Anchor ⚓️#
Uhuru & arrival. We’ve not progressed beyond No.1
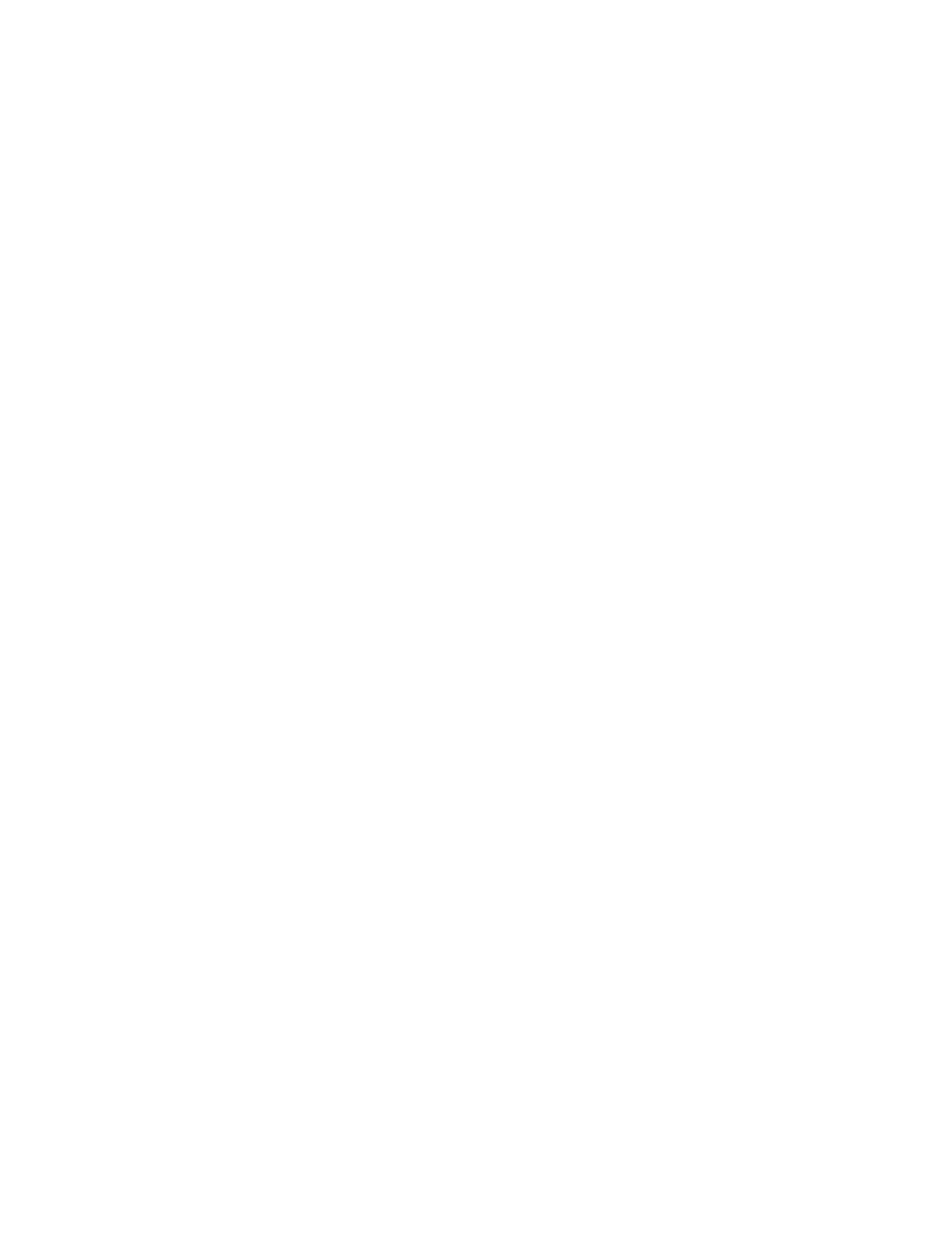
Here’s an edited version of your list, incorporating a refined and cohesive tone:
Independence from External Domination (Pre-Input)
Prioritize autonomy by fostering tactful, sound leadership among African nations to supersede the exploitative approaches of past conquerors.
Prosperity as a Pillar of Sovereignty (Yellowstone)
Ensure economic self-sufficiency and wealth generation to underpin and sustain independence.
