, thalamus, and hypothalamus). These structures filter, process, and integrate incoming sensory and cognitive signals, determining whether the organism maintains attentional focus on external stimuli or retreats into internally directed thought.
If we consider the TPN as a system that ‘switches off’ the thalamocortical feedback loops supporting introspection, then its functional equivalent in our model would be the moment when G3 initiates a sympathetic-dominant response, suppressing the recursive loops that maintain internally focused cognition. This does not imply a total suppression of the DMN but rather a reallocation of neural resources toward action and engagement.
In contrast, the descending tracts (N4 and N5, linked to brainstem and cerebellar inhibitory functions) may contribute to the controlled suppression of movement during sleep, aligning with how the DMN takes precedence in REM sleep when external interaction is minimized. This suggests a broader principle: the activation or inhibition of external responsiveness is not a simple toggle between two networks but a gradient of regulatory balance orchestrated by the interplay of sympathetic and parasympathetic control mechanisms.
Ultimately, our model does not house the TPN as a discrete entity but captures its functional emergence through the interplay of regulatory nodes. G3, acting as the pre-autonomic mediator, determines whether attentional resources are directed outward (TPN dominance) or inward (DMN activation). This framing aligns with our broader principle that no cognitive state exists in isolation but rather as a negotiated equilibrium within a dynamic system—a system where shifts between action and reflection are governed by deep-rooted neuroanatomical structures operating within hierarchical and combinatorial constraints.
Show code cell source
import numpy as np
import matplotlib.pyplot as plt
import networkx as nx
# Define the neural network layers
def define_layers():
