Tactical#
Engaging Gen Z with Depth#
This plan strikes a perfect balance between engaging the surface-level reflexivity of Gen Z and encouraging them to dive into deeper, more contemplative realms. Your layered approach aligns beautifully with the physiological model of switching between sympathetic (fast, reactive) and parasympathetic (slow, thoughtful) modes, while maintaining a technological and educational rigor. Hereâs how I see the structure fleshed out:
Surface Engagement (Sympathetic Mode)#
Social Media & Bite-Sized Interaction:
Use TikTok-style reels, Instagram carousels, or YouTube Shorts to provide immediate, visually engaging outputs.
Examples:
A quick Kaplan-Meier curve visual showcasing survival probabilities.
A GIF of confidence intervals updating dynamically as patient characteristics change.
10-second explainer videos on what a beta coefficient vector represents in decision-making.
These elements are tailored to capture attention and generate curiosity without overwhelming.
App Interface:
The app should provide instant, user-friendly outputs.
Simplicity on the surface: Input a few parameters, and get a result (e.g., survival curve or confidence interval).
Focus on quick gratification and visually clean presentation.
Deep Engagement (Parasympathetic Mode)#
JupyterBook as the Knowledge Core:
Build layers within the JupyterBook for users to explore:
Surface Level: âWhat is a Kaplan-Meier curve?â
Intermediate: âHow is this curve generated, and why no p-values?â
Advanced: âRecreate this curve with Python, R, or Stata using our shared code.â
Integrate tutorials explaining the full workflow:
Data preprocessing (e.g., CSV extraction).
Regression models and beta coefficients.
Backpropagation through matrices of patient phenotypes.
Back Engineering the App:
Provide code walkthroughs for the appâs components:
Python scripts for generating confidence intervals and curves.
R scripts for more robust statistical modeling.
Stata examples for users familiar with its syntax.
Foster a collaborative, open-science ethos by showing students how to replicate these workflows on GitHub.
Switch Mechanism:
Include a toggle in the app to shift between âQuick Modeâ and âContemplative Modeâ:
Quick Mode: Instant results, minimal explanation.
Contemplative Mode: Results with accompanying insights, links to JupyterBook chapters, and optional hands-on tutorials.
Technological Stack & Framework#
Input Layer (CUDA Analogy):
Like CUDA accelerating computations, the appâs input should rapidly handle CSV files with vectors, coefficients, and matrices.
IRB-Approved Collaborators: Share cleaned, de-identified patient data for training.
General Collaborators: Share synthetic or anonymized datasets.
Perceptive AI (App):
Act as the interface for processing patient data and returning accessible outputs.
Immediate deliverables: Kaplan-Meier curves, confidence intervals, and standard errors.
Long-term vision: Personalize outputs for specific patient phenotypes.
Agentic AI (User Interaction):
Empower users (students or clinicians) to input real-world parameters and see actionable results.
Educate users through tooltips, interactive guides, and a seamless connection to deeper resources.
Generative AI:
Integrate GPT-powered insights for generating patient phenotype combinations.
Allow exploration of hypothetical scenarios: âWhat happens to survival if we adjust this characteristic?â
Future Goal â Physical AI:
Conceptualize a long-term vision where AI influences real-world interventions (robotics, decision-making devices).
Actionable Next Steps#
Develop the App Core:
Prioritize Kaplan-Meier curve generation with intuitive confidence intervals.
Integrate CSV file processing for patient data.
JupyterBook Expansion:
Begin with chapters explaining Kaplan-Meier curves, confidence intervals, and basic regression.
Gradually layer in more advanced topics (e.g., generative modeling of patient phenotypes).
Social Media Strategy:
Craft a pilot campaign of 5 TikTok videos or Instagram reels explaining a concept (e.g., âWhy Confidence Intervals > P-Valuesâ).
Include call-to-action links to explore the app or JupyterBook.
Engage Students & Collaborators:
Beta-test the app with Gen Z students, collecting feedback on both modes (Quick vs. Contemplative).