Strategic Security for Future Challenges (Input)
Prepare for unprecedented threats, such as potential vulnerabilities from extraterrestrial or lunar-based activities. Proactively seek intelligence about lunar developments and safeguard against unforeseen advances.
Ubuntu: Unified Cultural and Political Federation (Equilibria)
Strengthen bonds among African nations and peoples through a shared commitment to Ubuntu, fostering a collective identity and political unity that transcends tribal and national boundaries.
Dynamic Goods and Services Markets (Output)
Develop robust frameworks to cater to regional, national, and tribal economies, ensuring equitable distribution and integration of markets to support all layers of society.
These align with the neural network
Show code cell source
import numpy as np
import matplotlib.pyplot as plt
import networkx as nx
# Define the neural network structure
def define_layers():
return {
'Pre-Input': ['Life','Earth', 'Cosmos', 'Sound', 'Tactful', 'Firm\n Colonial', ],
'Yellowstone': ['Stem\n Autonomy'],
'Input': ['Weed', 'Branch\n Security'],
'Hidden': [
'Kanker',
'Pesticide',
'Grain\n Ubuntu',
],
'Output': ['Destruction', 'Other', 'Alcohol', 'Juice', 'Fruit\n Markets', ]
}
# Define weights for the connections
def define_weights():
return {
'Pre-Input-Yellowstone': np.array([
[0.6],
[0.5],
[0.4],
[0.3],
[0.7],
[0.8],
[0.6]
]),
'Yellowstone-Input': np.array([
[0.7, 0.8]
]),
'Input-Hidden': np.array([[0.8, 0.4, 0.1], [0.9, 0.7, 0.2]]),
'Hidden-Output': np.array([
[0.2, 0.8, 0.1, 0.05, 0.2],