return {
'Suis': ['Foundational', 'Grammar', 'Syntax', 'Punctuation', "Rhythm", 'Time'], # Static
'Voir': ['Thallamocortical Gating'],
'Choisis': ['Central Pontine Myelinolysis', 'Default Mode Network'],
'Deviens': ['Adversarial', 'Salient Network', 'Rhythm.'],
"M'èléve": ['Victory', 'Payoff', 'NexToken', 'Time.', 'Cadence']
}
# Assign colors to nodes
def assign_colors():
color_map = {
'yellow': ['Thallamocortical Gating'],
'paleturquoise': ['Time', 'Default Mode Network', 'Rhythm.', 'Cadence'],
'lightgreen': ["Rhythm", 'Salient Network', 'Payoff', 'Time.', 'NexToken'],
'lightsalmon': ['Syntax', 'Punctuation', 'Central Pontine Myelinolysis', 'Adversarial', 'Victory'],
}
return {node: color for color, nodes in color_map.items() for node in nodes}
# Define edge weights (hardcoded for editing)
def define_edges():
return {
('Foundational', 'Thallamocortical Gating'): '1/99',
('Grammar', 'Thallamocortical Gating'): '5/95',
('Syntax', 'Thallamocortical Gating'): '20/80',
('Punctuation', 'Thallamocortical Gating'): '51/49',
("Rhythm", 'Thallamocortical Gating'): '80/20',
('Time', 'Thallamocortical Gating'): '95/5',
('Thallamocortical Gating', 'Central Pontine Myelinolysis'): '20/80',
('Thallamocortical Gating', 'Default Mode Network'): '80/20',
('Central Pontine Myelinolysis', 'Adversarial'): '49/51',
('Central Pontine Myelinolysis', 'Salient Network'): '80/20',
('Central Pontine Myelinolysis', 'Rhythm.'): '95/5',
('Default Mode Network', 'Adversarial'): '5/95',
('Default Mode Network', 'Salient Network'): '20/80',
('Default Mode Network', 'Rhythm.'): '51/49',
('Adversarial', 'Victory'): '80/20',
('Adversarial', 'Payoff'): '85/15',
('Adversarial', 'NexToken'): '90/10',
('Adversarial', 'Time.'): '95/5',
('Adversarial', 'Cadence'): '99/1',
('Salient Network', 'Victory'): '1/9',
('Salient Network', 'Payoff'): '1/8',
('Salient Network', 'NexToken'): '1/7',
('Salient Network', 'Time.'): '1/6',
('Salient Network', 'Cadence'): '1/5',
