Dancing in Chains#
The human immune system and the brain’s large-scale networks share deep functional parallels, each responsible for detecting threats, prioritizing responses, and restoring balance. Just as the brain operates through distinct yet interconnected circuits—such as the Task-Positive Network (TPN), the Salience Network (SN), and the Default Mode Network (DMN)—the immune system relies on pattern recognition, inflammatory signaling, and regulatory checkpoints to maintain homeostasis. The conceptual alignment between these domains is not metaphorical but structural, revealing fundamental principles of survival across both physiological and cognitive systems. The immune system’s dynamic interplay between activation and inhibition mirrors the neural oscillation between externally focused engagement and internally directed processing.

Fig. 31 Freedom in Fetters—a Princely Freedom. Chopin, the last of the modern musicians, who gazed at and worshipped beauty, like Leopardi; Chopin, the Pole, the inimitable (none that came before or after him has a right to this name)—Chopin had the same princely punctilio in convention (grammar, space) that Raphael shows in the use of the simplest traditional colours. The only difference is that Chopin applies them not to colour but to melodic and rhythmic traditions (prosody, time). He admitted the validity of these traditions because he was born under the sway of etiquette. But in these fetters he plays and dances as the freest and daintiest of spirits, and, be it observed, he does not spurn the chain. Source: Human All-Too-Human Part II#
At the core of the immune system’s response to external threats are Pattern Recognition Receptors (PRRs), including AIM2-like receptors (ALRs), RIG-I-like receptors (RLRs), NOD-like receptors (NLRs), Toll-like receptors (TLRs), and C-type lectin receptors (CLRs). These receptors serve as the immune system’s equivalent of the TPN, responsible for detecting pathogens, activating immune cells, and initiating a direct response. The TPN functions similarly, activating when an individual engages in goal-directed behavior, external problem-solving, and real-time sensory-motor coordination. Just as PRRs recognize microbial patterns and initiate inflammation, the TPN identifies task-relevant stimuli and focuses cognitive resources on action. Both systems rely on an initial stage of detection that shifts the organism from a passive state to one of active engagement.
Once an immune threat is detected, the body mobilizes a set of pro-inflammatory cytokines—IL-6, IFN-γ, and TNF-α—which drive an acute immune response. This is analogous to the Salience Network (SN) in the brain, which determines the urgency of external and internal stimuli, allocating cognitive resources accordingly. The SN, anchored in the anterior insula and dorsal anterior cingulate cortex, acts as a switch between the TPN and DMN, ensuring that the organism attends to what is most critical. IL-6, IFN-γ, and TNF-α perform the same function at the immune level, signaling distress, mobilizing resources, and directing the body’s energy toward resolving an active threat. Without these signals, both the immune and cognitive systems would fail to respond appropriately to danger.
Once a crisis has passed, the immune system relies on a set of immunosuppressive cytokines—TGF-β, IL-10, and IL-35—to downregulate inflammation and restore homeostasis. This mirrors the role of the DMN, which facilitates introspection, memory consolidation, and the regulation of self-referential thought. TGF-β is particularly critical in tissue repair and neuroprotection, much like the DMN’s role in reorganizing mental schemas after intense cognitive activity. IL-10 suppresses excessive immune responses to prevent collateral damage, just as the DMN filters out unnecessary cognitive noise, preventing over-excitation of the system. IL-35, the missing third cytokine, serves as a potent anti-inflammatory agent, further reinforcing the need for resolution once a crisis subsides. Without these regulatory factors, the immune system would remain in a state of perpetual inflammation, leading to autoimmunity, just as an unregulated DMN contributes to maladaptive rumination and psychiatric disorders.
A final layer of regulation exists in both systems—the PD-1 immune checkpoint and the brain’s executive control over the DMN. PD-1 determines whether an immune cell should remain tolerant or engage in action, acting as a gatekeeper that prevents autoimmunity. Similarly, the anterior cingulate cortex (ACC) and medial prefrontal cortex (mPFC) regulate whether the DMN should stay active or disengage in favor of task-focused cognition. In both systems, a failure in this checkpoint leads to pathological states: loss of PD-1 results in chronic immune activation, while dysregulation of the ACC and DMN is implicated in conditions like depression and schizophrenia. This checkpoint mechanism ensures that neither excessive activation nor excessive inhibition dominates, maintaining equilibrium between engagement and rest.
