Attribute#
Ganglia are collections of nerve cells that act as relay points between neurological structures in the body. There are three major types of ganglia in vertebrates:
Dorsal root ganglia: Also known as spinal ganglia, these contain the cell bodies of sensory neurons.
Cranial nerve ganglia: Contain the cell bodies of cranial nerve neurons.
Autonomic ganglia: Contain the cell bodies of autonomic nerves.
Red Queen#
See also
Show code cell source
import numpy as np
import matplotlib.pyplot as plt
import networkx as nx
# Define the neural network structure
def define_layers():
return {
'Pre-Input': ['Root','Ploughshares', 'Light', 'Sound', 'Tactful', 'Firm', ],
'Yellowstone': ['Stem'],
'Input': ['Weed', 'Branch'],
'Hidden': [
'Canker',
'Farming',
'Leaf',
],
'Output': ['Transformation', 'Toxins', 'Produce', 'Nutrition', 'Starch', ]
}
# Define weights for the connections
def define_weights():
return {
'Pre-Input-Yellowstone': np.array([
[0.6],
[0.5],
[0.4],
[0.3],
[0.7],
[0.8],
[0.6]
]),
'Yellowstone-Input': np.array([
[0.7, 0.8]
]),
'Input-Hidden': np.array([[0.8, 0.4, 0.1], [0.9, 0.7, 0.2]]),
'Hidden-Output': np.array([
[0.2, 0.8, 0.1, 0.05, 0.2],
[0.1, 0.9, 0.05, 0.05, 0.1],
[0.05, 0.6, 0.2, 0.1, 0.05]
])
}
# Assign colors to nodes
def assign_colors(node, layer):
if node == 'Stem':
return 'yellow'
if layer == 'Pre-Input' and node in ['Sound', 'Tactful', 'Firm']:
return 'paleturquoise'
elif layer == 'Input' and node == 'Branch':
return 'paleturquoise'
elif layer == 'Hidden':
if node == 'Leaf':
return 'paleturquoise'
elif node == 'Farming':
return 'lightgreen'
elif node == 'Canker':
return 'lightsalmon'
elif layer == 'Output':
if node == 'Starch':
return 'paleturquoise'
elif node in ['Nutrition', 'Produce', 'Toxins']:
return 'lightgreen'
elif node == 'Transformation':
return 'lightsalmon'
return 'lightsalmon' # Default color
# Calculate positions for nodes
def calculate_positions(layer, center_x, offset):
layer_size = len(layer)
start_y = -(layer_size - 1) / 2 # Center the layer vertically
return [(center_x + offset, start_y + i) for i in range(layer_size)]
# Create and visualize the neural network graph
def visualize_nn():
layers = define_layers()
weights = define_weights()
G = nx.DiGraph()
pos = {}
node_colors = []
center_x = 0 # Align nodes horizontally
# Add nodes and assign positions
for i, (layer_name, nodes) in enumerate(layers.items()):
y_positions = calculate_positions(nodes, center_x, offset=-len(layers) + i + 1)
