Revolution#
The link between the vestibular apparatus and hearing seems tenuous if considered purely in anatomical or physiological terms, but once we expand the frame to include group-level effects—particularly in the realms of dance, rhythm, and ritual—the connection deepens into something fundamental. The vestibular system, responsible for balance and spatial orientation, and the auditory system, responsible for perceiving sound, are not merely adjacent in the inner ear; they form an integrated sensory platform that underpins movement, coordination, and synchronization—elements that are vital for collective human experience.
Fig. 26 What Exactly is Identity. A node may represent goats (in general) and another sheep (in general). But the identity of any specific animal (not its species) is a network. For this reason we might have a “black sheep”, distinct in certain ways – perhaps more like a goat than other sheep. But that’s all dull stuff. Mistaken identity is often the fuel of comedy, usually when the adversarial is mistaken for the cooperative or even the transactional.#
From Individual Physiology to Collective Synchronization#
At the individual level, the vestibular system stabilizes vision and enables us to process auditory stimuli without the distortions caused by motion. This is crucial for speech perception, as head movements can otherwise disrupt the ability to track spoken words. But at the group level, the vestibular-auditory link becomes even more consequential. Dance, synchronized movement, and ritualistic actions depend on an intricate feedback loop between balance and rhythm. Without the vestibular system providing spatial stability, the capacity to entrain to a beat—one of the defining characteristics of human social cohesion—would be compromised.
Vestibular-Auditory Integration as a Group-Level Effect#