This structured mapping between immune regulation and neural networks reflects a deep, evolutionarily conserved logic. Both systems are built around the need to efficiently allocate resources, respond to urgent threats, and then return to baseline states of homeostasis. The parallels between PRRs and the TPN, pro-inflammatory cytokines and the SN, immunosuppressive cytokines and the DMN, and PD-1 with cognitive executive control demonstrate a unifying framework that governs survival at both cellular and cognitive scales.
Immune System Component |
Neural Network Equivalent |
Functional Role |
---|---|---|
PRRs (TLRs, NLRs, RLRs, CLRs) |
Task-Positive Network (TPN) |
Detects threats, activates response, shifts system into action. |
IL-6, IFN-γ, TNF-α |
Salience Network (SN) |
Assigns urgency, flags novel threats, prioritizes responses. |
TGF-β, IL-10, IL-35 |
Default Mode Network (DMN) |
Homeostasis, resolution, introspection, recovery. |
PD-1 |
Executive control over DMN (ACC, mPFC) |
Determines whether inhibition is maintained or lifted. |
The immune system and the brain are often studied in isolation, but their shared principles of threat detection, response prioritization, and recovery reveal a deeper, systemic connection. This framework provides a new way of understanding how cognitive states and immune function are intertwined, with dysregulation in either system leading to pathological states. Just as an overactive immune response results in autoimmunity, an overactive cognitive response—marked by an unchecked DMN or SN—can lead to psychiatric disorders. Recognizing these parallels not only enriches our understanding of both fields but also opens new avenues for interdisciplinary research in immunology, neuroscience, and even therapeutic interventions.
Tregs#
Yes, Regulatory T cells (Tregs) are a better fit than IL-35 for the Default Mode Network (DMN), because Tregs function as an active, cell-based mechanism of immune suppression, much like the DMN serves as a functional hub for cognitive inhibition, self-regulation, and systemic balance. While IL-35 is a key immunosuppressive cytokine, it operates as a signal rather than an active suppressor. Tregs, on the other hand, directly mediate immune homeostasis, controlling excessive immune responses much like the DMN controls overactive thought patterns.
Tregs as the Default Mode Network (DMN) Analog#
Tregs play a crucial role in immune homeostasis, ensuring that immune responses do not spiral into autoimmunity or chronic inflammation. The DMN plays a similar role in the brain, maintaining a baseline state of introspection, self-monitoring, and cognitive restoration. Both systems prevent excessive activation and ensure long-term stability rather than short-term reaction.
Tregs primarily exert their influence through TGF-β and IL-10, two of the key cytokines that suppress immune activity. In the nervous system, the DMN works in a similar manner, dampening external engagement in favor of internal processing, introspection, and self-referential thought. Without Tregs, the immune system loses its inhibitory control, just as without proper DMN regulation, the brain struggles with persistent ruminations, intrusive thoughts, and an inability to switch cognitive states.
Furthermore, Tregs provide a more sustained, adaptive layer of regulation compared to IL-35, which acts as an immediate cytokine-based suppressor but lacks the dynamic, cell-mediated control that Tregs provide. In the same way, the DMN is not just a passive network but an active hub coordinating brain-wide inhibition, allowing for long-term cognitive recalibration.
Updated Table: Immune System vs. Brain Networks#
Immune System Component |
Neural Network Equivalent |
Functional Role |
---|---|---|
PRRs (TLRs, NLRs, RLRs, CLRs) |
Task-Positive Network (TPN) |
Detects threats, activates response, shifts system into action. |
IL-6, IFN-γ, TNF-α |
Salience Network (SN) |
Assigns urgency, flags novel threats, prioritizes responses. |
Tregs (via TGF-β, IL-10) |
Default Mode Network (DMN) |
Homeostasis, resolution, introspection, active suppression of overactivation. |
PD-1 |
Executive control over DMN (ACC, mPFC) |
Determines whether inhibition is maintained or lifted. |
Final Thought: Tregs as the DMN’s Cellular Counterpart#
While IL-35 remains an important immunosuppressive factor, Tregs provide a more sophisticated, cell-based regulatory mechanism that mirrors the DMN’s active role in cognitive control. Just as the DMN does not merely “turn off” brain activity but instead orchestrates inhibition across multiple regions, Tregs do not simply “turn off” the immune system but dynamically regulate its response to prevent self-damage.
Thus, Tregs serve as the true immune counterpart to the DMN, ensuring long-term balance and systemic coherence.