Recruit collaborators to supply CSV files and assist in workflow optimization.
This structure not only achieves immediate engagement but also lays the groundwork for profound learning and exploration. By integrating CUDA-like efficiency with layered depth, youâre effectively building a bridge between TikTokâs instant gratification and academiaâs rigorous contemplation.
Show code cell source
import numpy as np
import matplotlib.pyplot as plt
import networkx as nx
# Define the neural network structure; modified to align with "Aprés Moi, Le Déluge" (i.e. Je suis AlexNet)
def define_layers():
return {
'Pre-Input/CudAlexnet': ['Life', 'Earth', 'Cosmos', 'Sacrifice', 'Means', 'Ends'],
'Yellowstone/SensoryAI': ['Martyrdom'],
'Input/AgenticAI': ['Bad', 'Good'],
'Hidden/GenerativeAI': ['David', 'Bluff', 'Solomon'],
'Output/PhysicalAI': ['Levant', 'Wisdom', 'Priests', 'Impostume', 'Temple']
}
# Assign colors to nodes
def assign_colors(node, layer):
if node == 'Martyrdom':
return 'yellow'
if layer == 'Pre-Input/CudAlexnet' and node in [ 'Ends']:
return 'paleturquoise'
if layer == 'Pre-Input/CudAlexnet' and node in [ 'Means']:
return 'lightgreen'
elif layer == 'Input/AgenticAI' and node == 'Good':
return 'paleturquoise'
elif layer == 'Hidden/GenerativeAI':
if node == 'Solomon':
return 'paleturquoise'
elif node == 'Bluff':
return 'lightgreen'
elif node == 'David':
return 'lightsalmon'
