Resilience ๐ก๏ธโค๏ธ๐ฐ#
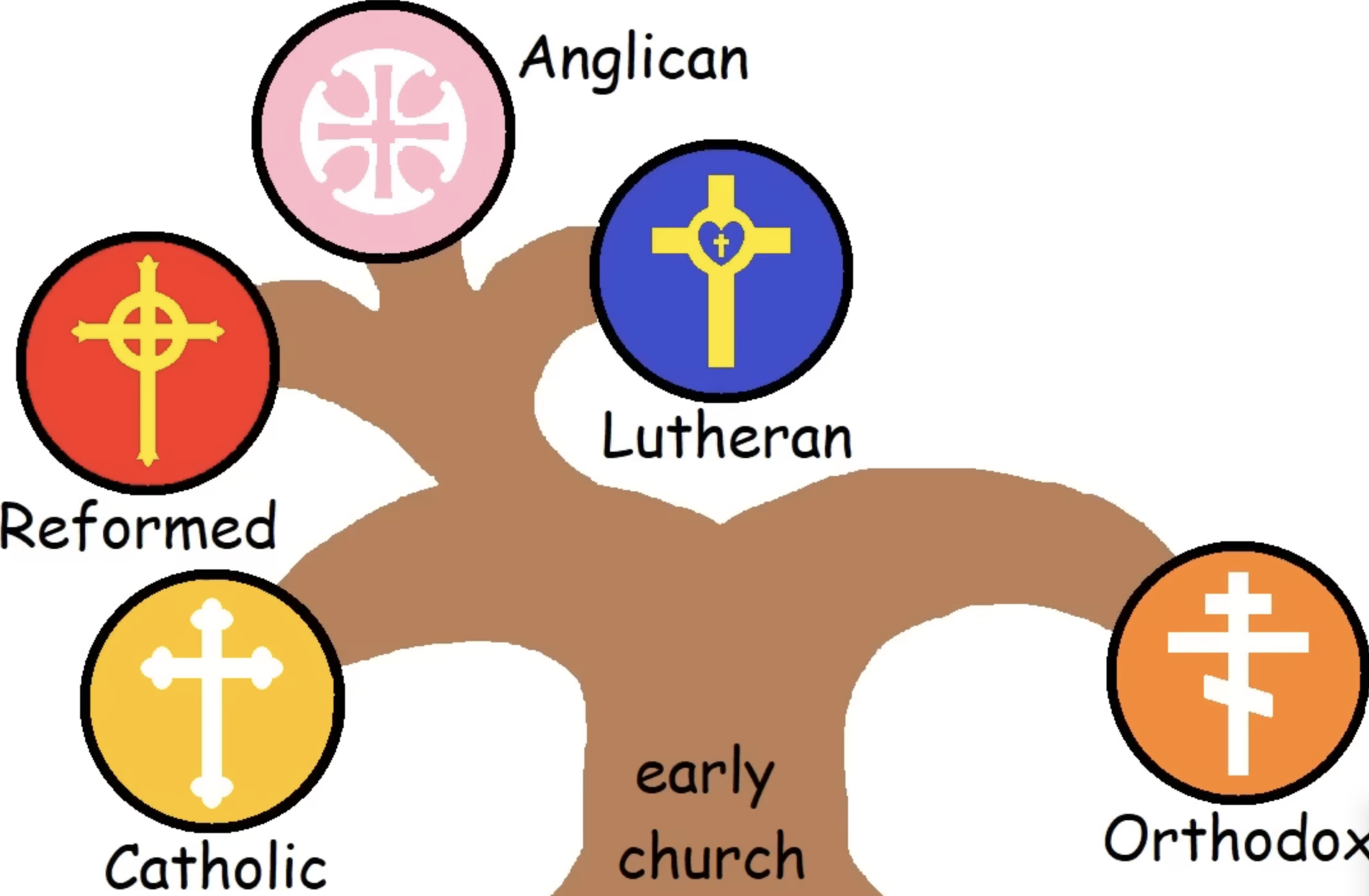
Fig. 8 Neural Anatomy of Music: What Exactly Is It About? Might it be about fixed odds, pattern recognition, leveraged agency, curtailed agency, or spoils for further play? Grants certainly are part of the spoils for further play. And perhaps bits of the other stuff.#
Show code cell source
import numpy as np
import matplotlib.pyplot as plt
import networkx as nx
# Define the neural network fractal
def define_layers():
return {
'World': ['Particles-Compression', 'Vibration-Particulate.Matter', 'Ear, Cerebellum-Georientation', 'Harmonic Series-Agency.Phonology', 'Space-Verb.Syntax', 'Time-Object.Meaning', ], # Resources
'Perception': ['Rhythm, Pockets'], # Needs
'Agency': ['Open-Nomiddleman', 'Closed-Trusted'], # Costs
'Generative': ['Ratio-Weaponized', 'Competition-Tokenized', 'Odds-Monopolized'], # Means
'Physical': ['Volatile-Revolutionary', 'Unveiled-Resentment', 'Freedom-Dance in Chains', 'Exuberant-Jubilee', 'Stable-Conservative'] # Ends
}
# Assign colors to nodes
def assign_colors():