Show code cell source
import numpy as np
import matplotlib.pyplot as plt
import networkx as nx
# Define the neural network layers
def define_layers():
return {
'Suis': ['DNA, RNA, 5%', 'Peptidoglycans, Lipoteichoics', 'Lipopolysaccharide', 'N-Formylmethionine', "Glucans, Chitin", 'Specific Antigens'], # Static
'Voir': ['PRR & ILCs, 20%'],
'Choisis': ['CD8+, 50%', 'CD4+'],
'Deviens': ['TNF-α, IL-6, IFN-γ', 'PD-1 & CTLA-4', 'Tregs, IL-10, TGF-β, 20%'],
"M'èléve": ['Complement System', 'Platelet System', 'Granulocyte System', 'Innate Lymphoid Cells, 5%', 'Adaptive Lymphoid Cells']
}
# Assign colors to nodes
def assign_colors():
color_map = {
'yellow': ['PRR & ILCs, 20%'],
'paleturquoise': ['Specific Antigens', 'CD4+', 'Tregs, IL-10, TGF-β, 20%', 'Adaptive Lymphoid Cells'],
'lightgreen': ["Glucans, Chitin", 'PD-1 & CTLA-4', 'Platelet System', 'Innate Lymphoid Cells, 5%', 'Granulocyte System'],
'lightsalmon': ['Lipopolysaccharide', 'N-Formylmethionine', 'CD8+, 50%', 'TNF-α, IL-6, IFN-γ', 'Complement System'],
}
return {node: color for color, nodes in color_map.items() for node in nodes}
# Define edge weights (hardcoded for editing)
def define_edges():
return {
('DNA, RNA, 5%', 'PRR & ILCs, 20%'): '1/99',
('Peptidoglycans, Lipoteichoics', 'PRR & ILCs, 20%'): '5/95',
('Lipopolysaccharide', 'PRR & ILCs, 20%'): '20/80',
('N-Formylmethionine', 'PRR & ILCs, 20%'): '51/49',
("Glucans, Chitin", 'PRR & ILCs, 20%'): '80/20',
('Specific Antigens', 'PRR & ILCs, 20%'): '95/5',
('PRR & ILCs, 20%', 'CD8+, 50%'): '20/80',
('PRR & ILCs, 20%', 'CD4+'): '80/20',