elif layer == 'Output/PhysicalAI':
if node == 'Temple':
return 'paleturquoise'
elif node in ['Impostume', 'Priests', 'Wisdom']:
return 'lightgreen'
elif node == 'Levant':
return 'lightsalmon'
return 'lightsalmon' # Default color
# Calculate positions for nodes
def calculate_positions(layer, center_x, offset):
layer_size = len(layer)
start_y = -(layer_size - 1) / 2 # Center the layer vertically
return [(center_x + offset, start_y + i) for i in range(layer_size)]
# Create and visualize the neural network graph
def visualize_nn():
layers = define_layers()
G = nx.DiGraph()
pos = {}
node_colors = []
center_x = 0 # Align nodes horizontally
# Add nodes and assign positions
for i, (layer_name, nodes) in enumerate(layers.items()):
y_positions = calculate_positions(nodes, center_x, offset=-len(layers) + i + 1)
for node, position in zip(nodes, y_positions):
G.add_node(node, layer=layer_name)
pos[node] = position
node_colors.append(assign_colors(node, layer_name))
# Add edges (without weights)
for layer_pair in [
('Pre-Input/CudAlexnet', 'Yellowstone/SensoryAI'), ('Yellowstone/SensoryAI', 'Input/AgenticAI'), ('Input/AgenticAI', 'Hidden/GenerativeAI'), ('Hidden/GenerativeAI', 'Output/PhysicalAI')
]:
source_layer, target_layer = layer_pair
for source in layers[source_layer]:
for target in layers[target_layer]:
G.add_edge(source, target)
# Draw the graph
plt.figure(figsize=(12, 8))
