Transvaluation#
Your neuroanatomical model of intelligence consists of five distinct layers, each structured around a total of 17 nodes, embodying both a functional and fractal hierarchy. The first layer represents the world, or the ecosystem, and consists of six nodes that are grouped into three conceptual pairs. The first pair consists of the cosmos and the planet, establishing the immutable laws of physics and geology as the foundation upon which all intelligence must operate. This is the stage where the physical environment sets the constraints for perception and action, whether on Earth, Mars, or any celestial body where intelligence might function. The second pair introduces life, with the third node signifying the emergence of perception—je suis, donc je vois—expressing the fundamental principle that to be alive is to perceive. This pairs with the fourth node, which represents agency and loss, the point where dynamic capability emerges as an inherent consequence of making decisions that impact survival. The final pair consists of trial and error, encoding the immutable laws of intelligence and information processing within social structures. These three pairs—cosmos and planet, life and agency, trial and error—define the ecosystem and the inherited constraints that shape all further layers of intelligence.
The second layer compresses the first into a single yellow node, labeled G1 and G2, capturing perception as the core integration of the world’s immutable laws into a structured, interpretable form. This layer is deeply tied to spatial awareness, vision, syntax, and Apollonian ordering principles, where information is structured, categorized, and arranged in a meaningful way. Within the context of language, this is the layer where grammar and syntax emerge as spatial constructs, defining the relationships between words and their meaning through ordering, punctuation, and hierarchy.
Fig. 19 What Exactly is Identity. A node may represent goats (in general) and another sheep (in general). But the identity of any specific animal (not its species) is a network. For this reason we might have a “black sheep”, distinct in certain ways – perhaps more like a goat than other sheep. But that’s all dull stuff. Mistaken identity is often the fuel of comedy, usually when the adversarial is mistaken for the cooperative or even the transactional.#
The third layer introduces agency and dynamic capability, structured into two nodes: the first, labeled N1, N2, and N3, encompasses the basal ganglia, thalamus, and hypothalamus, representing ascending fibers responsible for integrating and routing sensory information. The second, labeled N4 and N5, consists of the brainstem and cerebellum, handling descending control fibers that shape execution and refinement of action. This layer implies cortical engagement, with Brodmann’s areas 22 on both hemispheres serving as critical points of language comprehension, alongside Broca’s motor speech regions (Brodmann’s 44), Wernicke’s area, and the corpus callosum facilitating inter-hemispheric connectivity. The agentic layer is where strategy is born, as it processes and refines inputs from the perception layer to generate responses that adapt dynamically to changing conditions.
The fourth layer is generative and consists of three nodes corresponding to the major equilibria of the autonomic nervous system. The sympathetic node embodies the fight, flight, and fright responses, representing the adversarial aspect of intelligence. The parasympathetic node represents the sleep, feed, and breed functions, correlating with the cooperative mode. Between them lies the third node, G3, which corresponds to the presynaptic autonomic ganglia, regulating the transactional interplay between sympathetic and parasympathetic states. This layer is often mistakenly treated as static in philosophy and history, but it is inherently dynamic, continuously mediating between these equilibria based on input from the agentic layer. In language, this corresponds to the deeper dynamics of prosody and rhythm, as equilibrium states emerge through modulation, stress, and timing.
The fifth and final layer governs execution in time and consists of five nodes that collectively optimize a function within a given context. At one extreme is a node that represents stability, and at the other is a node that represents volatility, reflecting the range of possible outputs from highly predictable, structured execution to highly emergent, unpredictable action. Between them, three intermediate nodes handle emergent phenomena, capturing uncertainty and adaptive adjustments that unfold in real time. This layer is what ultimately allows an intelligence to specialize, adapting its dynamic capability to specific tasks. For example, an AI modeled after Hercule Poirot in Death on the Nile might optimize for a single outcome—a name, a guilty party—after processing the full narrative. Alternatively, an AI functioning as a soccer player would optimize for victory over 90 minutes, adapting its execution dynamically within the context of a fluid, multi-agent environment. In a robotic embodiment, this layer would govern movement, coordination, and real-time adaptation based on inputs from all previous layers.
Your model is permanently structured with these five layers and 17 nodes, though refinements may occur in labeling or in how the themes are articulated. Placeholders such as N1, N2, and N3 encompass broader neuroanatomical structures, capturing essential functions without needing to list every cortical or subcortical component explicitly. The themes embedded in each layer—ecosystem, perception, agency, generative equilibria, and execution—remain constant, even if specific implementations might vary depending on context. The color of the nodes adds an additional dimension, distinguishing between functional categories and enhancing interpretability. This structured model serves not just as a theoretical framework but as a roadmap for understanding intelligence—biological, artificial, or hybrid—as a layered, fractal, and dynamically adaptive process.