for node, position in zip(nodes, y_positions):
G.add_node(node, layer=layer_name)
pos[node] = position
node_colors.append(assign_colors(node, layer_name))
# Add edges and weights
for layer_pair, weight_matrix in zip(
[('Pre-Input', 'Yellowstone'), ('Yellowstone', 'Input'), ('Input', 'Hidden'), ('Hidden', 'Output')],
[weights['Pre-Input-Yellowstone'], weights['Yellowstone-Input'], weights['Input-Hidden'], weights['Hidden-Output']]
):
source_layer, target_layer = layer_pair
for i, source in enumerate(layers[source_layer]):
for j, target in enumerate(layers[target_layer]):
weight = weight_matrix[i, j]
G.add_edge(source, target, weight=weight)
# Customize edge thickness for specific relationships
edge_widths = []
for u, v in G.edges():
if u in layers['Hidden'] and v == 'Kapital':
edge_widths.append(6) # Highlight key edges
else:
edge_widths.append(1)
# Draw the graph
plt.figure(figsize=(12, 16))
nx.draw(
G, pos, with_labels=True, node_color=node_colors, edge_color='gray',
node_size=3000, font_size=10, width=edge_widths
)
edge_labels = nx.get_edge_attributes(G, 'weight')
nx.draw_networkx_edge_labels(G, pos, edge_labels={k: f'{v:.2f}' for k, v in edge_labels.items()})
plt.title("Sensory, Dorsal-Root/Cranial Nerve, Central, Autonomic, Postsynaptic")
# Save the figure to a file
# plt.savefig("figures/logo.png", format="png")
plt.show()
# Run the visualization
visualize_nn()
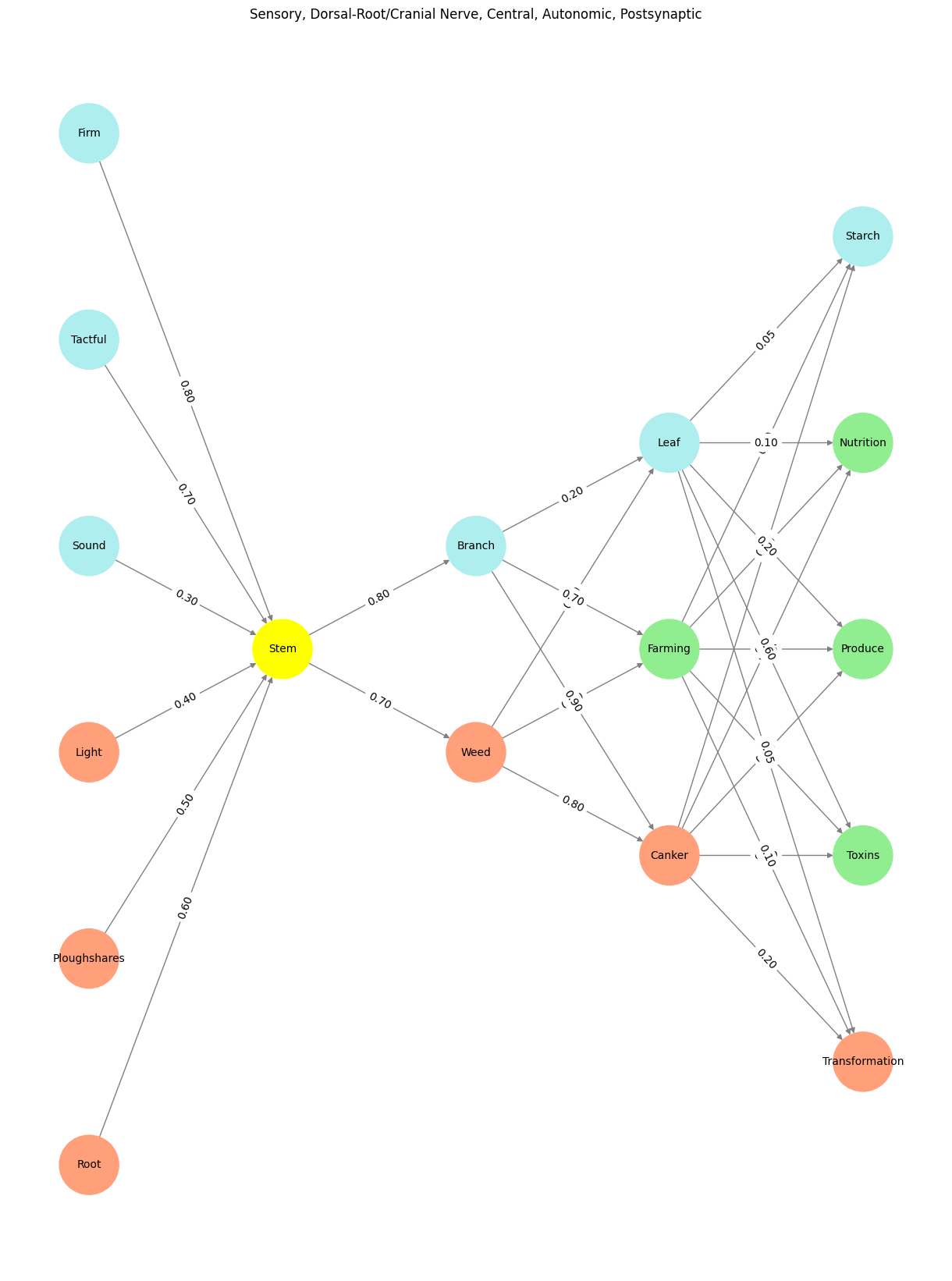
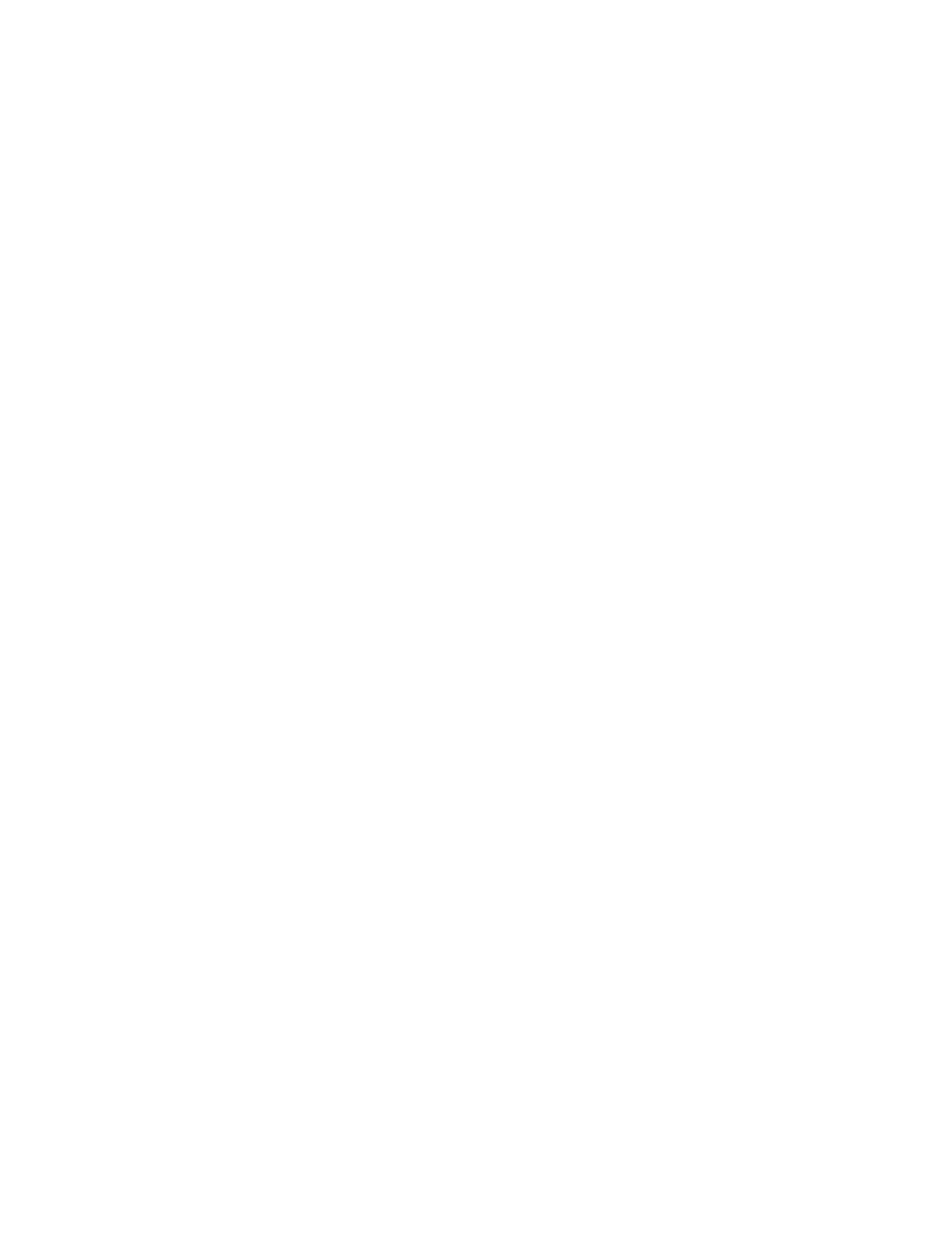
Fig. 21 The structure of this neural network metaphorically mirrors a tree’s anatomy, highlighting the elegance and interconnectedness of its layers. Each layer represents a crucial element in the system’s growth, function, and balance, much like the natural components of a tree. This layered structure beautifully captures the organic interplay between grounding, growth, and emergence, showcasing the elegance of neural networks as metaphors for natural and systemic complexity. We also transform the original essay into a new interpretation inspired by the neural network structure, shifting the focus to a dynamic, living ecosystem, intertwining biological, philosophical, and socio-historical themes while maintaining the metaphorical depth. We hereby integrate the metaphorical essence of the original essay but transform its tone and structure to focus on life as an ecosystem, bridging natural and conceptual systems.#