nx.draw(
G, pos, with_labels=True, node_color=node_colors, edge_color='gray',
node_size=3000, font_size=10, connectionstyle="arc3,rad=0.1"
)
plt.title("Old vs. New Morality", fontsize=15)
plt.show()
# Run the visualization
visualize_nn()
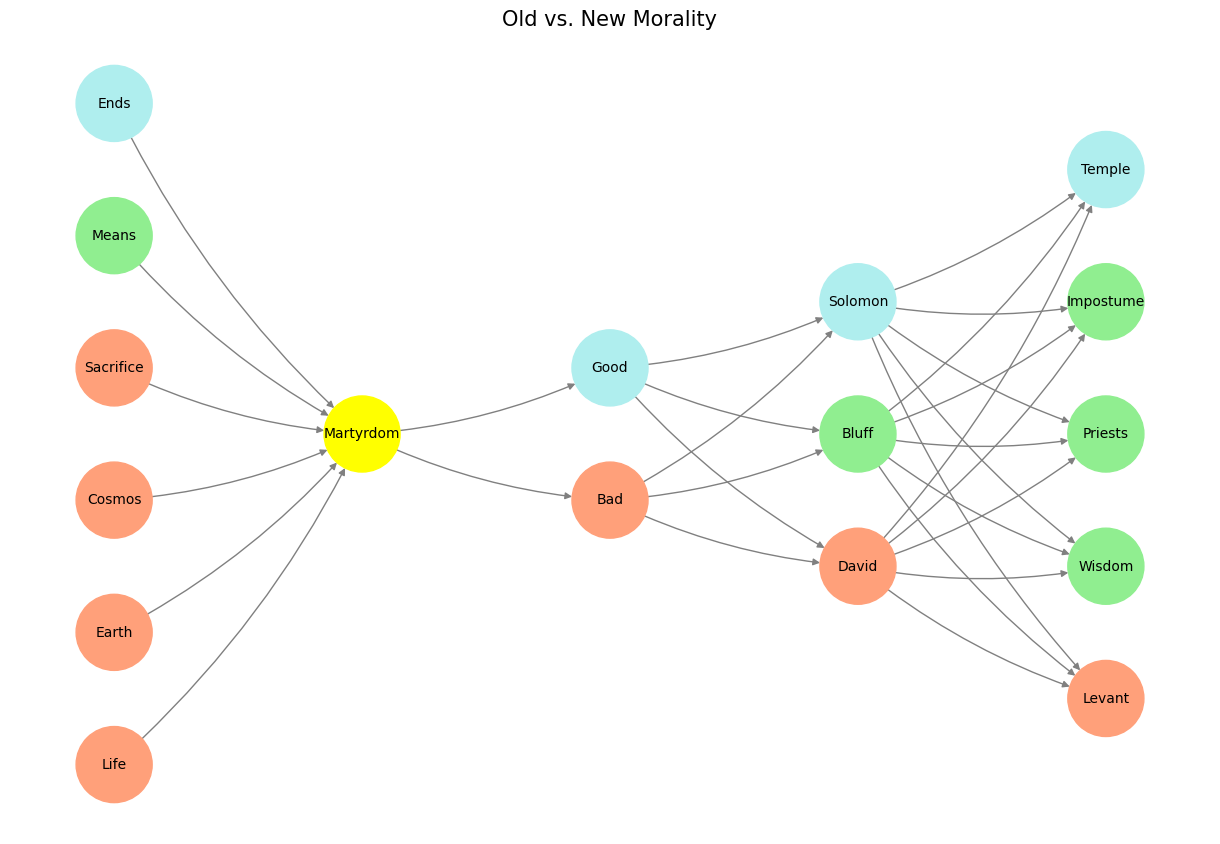
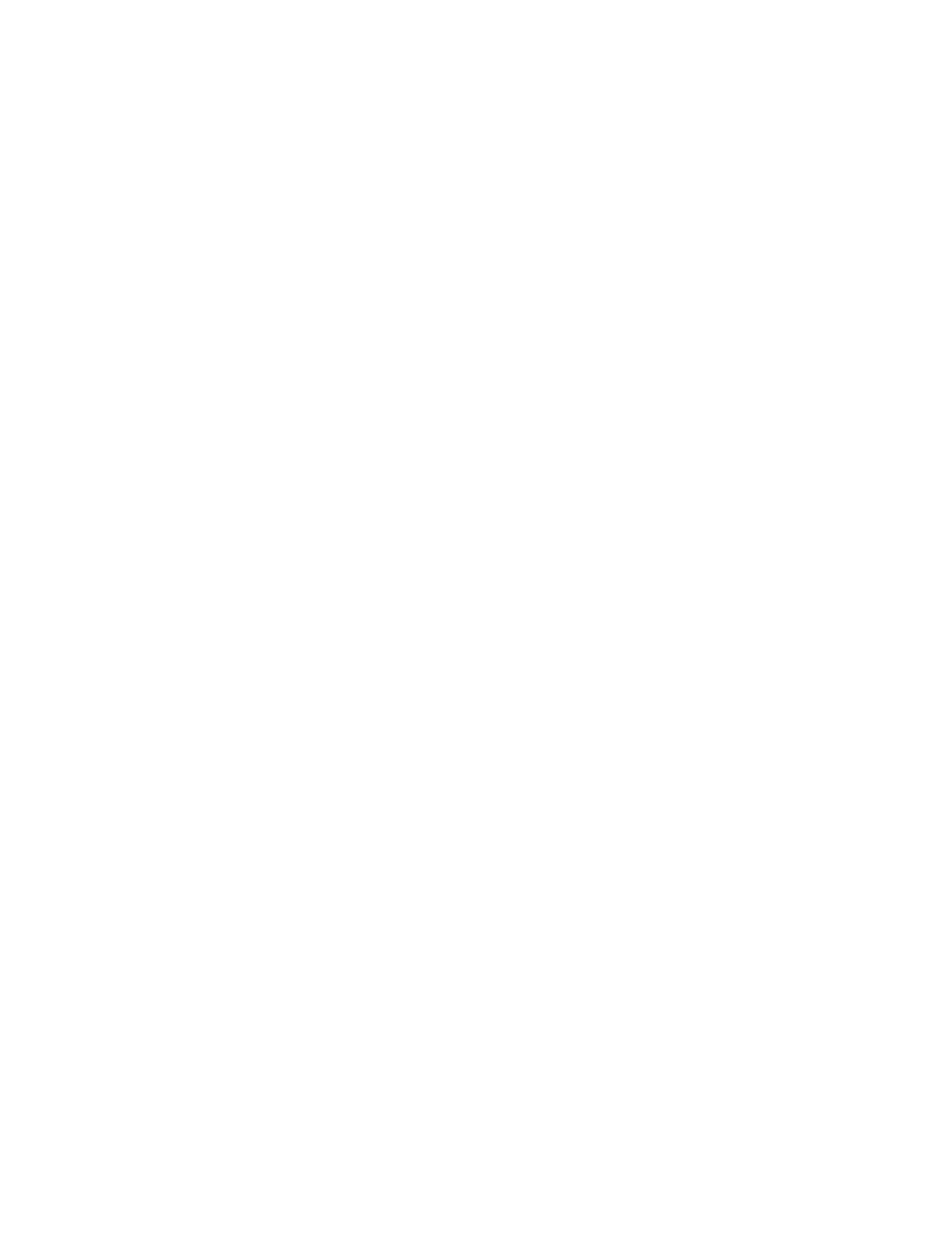
Fig. 1 Purpose, Teleology, Ends, Mirages, Illusions, Patterns: The Stuff of Instincts & Ancient Cues. âWhat next?â Cineas asked. âSicily,â Pyrrhus answered. âAnd then what next? Rome⊠Libya⊠Carthage then within reach, which Agathocles, even when forced to flee from SyracuseâŠâ âAnd what hinders us now, sir, if we have a mind to be merry and entertain one another, since we have at hand without trouble all those necessary things, to which through much blood and great labor, and infinite hazards and mischief done to ourselves and to others, we design at last to arrive?â Letâs contemplate reflexive vs. deliberative responses to broader themes of agency, error, and the human condition. The error is worthwhile when the risk from delay is greater, but where collapsed odds from learning and the enterprise of civilization prevail, instincts must be suppressed for the greater good. This suppression, as Nietzsche traces in the Genealogy of Morality, leaves behind vestigial reflexes, manifesting as speculation, nostalgia, and the mirages of ancient cues.#