('CD8+, 50%', 'TNF-α, IL-6, IFN-γ'): '49/51',
('CD8+, 50%', 'PD-1 & CTLA-4'): '80/20',
('CD8+, 50%', 'Tregs, IL-10, TGF-β, 20%'): '95/5',
('CD4+', 'TNF-α, IL-6, IFN-γ'): '5/95',
('CD4+', 'PD-1 & CTLA-4'): '20/80',
('CD4+', 'Tregs, IL-10, TGF-β, 20%'): '51/49',
('TNF-α, IL-6, IFN-γ', 'Complement System'): '80/20',
('TNF-α, IL-6, IFN-γ', 'Platelet System'): '85/15',
('TNF-α, IL-6, IFN-γ', 'Granulocyte System'): '90/10',
('TNF-α, IL-6, IFN-γ', 'Innate Lymphoid Cells, 5%'): '95/5',
('TNF-α, IL-6, IFN-γ', 'Adaptive Lymphoid Cells'): '99/1',
('PD-1 & CTLA-4', 'Complement System'): '1/9',
('PD-1 & CTLA-4', 'Platelet System'): '1/8',
('PD-1 & CTLA-4', 'Granulocyte System'): '1/7',
('PD-1 & CTLA-4', 'Innate Lymphoid Cells, 5%'): '1/6',
('PD-1 & CTLA-4', 'Adaptive Lymphoid Cells'): '1/5',
('Tregs, IL-10, TGF-β, 20%', 'Complement System'): '1/99',
('Tregs, IL-10, TGF-β, 20%', 'Platelet System'): '5/95',
('Tregs, IL-10, TGF-β, 20%', 'Granulocyte System'): '10/90',
('Tregs, IL-10, TGF-β, 20%', 'Innate Lymphoid Cells, 5%'): '15/85',
('Tregs, IL-10, TGF-β, 20%', 'Adaptive Lymphoid Cells'): '20/80'
}
# Calculate positions for nodes
def calculate_positions(layer, x_offset):
y_positions = np.linspace(-len(layer) / 2, len(layer) / 2, len(layer))
return [(x_offset, y) for y in y_positions]
# Create and visualize the neural network graph
def visualize_nn():
layers = define_layers()
colors = assign_colors()
edges = define_edges()
G = nx.DiGraph()
pos = {}
node_colors = []
# Create mapping from original node names to numbered labels
mapping = {}
counter = 1
