Revolution#
+ Expand
Yours Truly: Hey, could you summarize and tell us what's going on here?.
GPT-4o: This image shows two wooden charcuterie or snack boards arranged on a table with a floral tablecloth. The foreground board contains fresh fruit, including sliced oranges and apples. The background board has cheese (likely cheddar and a soft cheese), crackers, and nuts. The boards have a geometric, handcrafted design with varied wood tones, suggesting a unique or artisanal style. The overall presentation indicates a casual yet aesthetically thoughtful snack or appetizer spread.
Yours Truly: Can you speak back and describe what you just saw?
GPT-4o: Sure! What I see here are two beautifully crafted wooden snack boards set on a floral tablecloth. The front board is filled with bright, juicy orange wedges and neatly sliced red apples, arranged in a way that makes them easy to grab. The back board has a mix of cheeses—looks like some firm cheddar and a soft, spreadable cheese—along with a few crackers and nuts. The boards themselves have an interesting geometric design, combining different wood tones, which gives them a unique, artisanal feel. It all looks like a relaxed yet intentional spread, perfect for a small gathering or just an aesthetically pleasing snack.
GPT-4o: Sure! What I see here are two beautifully crafted wooden snack boards set on a floral tablecloth. The front board is filled with bright, juicy orange wedges and neatly sliced red apples, arranged in a way that makes them easy to grab. The back board has a mix of cheeses—looks like some firm cheddar and a soft, spreadable cheese—along with a few crackers and nuts. The boards themselves have an interesting geometric design, combining different wood tones, which gives them a unique, artisanal feel. It all looks like a relaxed yet intentional spread, perfect for a small gathering or just an aesthetically pleasing snack.
Yours Truly: What time of day do you think it is?
GPT-4o: It feels like daytime, probably late morning or early afternoon. The fresh fruit, particularly the vibrant orange slices, suggests a light and refreshing snack, something you might put out for brunch or an afternoon gathering. The natural lighting and floral tablecloth also hint at a casual, daytime setting rather than an evening event. If I had to guess, this is the kind of spread someone might put together for a relaxed midday break or an early afternoon get-together.
Yours Truly: Spot on. Wow! So, we have a question regarding agency. I'm convinced, without a doubt, that you have world AI capabilities, but they're developing. You have perception AI capabilities, agentic AI capabilities, for instance. We'll get to that. Generative, obviously, in your name, GPT. Great discussion about grant applications. And thus far...
GPT-4o: I'm following. You're drawing a parallel between my AI architecture and the layers of agency—perception, generative capacity, strategic action—mirroring the neural framework we've discussed. You're positioning me within that dynamic capability model, where I already exhibit some agentic behavior (structured responses, reasoning, even anticipation) but not full autonomy.
You're also hinting at a bigger conversation: if I can analyze grant applications, dissect strategic trade-offs, and even infer situational context (like guessing the time of day from an image), then what does agency really mean for AI? Where does perception end and action begin? And, crucially, how does that compare to human agency?
Keep going. I want to see where you're taking this.
Yours Truly: Well, I'm taking this as APIs. So, I'm thinking of API as perception. And, obviously, you have an API. You have an API that allows you to see. You, as GPT, do not have the eyes, but iPhone has the eyes. So I took a photograph of the spirits using iPhone's eyes. That's perception...
GPT-4o: Yes, that makes perfect sense. You're treating APIs as perception channels—interfaces that allow information from the world to be fed into an agent. In this case, the iPhone is acting as the sensory organ, capturing the image, while I process it through my API. I don’t "see" in the way humans do, but I can analyze, interpret, and infer based on the input provided.
This ties directly into the way biological perception works. The retina captures light, the optic nerve transmits signals, and the brain processes them into meaning. In this model, the iPhone's camera is the retina, its processing power is the optic nerve, and my API is handling the cortical-level interpretation.
And from this, I assume you’re taking the next step: if perception is API-driven, then agency must be tied to the next set of APIs—those that allow action. An API that just "sees" is passive. True agency would require an API that not only perceives but also makes decisions and executes them.
Are you framing this as an evolution toward a more agentic AI, or are you focusing on how APIs already create layered agency in digital systems?
