Engineering#
Art Neural Network Theory#
Artistic Neural Network Framework with Examples#
Pre-Input Layer (Cosmos, Earth, Life, Firmness, Tactful, Soundness)#
Cosmos: 2001: A Space Odyssey by Stanley Kubrick represents the awe-inspiring vastness of the cosmos, from the monolith’s role in human evolution to the journey beyond the infinite.
Earth: Dr. Zhivago by David Lean captures Earth’s brutal yet breathtaking extremes through the cold, sweeping landscapes of Russia during historical upheaval.
Life: The Revenant by Alejandro González Iñárritu embodies the primal struggle for survival against the natural world, including encounters with animals like the infamous bear.
Firmness: The Old Man and the Sea by Ernest Hemingway reflects human resilience and firmness against the forces of nature, through Santiago’s unwavering struggle with the marlin.
Tactful: The Godfather by Francis Ford Coppola explores tactfulness in human interaction, diplomacy, and strategy within a crime family’s dynamics.
Soundness: The Iliad by Homer represents monumental history and the soundness of epic storytelling, where the events of the Trojan War echo timeless truths.
Yellowstone Layer (Nostalgia and Compression)#
Je ne sais quoi / Nostalgia: Citizen Kane by Orson Welles epitomizes nostalgia through the symbolic power of “Rosebud,” a single object compressing a lifetime of memory and longing.
Input Layer (Protagonist and Antagonist)#
Protagonist (Blue): The Shawshank Redemption by Frank Darabont celebrates the indomitable spirit of Andy Dufresne as a protagonist who embodies hope and perseverance.
Antagonist (Red): No Country for Old Men by the Coen Brothers presents Anton Chigurh as a chilling antagonist representing chaos and fate.
Output Layer (Psychological Emergence)#
Industrial: Modern Times by Charlie Chaplin critiques industrialization’s psychological toll on individuals, blending humor with pathos.
Future: Blade Runner by Ridley Scott contemplates the future of humanity, artificial intelligence, and the psychology of identity and memory.
Prosecuted: The Trial by Franz Kafka delves into the psychological terror of bureaucracy and justice, encapsulating themes of guilt and alienation.