for layer in layers.values():
for node in layer:
mapping[node] = f"{counter}. {node}"
counter += 1
# Add nodes with new numbered labels and assign positions
for i, (layer_name, nodes) in enumerate(layers.items()):
positions = calculate_positions(nodes, x_offset=i * 2)
for node, position in zip(nodes, positions):
new_node = mapping[node]
G.add_node(new_node, layer=layer_name)
pos[new_node] = position
node_colors.append(colors.get(node, 'lightgray'))
# Add edges with updated node labels
for (source, target), weight in edges.items():
if source in mapping and target in mapping:
new_source = mapping[source]
new_target = mapping[target]
G.add_edge(new_source, new_target, weight=weight)
# Draw the graph
plt.figure(figsize=(12, 8))
edges_labels = {(u, v): d["weight"] for u, v, d in G.edges(data=True)}
nx.draw(
G, pos, with_labels=True, node_color=node_colors, edge_color='gray',
node_size=3000, font_size=9, connectionstyle="arc3,rad=0.2"
)
nx.draw_networkx_edge_labels(G, pos, edge_labels=edges_labels, font_size=8)
plt.title("OPRAH™: aAPCs", fontsize=25)
plt.show()
# Run the visualization
visualize_nn()
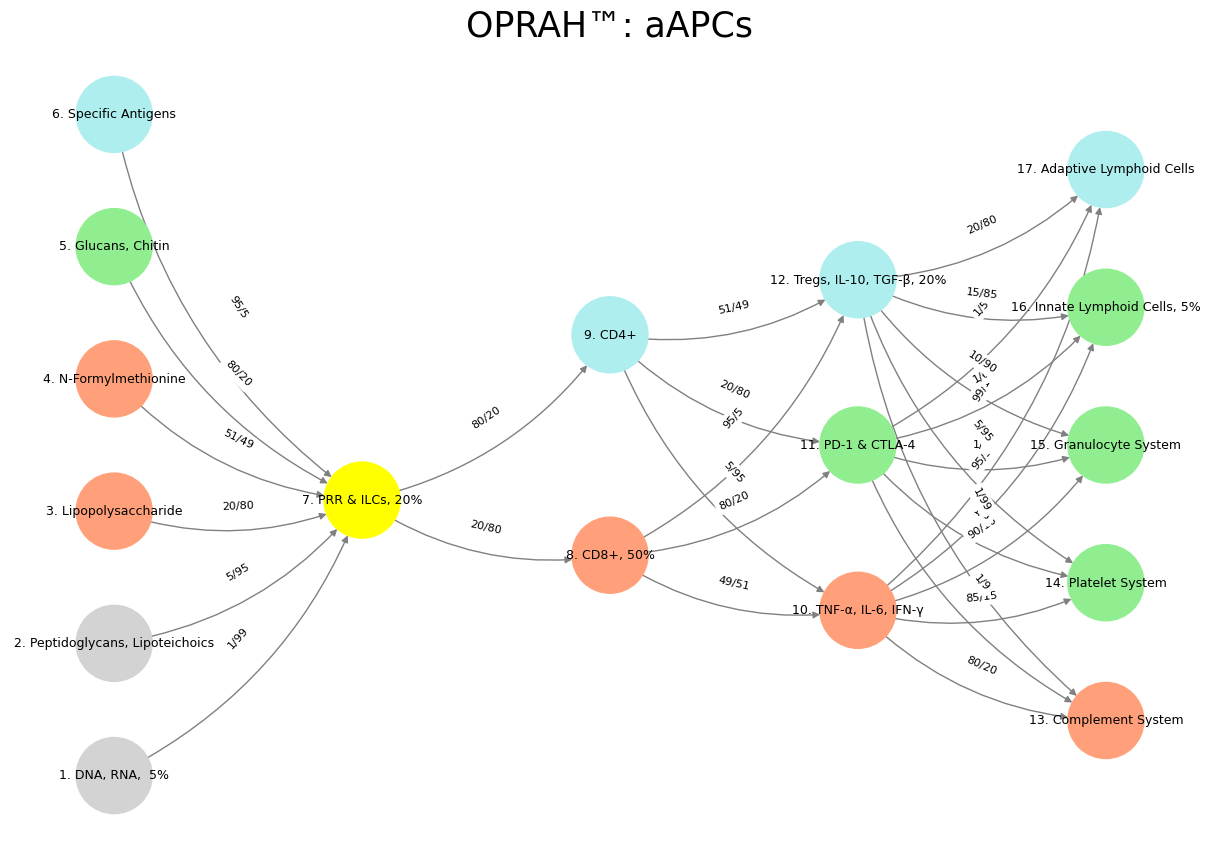
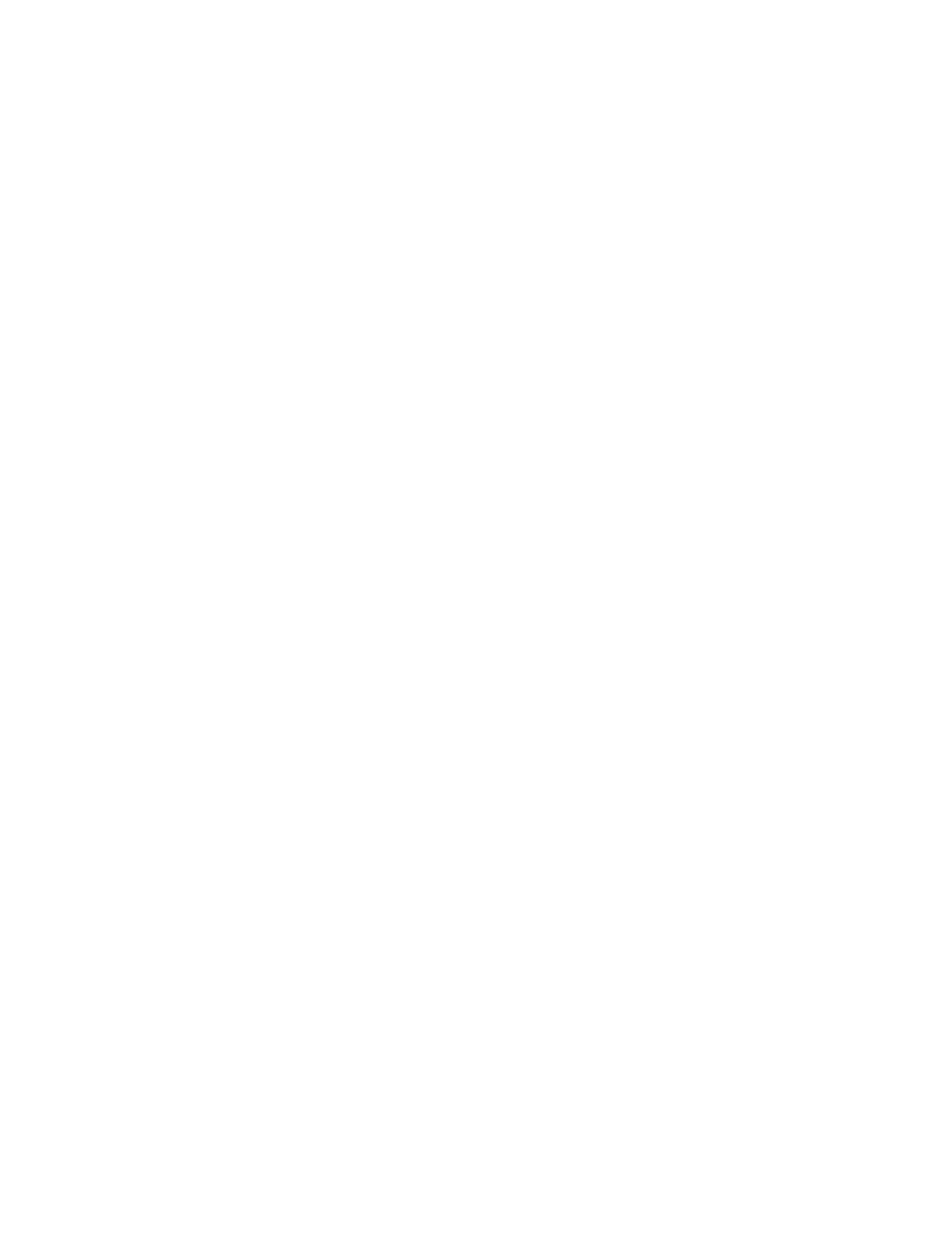
Fig. 32 G1-G3: Ganglia & N1-N5 Nuclei. These are cranial nerve, dorsal-root (G1 & G2); basal ganglia, thalamus, hypothalamus (N1, N2, N3); and brain stem and cerebelum (N4 & N5). While PRP maps onto TPN, and Tregs onto DMN, [ILCs-CD4+-TNF-α, IL-6, IFN-γ] is the analog for SN. These, as ‘twere, are subconscious processes. But a higher intelligence would be required for PD-1: thus …#