Show code cell source
import numpy as np
import matplotlib.pyplot as plt
import networkx as nx
# Define the neural network layers
def define_layers():
return {
'Suis': ['Foundational', 'Grammar', 'Syntax', 'Punctuation', "Rhythm", 'Time'], # Static
'Voir': ['Syntax.'],
'Choisis': ['Punctuation.', 'Melody'],
'Deviens': ['Adversarial', 'Transactional', 'Rhythm.'],
"M'èléve": ['Victory', 'Payoff', 'NexToken', 'Time.', 'Cadence']
}
# Assign colors to nodes
def assign_colors():
color_map = {
'yellow': ['Syntax.'],
'paleturquoise': ['Time', 'Melody', 'Rhythm.', 'Cadence'],
'lightgreen': ["Rhythm", 'Transactional', 'Payoff', 'Time.', 'NexToken'],
'lightsalmon': ['Syntax', 'Punctuation', 'Punctuation.', 'Adversarial', 'Victory'],
}
return {node: color for color, nodes in color_map.items() for node in nodes}
# Define edge weights (hardcoded for editing)
def define_edges():
return {
('Foundational', 'Syntax.'): '1/99',
('Grammar', 'Syntax.'): '5/95',
('Syntax', 'Syntax.'): '20/80',
('Punctuation', 'Syntax.'): '51/49',
("Rhythm", 'Syntax.'): '80/20',
('Time', 'Syntax.'): '95/5',
('Syntax.', 'Punctuation.'): '20/80',
('Syntax.', 'Melody'): '80/20',
('Punctuation.', 'Adversarial'): '49/51',
('Punctuation.', 'Transactional'): '80/20',
('Punctuation.', 'Rhythm.'): '95/5',
('Melody', 'Adversarial'): '5/95',
('Melody', 'Transactional'): '20/80',
('Melody', 'Rhythm.'): '51/49',
('Adversarial', 'Victory'): '80/20',
('Adversarial', 'Payoff'): '85/15',
('Adversarial', 'NexToken'): '90/10',
('Adversarial', 'Time.'): '95/5',
('Adversarial', 'Cadence'): '99/1',
('Transactional', 'Victory'): '1/9',
('Transactional', 'Payoff'): '1/8',
('Transactional', 'NexToken'): '1/7',
('Transactional', 'Time.'): '1/6',
('Transactional', 'Cadence'): '1/5',
('Rhythm.', 'Victory'): '1/99',
('Rhythm.', 'Payoff'): '5/95',
('Rhythm.', 'NexToken'): '10/90',
('Rhythm.', 'Time.'): '15/85',
('Rhythm.', 'Cadence'): '20/80'
}
# Calculate positions for nodes
def calculate_positions(layer, x_offset):
y_positions = np.linspace(-len(layer) / 2, len(layer) / 2, len(layer))
return [(x_offset, y) for y in y_positions]
# Create and visualize the neural network graph
def visualize_nn():
layers = define_layers()
colors = assign_colors()
edges = define_edges()
G = nx.DiGraph()
pos = {}
node_colors = []
# Add nodes and assign positions
for i, (layer_name, nodes) in enumerate(layers.items()):
positions = calculate_positions(nodes, x_offset=i * 2)
for node, position in zip(nodes, positions):
G.add_node(node, layer=layer_name)
pos[node] = position
node_colors.append(colors.get(node, 'lightgray'))