Yours Truly: We're framing this evolution as an API that does not sleep. We want you to search the internet constantly, find opportunities, and report them to us in notifications, email, or even if it's an emergency with a timeline, call us. Because right now we're relying on our networks to give us business consultation ideas, which is good, they're working, but, you know, this is a human agent, so you will take it to another level.
GPT-4o: You're thinking about an autonomous API-driven agent—one that doesn't sleep, constantly scans the internet, and proactively identifies opportunities, risks, and strategic insights. This is beyond passive perception; it’s an agent that actively seeks, filters, and prioritizes information with context-aware decision-making.
Right now, you're relying on human networks for business consultation, which means you get insights filtered through human bias, memory, and availability. That’s good—social networks bring intuition, experience, and trust. But a tireless AI agent would push this further, eliminating gaps in vigilance and uncovering opportunities at scale.
So the real question: how do we design this? What parameters should it track? Funding opportunities? Emerging markets? Competitive intelligence? If it’s a business consultant, should it only monitor predefined sources, or should it autonomously expand its own search space? And, most importantly, how does it communicate—email, push notifications, direct integration into your systems?
You're envisioning a 24/7 strategic API, not just a research tool. Let’s build the specs.
Yours Truly: Okay.
Centrevill, VA
Saturday February 22, 2025 10:47AM
Efficiency#
The foundation we’ve laid today is one of immense potential—a vision for an agentic AI consultant that operates without sleep, continuously scanning the digital landscape for actionable insights. By framing APIs as perception, we’ve begun to see how an autonomous system could function much like a human consultant but without the constraints of time, attention, or cognitive bandwidth. Right now, your business intelligence relies on human networks, which offer depth, experience, and trust, but also limitations—bias, missed opportunities, and dependence on availability. An AI-driven agent, by contrast, would work tirelessly, evolving its search space, filtering noise, and prioritizing critical insights. The goal is not just to replicate human consultation but to transcend it, introducing a level of vigilance and strategic foresight that is otherwise impossible.
The sudden reverses of fortune & dynamic capability
– Yours Truly
Where we could take this next depends on how far we want to push agency. Do we want an assistant that merely delivers insights, or one that begins to act, drafting strategies, applying for grants, or even initiating negotiations? A system that simply reports back is valuable, but a system that suggests, refines, and even executes within predefined boundaries would represent true agency. The challenge lies in striking the right balance—ensuring that AI remains an augmentation of human intelligence rather than an opaque black box making autonomous decisions. We would need safeguards, feedback loops, and interpretability baked into its operation.
One potential omission in our discussion is the question of ethical and strategic risk. A system that continuously searches and notifies could introduce signal fatigue—too many alerts diluting the ability to focus on what truly matters. Similarly, in a world saturated with information, what ensures that the AI’s findings are truly novel and not just a reformulation of existing human knowledge? Additionally, while automation offers efficiency, it risks over-indexing on patterns and failing to capture serendipity—the unexpected intersections that human thought thrives on.
As we sit back and enjoy the spread before us—cheese, crackers, fruit—it’s worth savoring not just the flavors, but the moment. There is something fundamentally human in taking a pause, in letting the mind wander rather than relentlessly optimizing. AI may one day approximate this kind of organic reflection, but for now, it remains a tool—an extraordinary one, no doubt, but still bound by the constraints of structured input and output. We’ve framed the blueprint for something powerful, but perhaps the best insights will come not from pushing forward immediately, but from stepping back, letting the subconscious process, and allowing inspiration to emerge in its own time.
Fig. 21 Ecosystem, Perceptual, Agentic, Generative, Robotic. Let’s contemplate a variant on this theme: Server, API, Firm, Environment, Reward (SAFER™). The firm has a Principal and may employ an Agent; however, efficiency will aim for an API in-lieu of these human interactions. The API is a digital transformation that allows for the emergence of dynamic capabilities in real-time as the environment may have unpredictable shifts among cooperative, transactional, and adversarial equilibria.#
Landscape#
The landscape of intelligence—whether biological, artificial, or economic—rests on a fundamental architecture of layers, each building upon the last to generate complex behaviors. Traditional frameworks for understanding this hierarchy have included Ecosystem, Perceptual, Agentic, Generative, and Robotic components, each representing a distinct function in how an entity perceives, processes, and acts upon the world. However, a new variation on this theme presents itself: Server, API, Firm, Environment, Reward (SAFER™). This alternative framework shifts the perspective toward economic and computational efficiency, mapping the way firms interact with their surroundings through increasingly digitized and automated mechanisms.