If we accept that hearing and balance are integrated not just for individual function but for group-level effects, the tenuousness of their link dissolves. They are part of the same underlying system that makes rhythm, dance, and ritual possible. The vestibular system ensures stability in motion, the auditory system provides structure in time, and together they create the conditions for synchrony—a force that binds individuals into a cohesive whole. Seen through this lens, their connection is not an accident of anatomy but a profound evolutionary innovation that enables collective intelligence, cooperation, and culture.
Show code cell source
import numpy as np
import matplotlib.pyplot as plt
import networkx as nx
# Define the neural network fractal
def define_layers():
return {
'World': ['Particles-Compression', 'Vibration-Particulate.Matter', 'Ear, Cerebellum-Georientation', 'Harmonic Series-Agency.Phonology', 'Space-Verb.Syntax', 'Time-Object.Meaning', ], # Resources, Strength
'Perception': ['Rhythm, Pockets'], # Needs, Will
'Agency': ['Open-Nomiddleman', 'Closed-Trusted'], # Costs, Cause
'Generative': ['Ratio-Weaponized', 'Competition-Tokenized', 'Odds-Monopolized'], # Means, Ditto
'Physical': ['Volatile-Revolutionary', 'Unveiled-Resentment', 'Freedom-Dance in Chains', 'Exuberant-Jubilee', 'Stable-Conservative'] # Ends, To Do
}
# Assign colors to nodes
def assign_colors():
color_map = {
'yellow': ['Rhythm, Pockets'],
'paleturquoise': ['Time-Object.Meaning', 'Closed-Trusted', 'Odds-Monopolized', 'Stable-Conservative'],
'lightgreen': ['Space-Verb.Syntax', 'Competition-Tokenized', 'Exuberant-Jubilee', 'Freedom-Dance in Chains', 'Unveiled-Resentment'],
'lightsalmon': [
'Ear, Cerebellum-Georientation', 'Harmonic Series-Agency.Phonology', 'Open-Nomiddleman',
'Ratio-Weaponized', 'Volatile-Revolutionary'
],
}
return {node: color for color, nodes in color_map.items() for node in nodes}