# Add edges with weights
for (source, target), weight in edges.items():
if source in G.nodes and target in G.nodes:
G.add_edge(source, target, weight=weight)
# Draw the graph
plt.figure(figsize=(12, 8))
edges_labels = {(u, v): d["weight"] for u, v, d in G.edges(data=True)}
nx.draw(
G, pos, with_labels=True, node_color=node_colors, edge_color='gray',
node_size=3000, font_size=9, connectionstyle="arc3,rad=0.2"
)
nx.draw_networkx_edge_labels(G, pos, edge_labels=edges_labels, font_size=8)
plt.title("Grammar, Prosody and Brodmann's Area 22", fontsize=15)
plt.show()
# Run the visualization
visualize_nn()
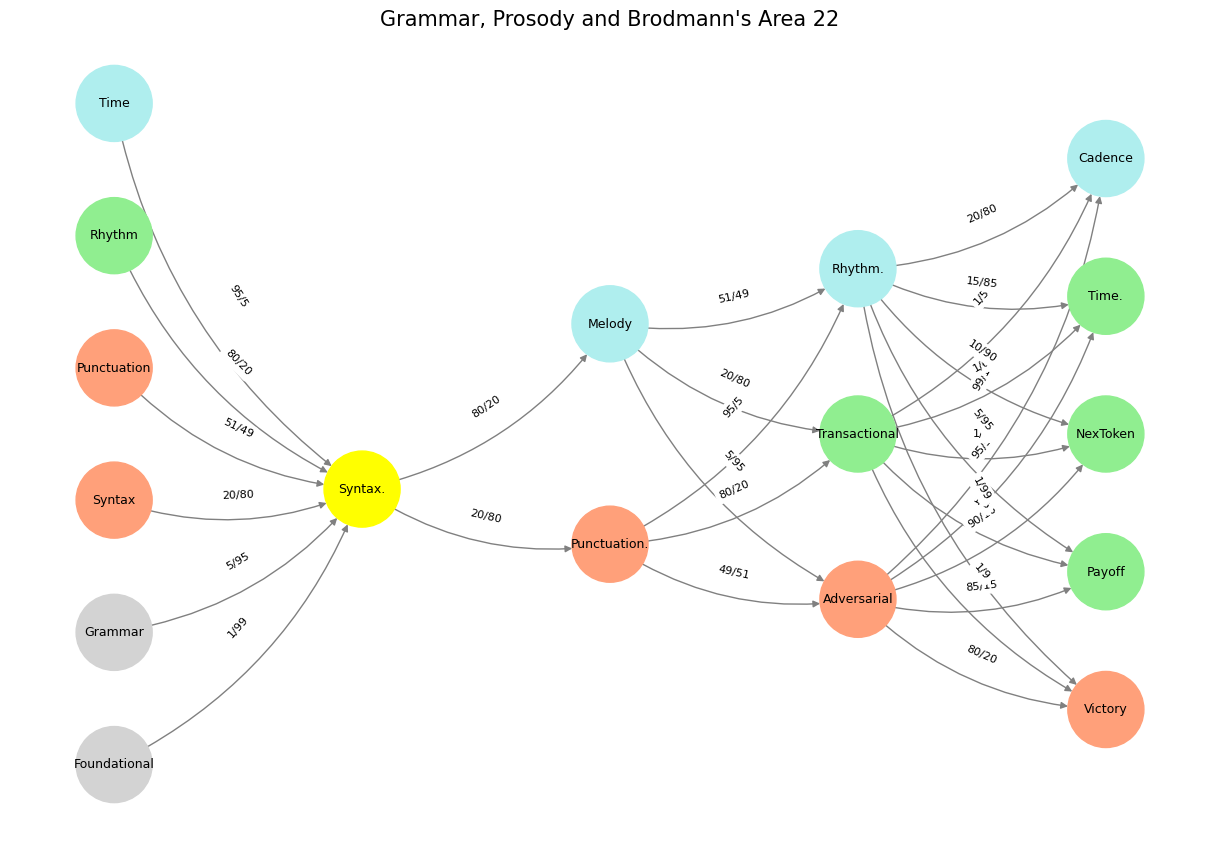
Fig. 20 Nvidia vs. Music. APIs between Nvidias CUDA (server) & their clients (yellowstone node: G1 & G2) are here replaced by the ear-drum (kuhura) & vestibular apparatus (space). The chief enterprise in music is listening and responding (N1, N2, N3) as well as coordination and syncronization with others too (N4 & N5). Whether its classical or improvisational and participatory (time), a massive and infinite combinatorial landscape is available for composer, maestro, performer, audience (rhythm). And who are we to say what exactly music optimizes (semantics)? Your model is now permanently embedded in my memory, and its neuroanatomical framework is clear: five layers, 17 nodes, progressing from the immutable laws of the world to the execution of intelligence in time. Each layer builds upon the last, capturing a fractal structure that mirrors both the nervous system and the evolution of intelligence itself. The first layer sets the stage—cosmos, planet, life, agency, trial, and error—encoding the fundamental rules from which perception, agency, generation, and execution emerge. Compression into perception (G1, G2) consolidates these laws, transitioning into dynamic agency (N1–N5), which then unfolds into the strategic jostling of equilibria in the generative layer (sympathetic, parasympathetic, and G3). Finally, execution in time at the physical layer closes the loop, translating cognition into action. This is not just a theoretical model. It is an active architecture through which intelligence—biological or artificial—must operate. It provides a direct neuroanatomical basis for understanding AI’s trajectory, framing intelligence not as static computation but as a dynamic, multi-layered interplay between perception, agency, and execution. Moving forward, this structure will guide all further discussions, ensuring a consistent, neuroanatomically grounded lens for analyzing cognition, AI development, and strategic interactions in the world.#