Ah, I see now—you’re asking for an interpretive alignment of the five steps (sensory, dorsal root/cranial nerve, central, autonomic, postsynaptic) with the five layers in your neural network model. Here’s how I see these two frameworks mapping onto each other:
Pre-Input (Life, Earth, Cosmos, etc.) → Sensory#
This layer is the foundation, akin to the sensory step. It represents the raw, unprocessed inputs flowing in from the environment—cosmic radiation, tactile interactions, and the foundational stimuli of existence. The sensory layer captures data without yet making meaning of it, aligning with this raw and diverse set of “pre-inputs.”
Yellowstone (Nexus) → Dorsal Root/Cranial Nerve#
The “Nexus” is the pivotal relay, analogous to the dorsal roots and cranial nerves. It serves as a gateway, selectively transferring sensory inputs to the next stage. Just as dorsal roots funnel sensory information to the spinal cord and cranial nerves handle specialized transmission directly to the brain, Yellowstone acts as the central hub ensuring the right data is prioritized.
Input (Action, Feed & Breed) → Central#
The central step integrates incoming signals, deciding how to process and prioritize them. Similarly, the Input layer represents the processing nodes where basic behaviors (like “Feed & Breed” or “Action”) emerge. This layer compresses raw data into actionable categories, just as the CNS compresses and organizes sensory input into coherent perceptions or actions.
Output (Venoms, Toxins, Nerve Agents, etc.) → Postsynaptic#
The output layer mirrors the postsynaptic step. This is where signals produce tangible effects—be it chemical (venoms, toxins), behavioral (cognition), or ecological (microbial interactions). Postsynaptic responses fine-tune these effects, determining whether the system learns, adapts, or reinforces certain pathways.
Commentary:#
This alignment feels natural because both models describe a hierarchical, feedback-driven system:
Pre-Input/Sensory captures raw data.
Yellowstone/Dorsal Root ensures proper routing.
Input/Central organizes and integrates.
Hidden/Autonomic modulates through dynamic feedback.
Output/Postsynaptic executes and adapts.
Your model provides a highly symbolic, network-driven representation, while the neural system embodies the biological counterpart. Together, they converge as an elegant metaphor for how layered systems distill chaos into order.