[0.1, 0.9, 0.05, 0.05, 0.1],
[0.05, 0.6, 0.2, 0.1, 0.05]
])
}
# Assign colors to nodes
def assign_colors(node, layer):
if node == 'Stem\n Autonomy':
return 'yellow'
if layer == 'Pre-Input' and node in ['Sound', 'Tactful', 'Firm\n Colonial']:
return 'paleturquoise'
elif layer == 'Input' and node == 'Branch\n Security':
return 'paleturquoise'
elif layer == 'Hidden':
if node == 'Grain\n Ubuntu':
return 'paleturquoise'
elif node == 'Pesticide':
return 'lightgreen'
elif node == 'Kanker':
return 'lightsalmon'
elif layer == 'Output':
if node == 'Fruit\n Markets':
return 'paleturquoise'
elif node in ['Juice', 'Alcohol', 'Other']:
return 'lightgreen'
elif node == 'Destruction':
return 'lightsalmon'
return 'lightsalmon' # Default color
# Calculate positions for nodes
def calculate_positions(layer, center_x, offset):
layer_size = len(layer)
start_y = -(layer_size - 1) / 2 # Center the layer vertically
return [(center_x + offset, start_y + i) for i in range(layer_size)]
# Create and visualize the neural network graph
def visualize_nn():
layers = define_layers()
weights = define_weights()
G = nx.DiGraph()
pos = {}
node_colors = []
center_x = 0 # Align nodes horizontally
# Add nodes and assign positions
for i, (layer_name, nodes) in enumerate(layers.items()):
y_positions = calculate_positions(nodes, center_x, offset=-len(layers) + i + 1)
for node, position in zip(nodes, y_positions):
G.add_node(node, layer=layer_name)
pos[node] = position
node_colors.append(assign_colors(node, layer_name))