History: War and Peace by Leo Tolstoy reflects the psychological impact of history’s grand sweep on individuals caught in its tides.
Slavery: 12 Years a Slave by Steve McQueen captures the devastating psychological impact of slavery on individuals and their humanity.
This neural network offers a dynamic way to explore the intersection of art and human endeavor, framing films, novels, and plays as expressions of universal and emergent truths. Each node represents a unique facet of existence, interconnected within this creative framework.
Show code cell source
import numpy as np
import matplotlib.pyplot as plt
import networkx as nx
# Define the neural network structure
def define_layers():
return {
'Pre-Input': ['Cosmos', 'Earth', 'Life', 'Firm', 'Tactful', 'Sound', ],
'Yellowstone': ['Je ne sais quoi\n Nostalgia\n Gone with Wind'],
'Input': ['Yankee', 'Confederacy'],
'Hidden': [
'Disturbing',
'Law',
'Peace',
],
'Output': ['Industrial', 'Future', 'Prosecuted', 'History', 'Slavery', ]
}
# Define weights for the connections
def define_weights():
return {
'Pre-Input-Yellowstone': np.array([
[0.6],
[0.5],
[0.4],
[0.3],
[0.7],
[0.8],
[0.6]
]),
'Yellowstone-Input': np.array([
[0.7, 0.8]
]),
'Input-Hidden': np.array([[0.8, 0.4, 0.1], [0.9, 0.7, 0.2]]),
'Hidden-Output': np.array([
[0.2, 0.8, 0.1, 0.05, 0.2],
[0.1, 0.9, 0.05, 0.05, 0.1],
[0.05, 0.6, 0.2, 0.1, 0.05]
])
}
# Assign colors to nodes
def assign_colors(node, layer):
if node == 'Je ne sais quoi\n Nostalgia\n Gone with Wind':
return 'yellow'
if layer == 'Pre-Input' and node in ['Sound', ]:
return 'paleturquoise'
elif layer == 'Pre-Input' and node in ['Tactful', ]:
return 'lightgreen'
elif layer == 'Input' and node == 'Confederacy':
return 'paleturquoise'
elif layer == 'Hidden':
if node == 'Peace':
return 'paleturquoise'
elif node == 'Law':
return 'lightgreen'
elif node == 'Disturbing':
return 'lightsalmon'
elif layer == 'Output':
if node == 'Slavery':
return 'paleturquoise'
elif node in ['History', 'Prosecuted', 'Future']:
return 'lightgreen'
elif node == 'Industrial':
return 'lightsalmon'
return 'lightsalmon' # Default color
# Calculate positions for nodes
def calculate_positions(layer, center_x, offset):
layer_size = len(layer)