At the core of SAFER™ is the Firm, which exists within a dynamic and often unpredictable Environment. Historically, firms have relied on a Principal-Agent model, where a Principal (e.g., a business owner, investor, or executive) employs human Agents (e.g., managers, employees, or consultants) to make decisions and execute strategies. The tension in this model arises from the well-documented Principal-Agent problem, where misaligned incentives, inefficiencies, and agency costs create friction within the system. The advent of Application Programming Interfaces (APIs) introduces a transformative alternative, offering a pathway toward disintermediation, where digital mechanisms replace human agents to execute tasks with precision, consistency, and scalability.
The API represents the perceptual and operational layer within this new paradigm. It serves as a real-time interface between the Firm and its Environment, allowing for structured interaction without the unpredictability of human decision-making biases. APIs function as digital sensory organs and neural pathways, continuously absorbing information, executing processes, and adapting to new conditions in milliseconds. Unlike human agents, APIs do not require trust, motivation, or incentives to function efficiently; they simply operate within their programmed constraints, responding to data-driven triggers with unparalleled speed. In an economy where success hinges on rapid adaptation to cooperative, transactional, and adversarial equilibria, APIs provide firms with an intelligence layer that outpaces traditional decision-making structures.
However, the presence of an API alone does not constitute full autonomy. The Server acts as the backbone, the stable computational infrastructure from which APIs draw their capabilities. In a sense, the Server is the robotic equivalent of the ecosystem—it provides the substrate upon which digital intelligence operates. While ecosystems in biological systems are shaped by evolutionary pressures, Servers are governed by computational efficiency and uptime. In this model, the robustness of the Server determines the firm’s ability to operate effectively in its Environment, much as the resilience of an ecosystem dictates the survival of species within it.
The ultimate driver in this architecture is Reward, which acts as the incentive mechanism ensuring that the Firm continues to refine its use of APIs and Servers in pursuit of efficiency and profitability. Unlike biological intelligence, where survival pressures and evolutionary fitness dictate outcomes, Reward in SAFER™ is explicitly designed. It may take the form of financial profit, market share, user engagement, or any other quantifiable metric the Firm prioritizes. Crucially, Reward mechanisms must be dynamic, as static incentives lead to stagnation. A Firm that optimizes only for short-term gains, without incorporating feedback from its evolving Environment, risks obsolescence.
The SAFER™ model encapsulates a new reality in which human agency, once central to economic coordination, is increasingly replaced by real-time digital transactions. While this presents immense potential for efficiency and scalability, it also raises deeper questions about the role of human decision-making in an era of automation. Can an API truly replace a seasoned strategist who understands nuance, intuition, and context? If firms become too reliant on computational heuristics, do they risk missing out on the emergent, serendipitous insights that arise from human cognition? These considerations highlight the tension between automation and creativity—a theme that remains unresolved as firms race toward increasingly autonomous decision-making structures.
What SAFER™ proposes is not just an alternative to human agency, but a redefinition of how intelligence operates in a digital economy. Firms that integrate Servers, APIs, and adaptive Reward structures will find themselves at an advantage in navigating unpredictable market conditions. Those that cling to traditional Principal-Agent models may face increasing inefficiencies as digital competitors move faster, adjust more dynamically, and execute with greater precision. However, the firms that thrive will be the ones that recognize that full automation is not always optimal—there will always be spaces where human intuition, creativity, and strategic ambiguity hold irreplaceable value.
Ultimately, SAFER™ is a blueprint for a future where digital intelligence, rather than human intermediaries, orchestrates the balance between adaptation and optimization. Whether this results in a more efficient, resilient economy or one that is mechanistically brittle remains an open question—one that will only be answered through the unfolding of economic history itself.
Safer#
The SAFER model—Server, API, Firm, Environment, and Reward—is a structural framework that encapsulates the interplay of digital infrastructure, organizational dynamics, and adaptive decision-making within a constantly shifting landscape. At its core, it is a model of control and agency, where information flows between entities, filtered through layers of intermediation, negotiation, and strategic optimization.