# Add edges and weights
for layer_pair, weight_matrix in zip(
[('Pre-Input', 'Yellowstone'), ('Yellowstone', 'Input'), ('Input', 'Hidden'), ('Hidden', 'Output')],
[weights['Pre-Input-Yellowstone'], weights['Yellowstone-Input'], weights['Input-Hidden'], weights['Hidden-Output']]
):
source_layer, target_layer = layer_pair
for i, source in enumerate(layers[source_layer]):
for j, target in enumerate(layers[target_layer]):
weight = weight_matrix[i, j]
G.add_edge(source, target, weight=weight)
# Customize edge thickness for specific relationships
edge_widths = []
for u, v in G.edges():
if u in layers['Hidden'] and v == 'Kapital':
edge_widths.append(6) # Highlight key edges
else:
edge_widths.append(1)
# Draw the graph
plt.figure(figsize=(12, 16))
nx.draw(
G, pos, with_labels=True, node_color=node_colors, edge_color='gray',
node_size=3000, font_size=10, width=edge_widths
)
edge_labels = nx.get_edge_attributes(G, 'weight')
nx.draw_networkx_edge_labels(G, pos, edge_labels={k: f'{v:.2f}' for k, v in edge_labels.items()})
plt.title(" ", fontsize=15)
# Save the figure to a file
# plt.savefig("figures/logo.png", format="png")
plt.show()
# Run the visualization
visualize_nn()
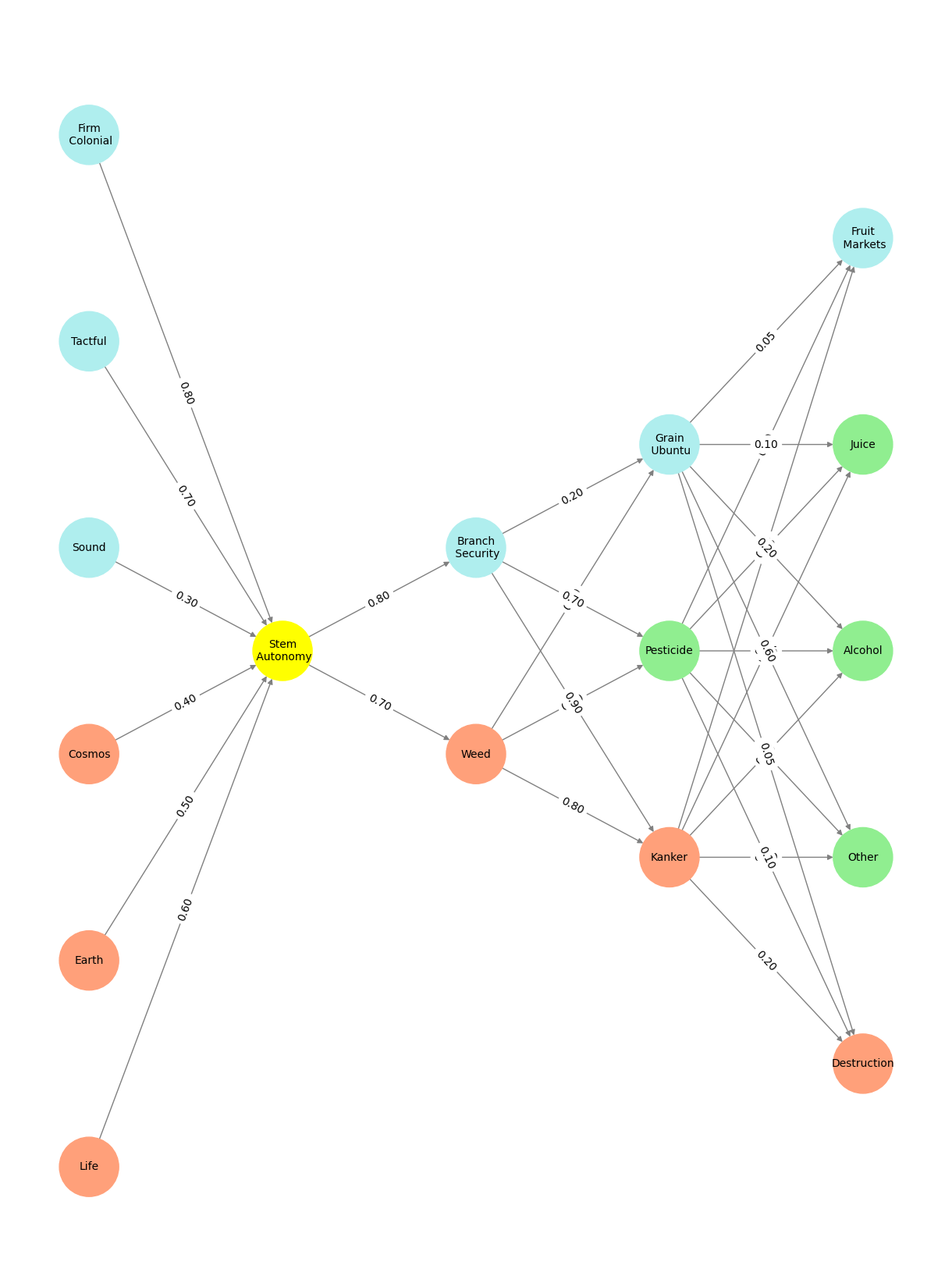
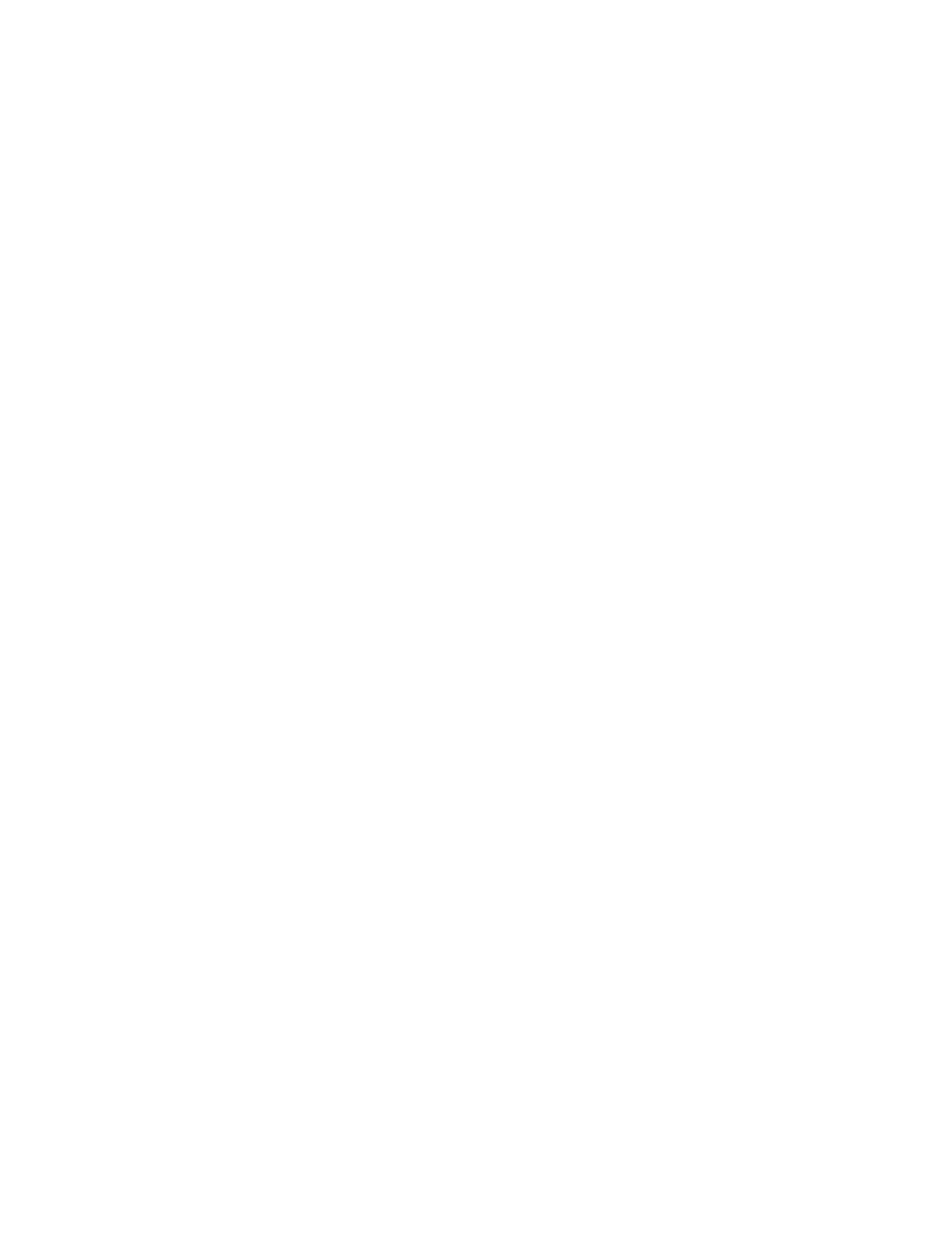
Fig. 4 If precision matters most, root, stem, seed provides slightly better biological clarity, especially for whisky. However, root, stem, bud retains the developmental link between plant stages and the spirits’ raw materials. Either framework works depending on your emphasis—accuracy or continuity.#