start_y = -(layer_size - 1) / 2 # Center the layer vertically
return [(center_x + offset, start_y + i) for i in range(layer_size)]
# Create and visualize the neural network graph
def visualize_nn():
layers = define_layers()
weights = define_weights()
G = nx.DiGraph()
pos = {}
node_colors = []
center_x = 0 # Align nodes horizontally
# Add nodes and assign positions
for i, (layer_name, nodes) in enumerate(layers.items()):
y_positions = calculate_positions(nodes, center_x, offset=-len(layers) + i + 1)
for node, position in zip(nodes, y_positions):
G.add_node(node, layer=layer_name)
pos[node] = position
node_colors.append(assign_colors(node, layer_name))
# Add edges and weights
for layer_pair, weight_matrix in zip(
[('Pre-Input', 'Yellowstone'), ('Yellowstone', 'Input'), ('Input', 'Hidden'), ('Hidden', 'Output')],
[weights['Pre-Input-Yellowstone'], weights['Yellowstone-Input'], weights['Input-Hidden'], weights['Hidden-Output']]
):
source_layer, target_layer = layer_pair
for i, source in enumerate(layers[source_layer]):
for j, target in enumerate(layers[target_layer]):
weight = weight_matrix[i, j]
G.add_edge(source, target, weight=weight)
# Customize edge thickness for specific relationships
edge_widths = []
for u, v in G.edges():
if u in layers['Hidden'] and v == 'Kapital':
edge_widths.append(6) # Highlight key edges
else:
edge_widths.append(1)
# Draw the graph
plt.figure(figsize=(12, 16))
nx.draw(
G, pos, with_labels=True, node_color=node_colors, edge_color='gray',
node_size=3000, font_size=10, width=edge_widths
)
edge_labels = nx.get_edge_attributes(G, 'weight')
nx.draw_networkx_edge_labels(G, pos, edge_labels={k: f'{v:.2f}' for k, v in edge_labels.items()})
plt.title(" ")
# Save the figure to a file
# plt.savefig("figures/logo.png", format="png")
plt.show()
# Run the visualization
visualize_nn()
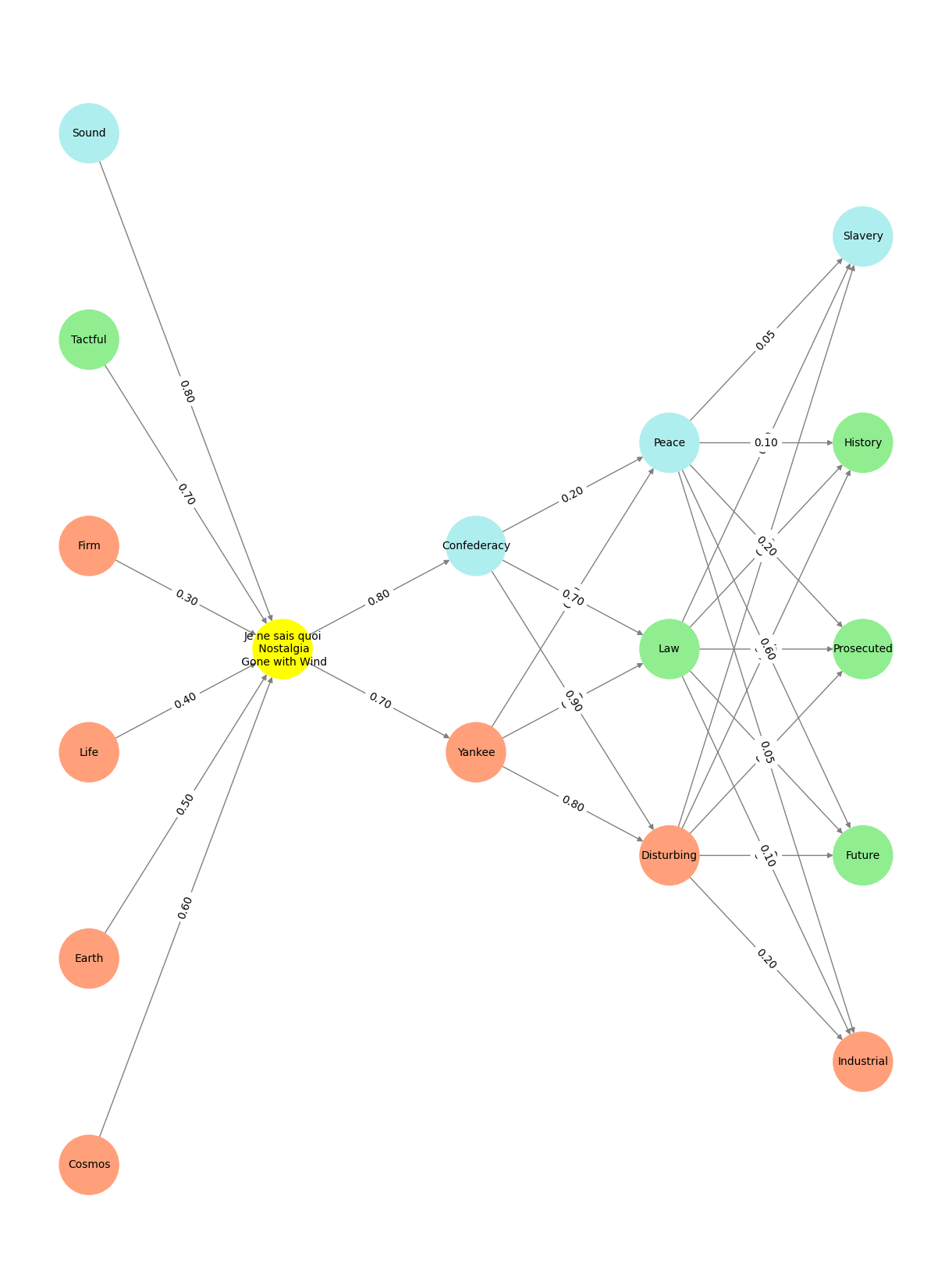
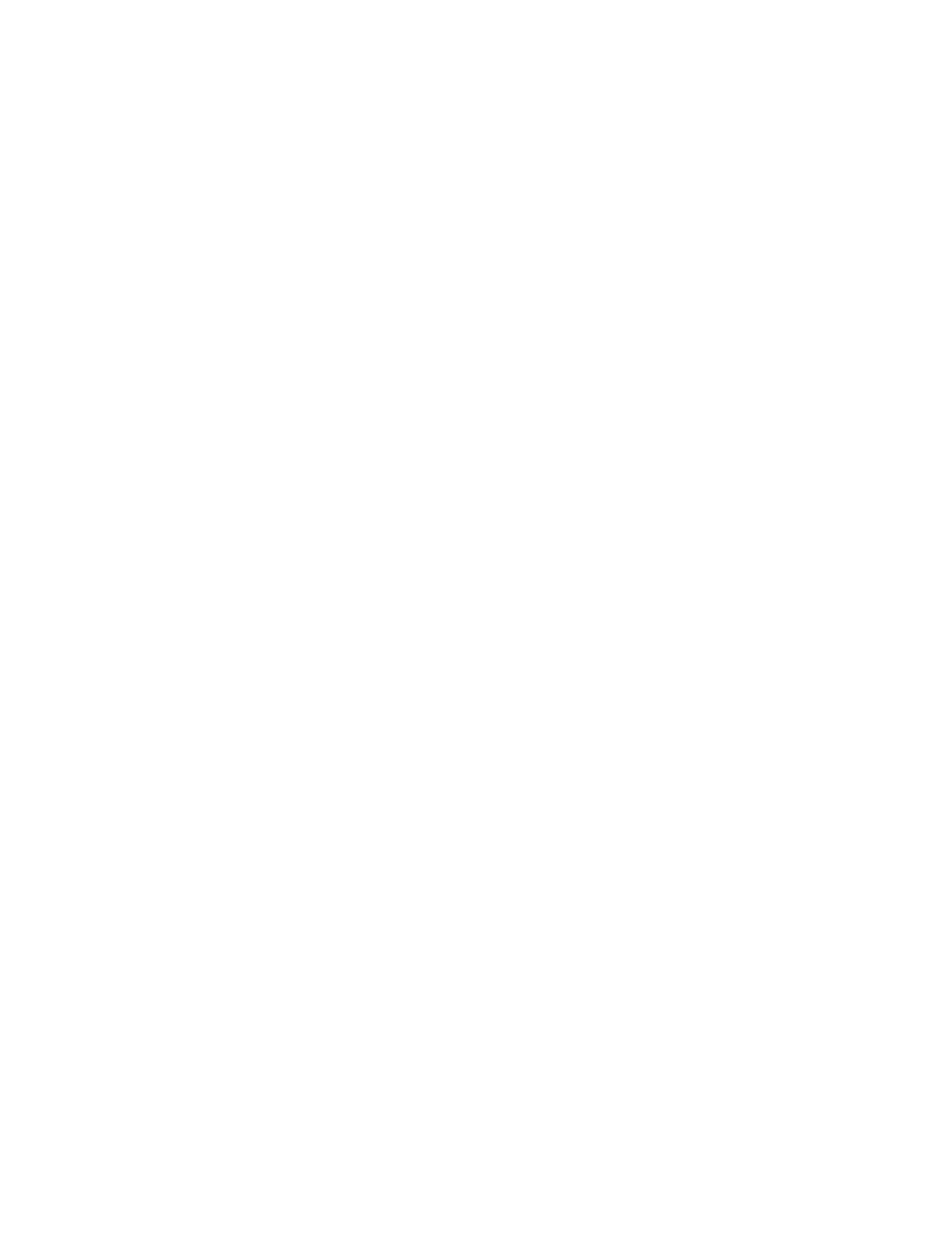
Fig. 8 The Foreign Office. It’s quite ready to go along with the European ID as a quid pro quo for a deal over the Butter Mountain, The Wine Lake, and Milk Ocean … the Lamb War and the Cod Stick (life node
). But in brief, the UK joined the EU with anarchic intent – easier to blow it up from the inside.#