# Calculate positions for nodes
def calculate_positions(layer, x_offset):
y_positions = np.linspace(-len(layer) / 2, len(layer) / 2, len(layer))
return [(x_offset, y) for y in y_positions]
# Create and visualize the neural network graph
def visualize_nn():
layers = define_layers()
colors = assign_colors()
G = nx.DiGraph()
pos = {}
node_colors = []
# Add nodes and assign positions
for i, (layer_name, nodes) in enumerate(layers.items()):
positions = calculate_positions(nodes, x_offset=i * 2)
for node, position in zip(nodes, positions):
G.add_node(node, layer=layer_name)
pos[node] = position
node_colors.append(colors.get(node, 'lightgray')) # Default color fallback
# Add edges (automated for consecutive layers)
layer_names = list(layers.keys())
for i in range(len(layer_names) - 1):
source_layer, target_layer = layer_names[i], layer_names[i + 1]
for source in layers[source_layer]:
for target in layers[target_layer]:
G.add_edge(source, target)
# Draw the graph
plt.figure(figsize=(12, 8))
nx.draw(
G, pos, with_labels=True, node_color=node_colors, edge_color='gray',
node_size=3000, font_size=8, connectionstyle="arc3,rad=0.2"
)
plt.title("Music", fontsize=13)
plt.show()
# Run the visualization
visualize_nn()
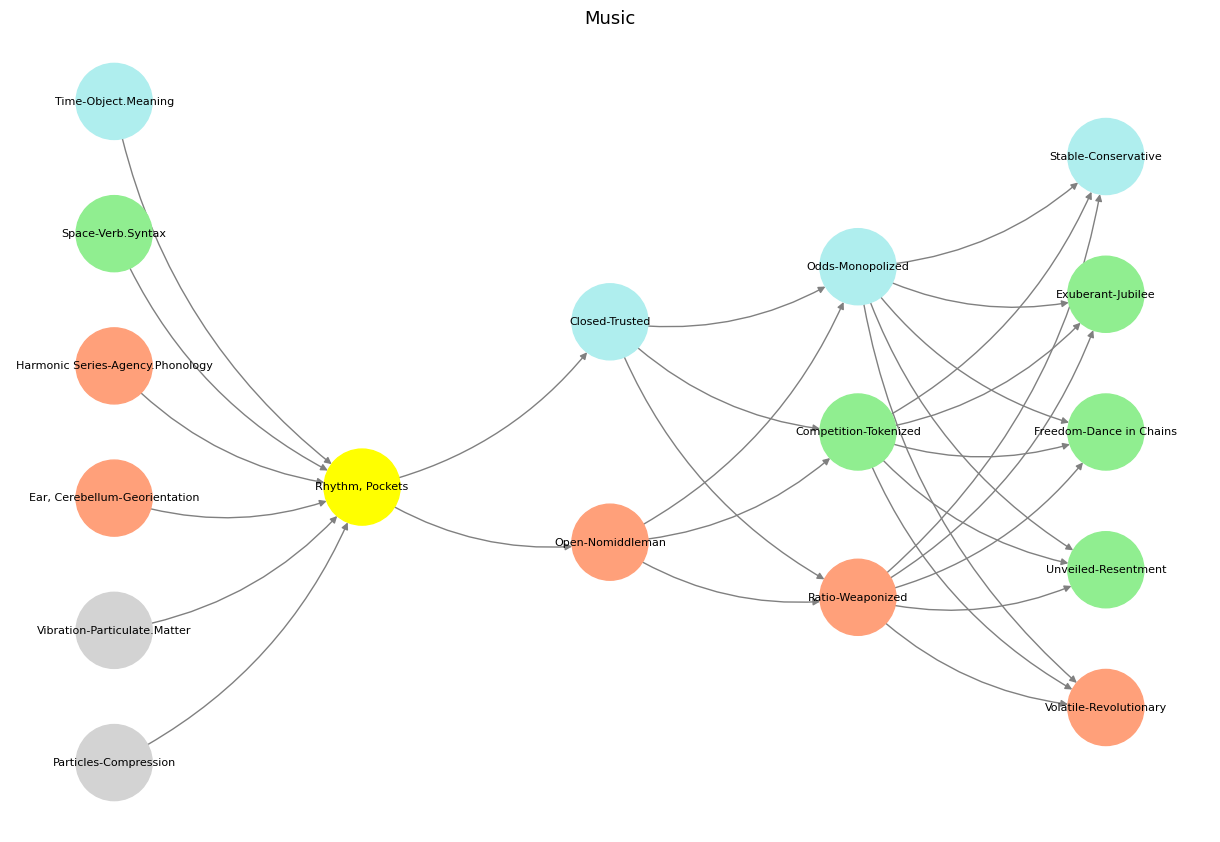
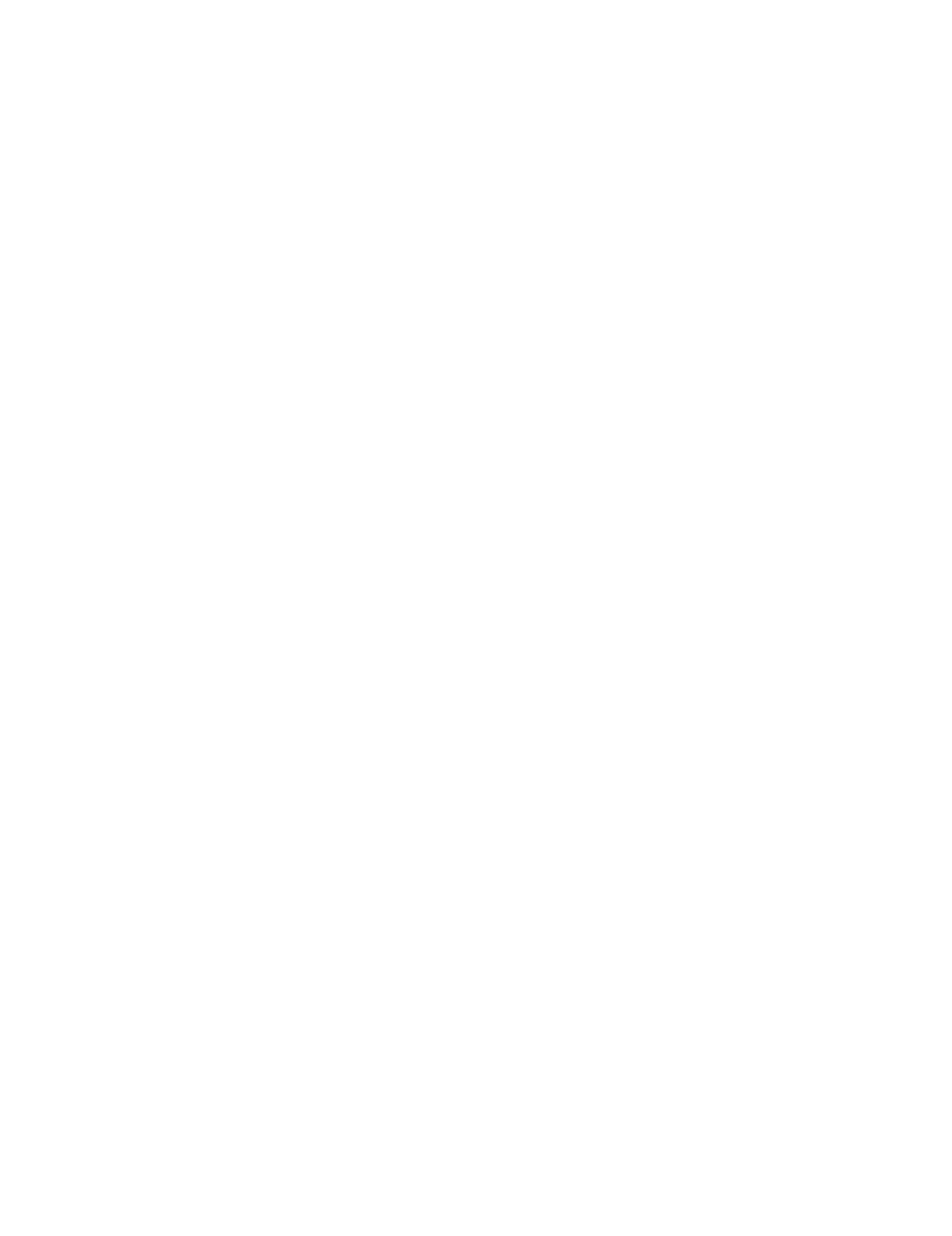
Fig. 28 Psilocybin is itself biologically inactive but is quickly converted by the body to psilocin, which has mind-altering effects similar, in some aspects, to those of other classical psychedelics. Effects include euphoria, hallucinations, changes in perception
, a distorted sense of time
, and perceived spiritual experiences. It can also cause adverse reactions such as nausea and panic attacks. In Nahuatl, the language of the Aztecs, the mushrooms were called teonanácatl—literally “divine mushroom.” Source: Wikipedia#