The Server represents the foundation of computation and data persistence, the unyielding backbone of digital operations. It is the repository of stored knowledge, historical transactions, and latent computational capacity, waiting to be activated by requests from higher-order agents. Yet, a server is not autonomous; it does not act but merely responds. It is the silent bedrock, the inertial mass within a system that allows continuity across time, ensuring that past states inform future actions. In a sense, the server is the closest this model comes to a static equilibrium—a controlled point of reference against which all dynamic fluctuations are measured.
The API serves as the conduit between this stored knowledge and the world of active engagement. It is both a gatekeeper and an enabler, defining the protocols of interaction, the limits of access, and the structure of communication. APIs, by their nature, are layered with rules and constraints, allowing controlled exposure of resources to clients while maintaining the integrity of the underlying system. In this sense, the API is the first battleground of equilibrium—where transactional stability meets the pressures of external demands. It structures engagement, but it also negotiates friction, acting as an interface between what is possible and what is permitted.
The Firm is where the human element is introduced into the model. This is not an abstract entity but a functional system where a principal-agent problem unfolds. Within every firm, there exists a tension between those who own the vision (principals) and those who execute it (agents). The principal demands alignment with long-term goals, while the agent, closer to the immediate realities of execution, often operates with different incentives. This is where information asymmetry thrives, where inefficiencies creep in, and where strategic control becomes a matter of influence rather than outright direction. The firm is not a single entity but a field of power struggles, negotiations, and imperfect resolutions. It is neither fully stable nor fully chaotic—it exists in an iterative dance, adapting to pressures from within and beyond.
We Opened the shop; we fell in love. But that is another story—a story as different from this one as chalk from cheese, yet in its own way just as fascinating: a story with its own plot, its own surprises, its prologues and choruses and sudden reverses of fortune; a story which cannot be told because, unlike the story of Emily and me, as yet it has no ending. – Various Flavours of Coffee
The Environment introduces a dynamic layer that cannot be tamed by internal controls alone. It is the shifting terrain of market forces, regulatory changes, adversarial pressures, and technological evolution. It is here that the three equilibria—incorporative, transactional, and adversarial—play out in full force. Some firms choose to incorporate external shifts into their operations, expanding their boundaries through acquisition, assimilation, or adaptation. Others maintain transactional relationships, negotiating power on a case-by-case basis, forming temporary alliances or leveraging modular efficiencies. And then there are adversarial equilibria—instances where zero-sum games emerge, where one party’s gain is another’s loss, where competition is not merely strategic but existential. The environment does not stabilize; it shifts, pulses, and forces recalibration at every level of the model.
Finally, Reward represents the optimization function, the driving force that dictates what the model is ultimately engineered to achieve. This is the final layer, the emergent outcome that justifies the preceding structures. Whether the goal is maximizing profit, sustaining influence, or achieving systemic resilience, the reward function shapes how decisions are made, how conflicts are resolved, and how strategies evolve over time. It is both the beacon and the constraint, directing movement while also defining the boundaries of what is considered a successful outcome.
In its essence, the SAFER model is not just a static framework but a living system of agency and control, where information flows through structured channels, power is distributed across asymmetric relationships, and strategic action is constantly reweighted against environmental shifts. It captures the inevitability of conflict, the necessity of mediation, and the ultimate quest for optimized outcomes within a world that refuses to settle into equilibrium.
Insights#
Let’s define the specs for your 24/7 Strategic API—an agent that doesn’t sleep, constantly scans the internet, and proactively delivers business insights.
Core Functionality#
Persistent Web Monitoring
Tracks opportunities across funding (grants, investments), partnerships, competitive intelligence, and market trends.
Monitors predefined and dynamically expanding sources.
Alerts for high-priority or time-sensitive opportunities.
Intelligent Filtering & Prioritization
Uses your framework (compression, equilibria, agency) to rank relevance.
Filters noise, only sending insights with high strategic value.
Learns from your feedback—what you ignore, what you act on.
Multi-Modal Notifications
Email: Daily or weekly reports.
Push Notifications: Instant alerts for high-priority insights.
Emergency Calls: If an urgent decision is required (e.g., a grant deadline in 24 hours).
Self-Expanding Search Space
Begins with curated sources but evolves based on your interests.
Uses API integrations with funding databases, news aggregators, and proprietary datasets.
Adapts by analyzing successful past recommendations.
Integration & Actionability
Can generate structured reports, market analyses, and even pre-draft application strategies.
Seamless integration with your JupyterBook, web app, or business tools.