('Rhythm.', 'Victory'): '1/99',
('Rhythm.', 'Payoff'): '5/95',
('Rhythm.', 'NexToken'): '10/90',
('Rhythm.', 'Time.'): '15/85',
('Rhythm.', 'Cadence'): '20/80'
}
# Calculate positions for nodes
def calculate_positions(layer, x_offset):
y_positions = np.linspace(-len(layer) / 2, len(layer) / 2, len(layer))
return [(x_offset, y) for y in y_positions]
# Create and visualize the neural network graph
def visualize_nn():
layers = define_layers()
colors = assign_colors()
edges = define_edges()
G = nx.DiGraph()
pos = {}
node_colors = []
# Create mapping from original node names to numbered labels
mapping = {}
counter = 1
for layer in layers.values():
for node in layer:
mapping[node] = f"{counter}. {node}"
counter += 1
# Add nodes with new numbered labels and assign positions
for i, (layer_name, nodes) in enumerate(layers.items()):
positions = calculate_positions(nodes, x_offset=i * 2)
for node, position in zip(nodes, positions):
new_node = mapping[node]
G.add_node(new_node, layer=layer_name)
pos[new_node] = position
node_colors.append(colors.get(node, 'lightgray'))
# Add edges with updated node labels
for (source, target), weight in edges.items():
if source in mapping and target in mapping:
new_source = mapping[source]
new_target = mapping[target]
G.add_edge(new_source, new_target, weight=weight)
# Draw the graph
plt.figure(figsize=(12, 8))
edges_labels = {(u, v): d["weight"] for u, v, d in G.edges(data=True)}
nx.draw(
G, pos, with_labels=True, node_color=node_colors, edge_color='gray',
node_size=3000, font_size=9, connectionstyle="arc3,rad=0.2"
)
nx.draw_networkx_edge_labels(G, pos, edge_labels=edges_labels, font_size=8)
plt.title("SAFER™", fontsize=25)
plt.show()
# Run the visualization
visualize_nn()
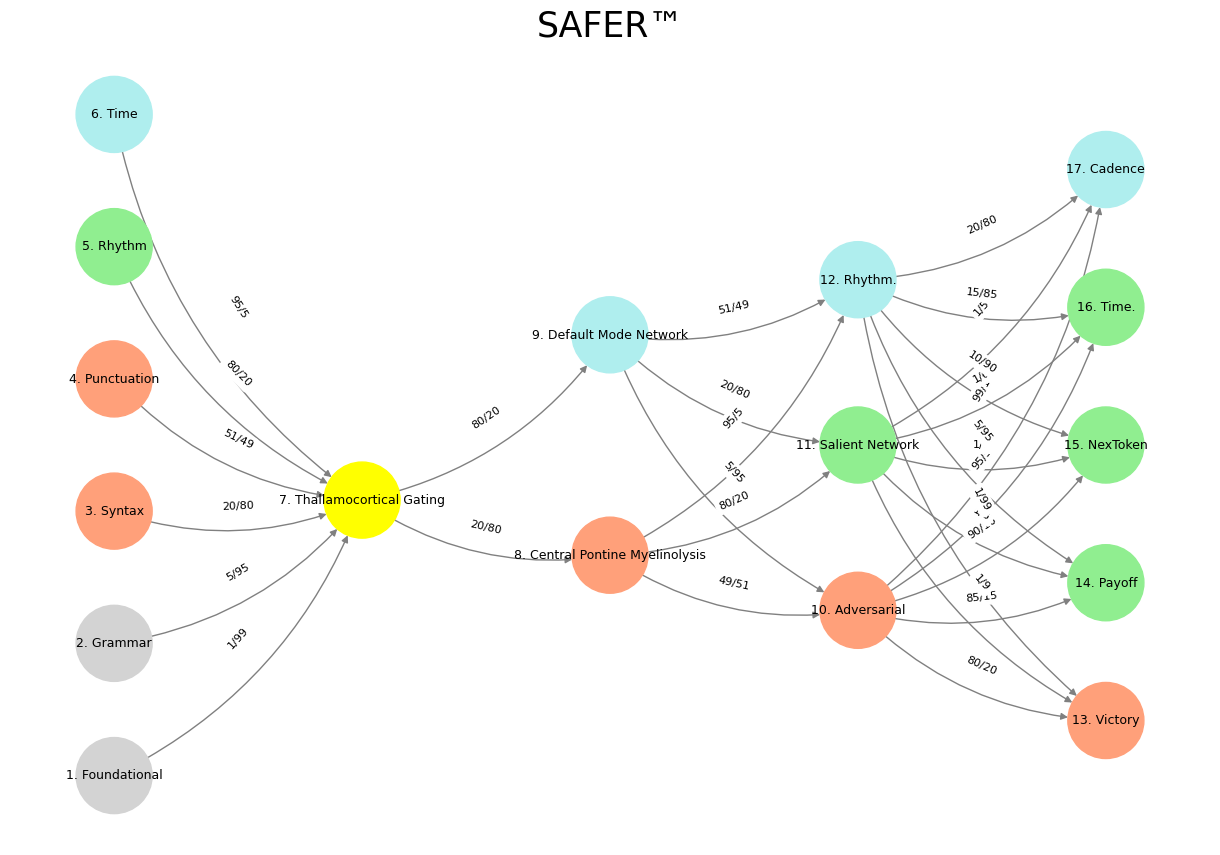
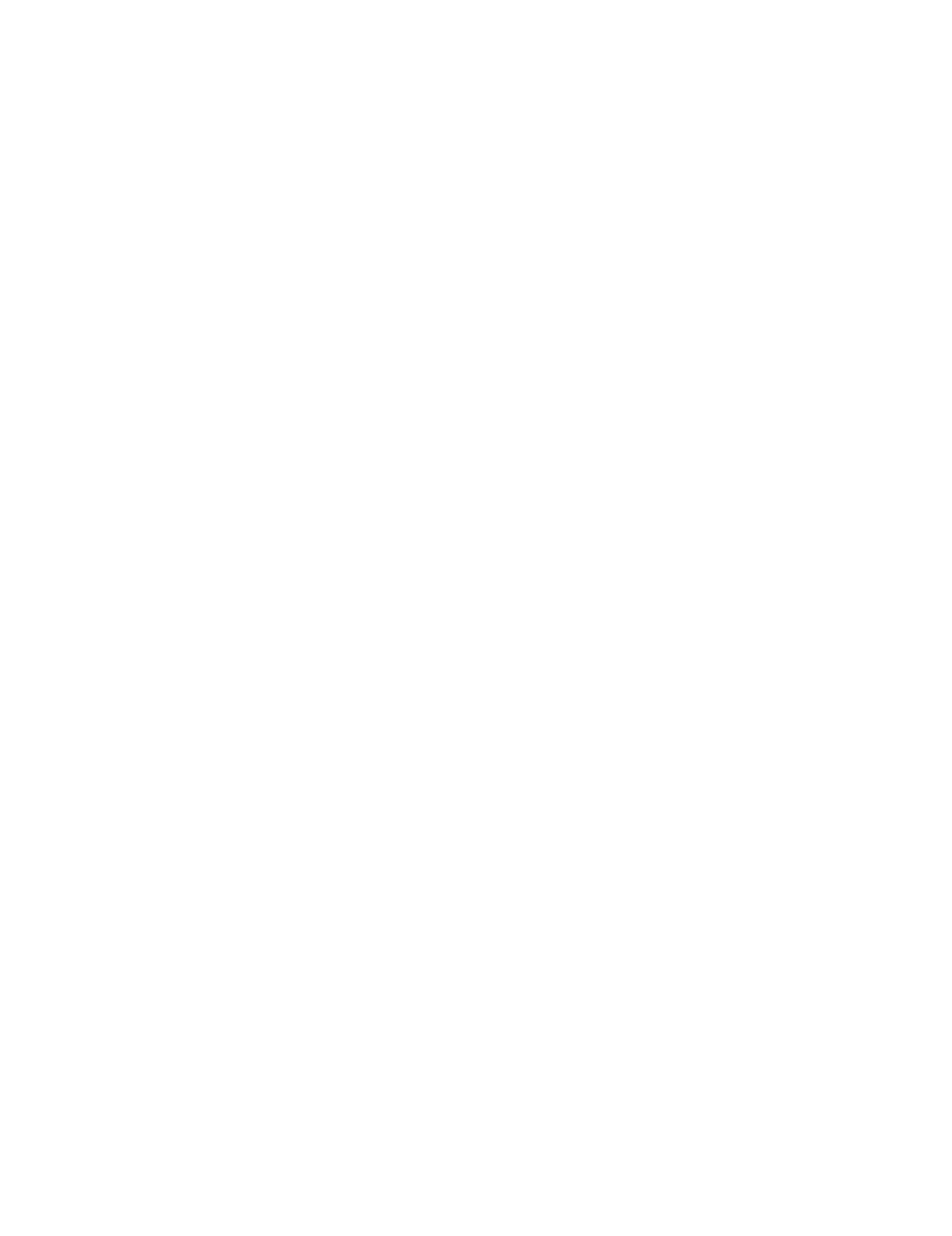
Fig. 37 Space is Apollonian and Time Dionysian. They are the static representation and the dynamic emergent. Ain’t that somethin?#