color_map = {
'yellow': ['Rhythm, Pockets'],
'paleturquoise': ['Time-Object.Meaning', 'Closed-Trusted', 'Odds-Monopolized', 'Stable-Conservative'],
'lightgreen': ['Space-Verb.Syntax', 'Competition-Tokenized', 'Exuberant-Jubilee', 'Freedom-Dance in Chains', 'Unveiled-Resentment'],
'lightsalmon': [
'Ear, Cerebellum-Georientation', 'Harmonic Series-Agency.Phonology', 'Open-Nomiddleman',
'Ratio-Weaponized', 'Volatile-Revolutionary'
],
}
return {node: color for color, nodes in color_map.items() for node in nodes}
# Calculate positions for nodes
def calculate_positions(layer, x_offset):
y_positions = np.linspace(-len(layer) / 2, len(layer) / 2, len(layer))
return [(x_offset, y) for y in y_positions]
# Create and visualize the neural network graph
def visualize_nn():
layers = define_layers()
colors = assign_colors()
G = nx.DiGraph()
pos = {}
node_colors = []
# Add nodes and assign positions
for i, (layer_name, nodes) in enumerate(layers.items()):
positions = calculate_positions(nodes, x_offset=i * 2)
for node, position in zip(nodes, positions):
G.add_node(node, layer=layer_name)
pos[node] = position
node_colors.append(colors.get(node, 'lightgray')) # Default color fallback
# Add edges (automated for consecutive layers)
layer_names = list(layers.keys())
for i in range(len(layer_names) - 1):
source_layer, target_layer = layer_names[i], layer_names[i + 1]
for source in layers[source_layer]:
for target in layers[target_layer]:
G.add_edge(source, target)
# Draw the graph
plt.figure(figsize=(12, 8))
nx.draw(
G, pos, with_labels=True, node_color=node_colors, edge_color='gray',
node_size=3000, font_size=8, connectionstyle="arc3,rad=0.2"
)
plt.title("Music", fontsize=13)
plt.show()
# Run the visualization
visualize_nn()
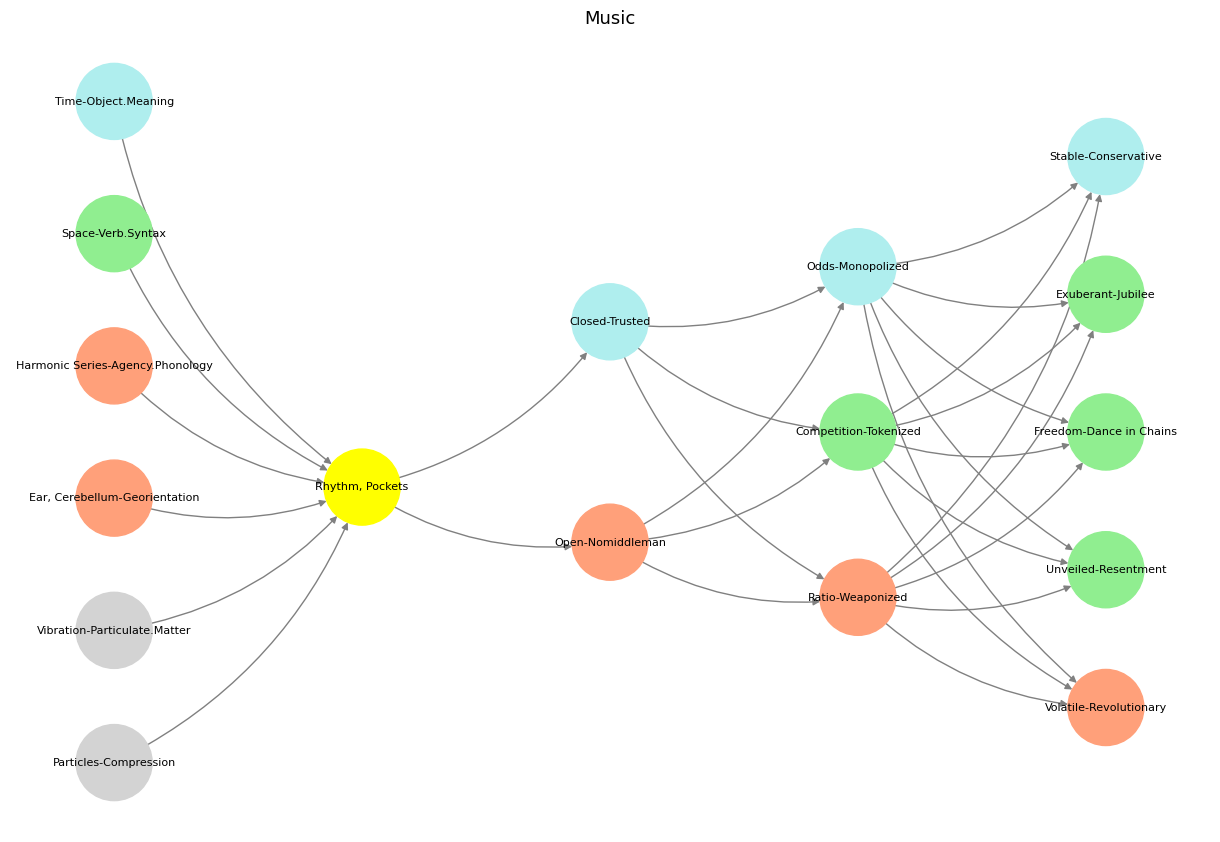
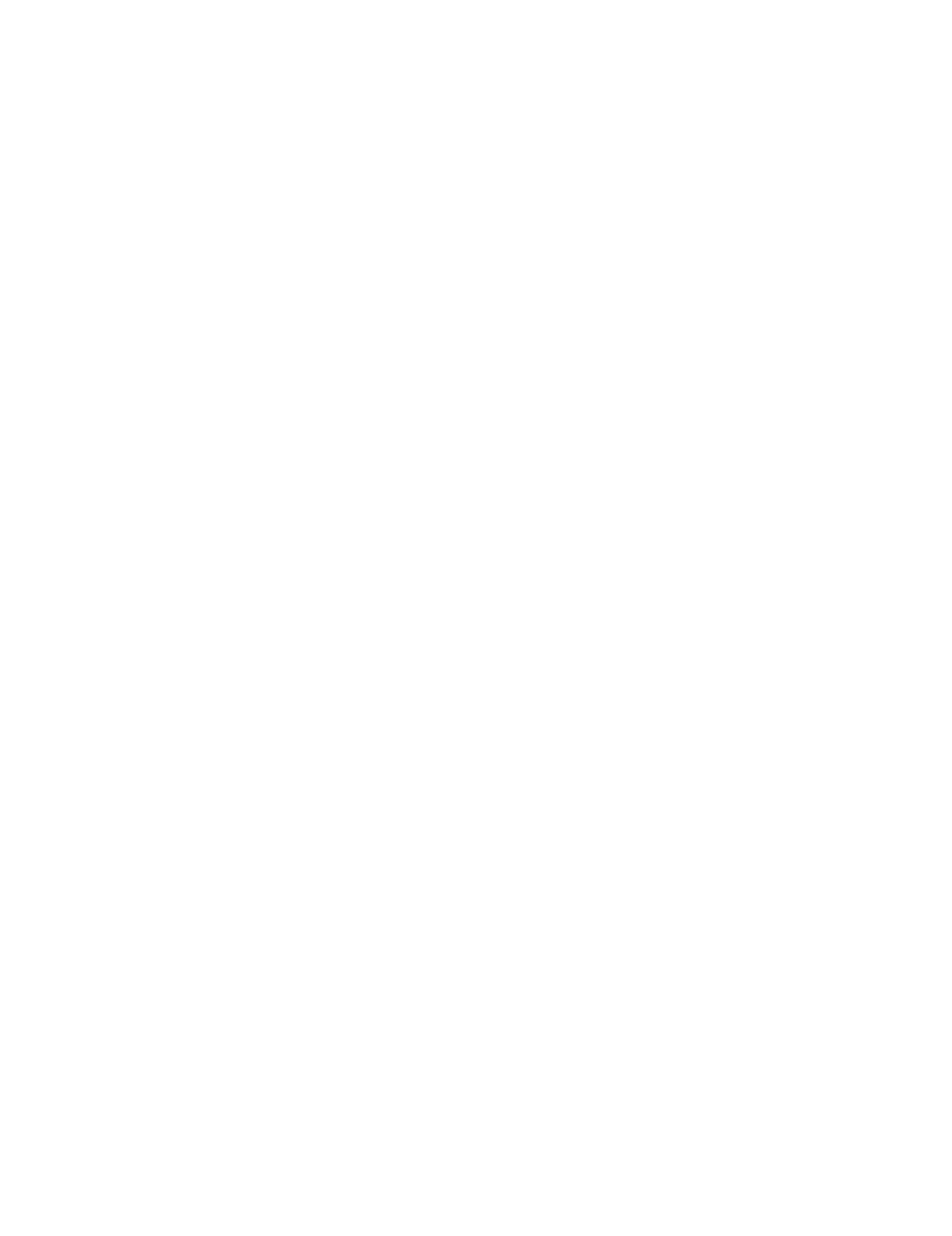
Fig. 9 Resources, Needs, Costs, Means, Ends. This is an updated version of the script with annotations tying the neural network layers, colors, and nodes to specific moments in Vita รจ Bella, enhancing the connection to the filmโs narrative and themes:#