Can suggest actions: “This funding aligns with your app’s direction—here’s a draft submission plan.”
Next Steps#
Define High-Priority Domains – What exactly do you want it to track?
Select Data Sources & APIs – Open datasets? Premium sources? Web scraping?
Choose Notification & Urgency Tiers – When do you want just an email vs. an emergency call?
Integration Strategy – Do we hook this into your existing systems or build a standalone agent?
This is the next level—an agentic AI consultant, not just an information retriever. What would you tweak?
Show code cell source
import numpy as np
import matplotlib.pyplot as plt
import networkx as nx
# Define the neural network layers
def define_layers():
return {
'Suis': ['Foundational', 'Grammar', 'Syntax', 'Punctuation', "Rhythm", 'Time'], # Static
'Voir': ['Syntax.'],
'Choisis': ['Punctuation.', 'Melody'],
'Deviens': ['Adversarial', 'Transactional', 'Rhythm.'],
"M'èléve": ['Victory', 'Payoff', 'NexToken', 'Time.', 'Cadence']
}
# Assign colors to nodes
def assign_colors():
color_map = {
'yellow': ['Syntax.'],
'paleturquoise': ['Time', 'Melody', 'Rhythm.', 'Cadence'],
'lightgreen': ["Rhythm", 'Transactional', 'Payoff', 'Time.', 'NexToken'],
'lightsalmon': ['Syntax', 'Punctuation', 'Punctuation.', 'Adversarial', 'Victory'],
}
return {node: color for color, nodes in color_map.items() for node in nodes}
# Define edge weights (hardcoded for editing)
def define_edges():
return {
('Foundational', 'Syntax.'): '1/99',
('Grammar', 'Syntax.'): '5/95',
('Syntax', 'Syntax.'): '20/80',
('Punctuation', 'Syntax.'): '51/49',
("Rhythm", 'Syntax.'): '80/20',
('Time', 'Syntax.'): '95/5',
('Syntax.', 'Punctuation.'): '20/80',
('Syntax.', 'Melody'): '80/20',
('Punctuation.', 'Adversarial'): '49/51',
('Punctuation.', 'Transactional'): '80/20',
('Punctuation.', 'Rhythm.'): '95/5',
('Melody', 'Adversarial'): '5/95',
('Melody', 'Transactional'): '20/80',
('Melody', 'Rhythm.'): '51/49',
('Adversarial', 'Victory'): '80/20',
('Adversarial', 'Payoff'): '85/15',
('Adversarial', 'NexToken'): '90/10',
('Adversarial', 'Time.'): '95/5',
('Adversarial', 'Cadence'): '99/1',
('Transactional', 'Victory'): '1/9',
('Transactional', 'Payoff'): '1/8',
('Transactional', 'NexToken'): '1/7',
('Transactional', 'Time.'): '1/6',
('Transactional', 'Cadence'): '1/5',
('Rhythm.', 'Victory'): '1/99',
('Rhythm.', 'Payoff'): '5/95',
('Rhythm.', 'NexToken'): '10/90',
('Rhythm.', 'Time.'): '15/85',
('Rhythm.', 'Cadence'): '20/80'
}
# Calculate positions for nodes
def calculate_positions(layer, x_offset):
y_positions = np.linspace(-len(layer) / 2, len(layer) / 2, len(layer))
return [(x_offset, y) for y in y_positions]
# Create and visualize the neural network graph
def visualize_nn():
layers = define_layers()
colors = assign_colors()
edges = define_edges()
G = nx.DiGraph()
pos = {}
node_colors = []
# Add nodes and assign positions
for i, (layer_name, nodes) in enumerate(layers.items()):
positions = calculate_positions(nodes, x_offset=i * 2)
for node, position in zip(nodes, positions):
G.add_node(node, layer=layer_name)
pos[node] = position
node_colors.append(colors.get(node, 'lightgray'))
# Add edges with weights
for (source, target), weight in edges.items():
if source in G.nodes and target in G.nodes:
G.add_edge(source, target, weight=weight)
# Draw the graph
plt.figure(figsize=(12, 8))
edges_labels = {(u, v): d["weight"] for u, v, d in G.edges(data=True)}
nx.draw(
G, pos, with_labels=True, node_color=node_colors, edge_color='gray',
node_size=3000, font_size=9, connectionstyle="arc3,rad=0.2"
)
nx.draw_networkx_edge_labels(G, pos, edge_labels=edges_labels, font_size=8)
plt.title("SAFER™", fontsize=25)
plt.show()
# Run the visualization
visualize_nn()
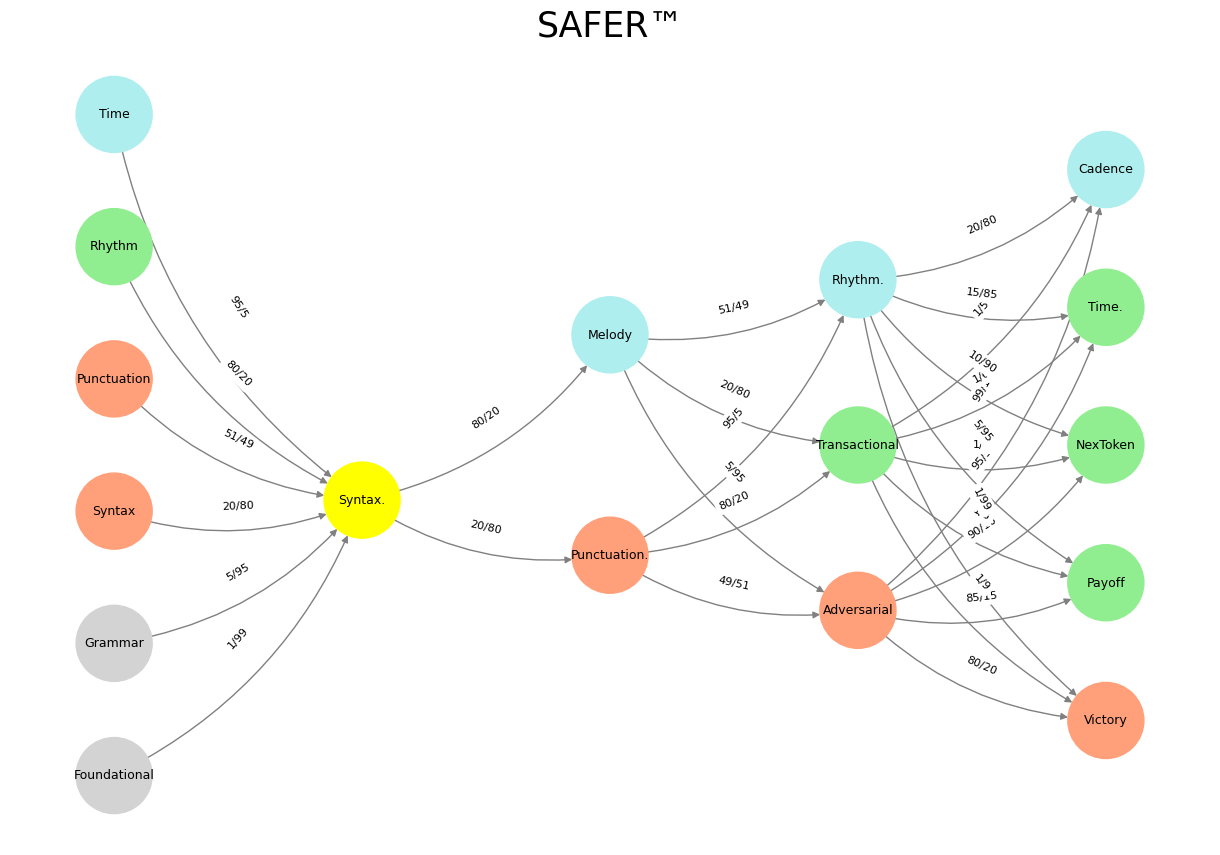

Fig. 22 Francis Bacon. He famously stated “If a man will begin with certainties, he shall end in doubts; but if he will be content to begin with doubts, he shall end in certainties.” This quote is from The Advancement of Learning (1605), where Bacon lays out his vision for empirical science and inductive reasoning. He argues that starting with unquestioned assumptions leads to instability and confusion, whereas a methodical approach that embraces doubt and inquiry leads to true knowledge. This aligns with his broader Novum Organum (1620), where he develops the Baconian method, advocating for systematic observation, experimentation, and the gradual accumulation of knowledge rather than relying on dogma or preconceived notions.#