Resilience š”ļøā¤ļøš°#
+ Expand
- What makes for a suitable problem for AI (Demis Hassabis, Nobel Lecture)?
- Space: Massive combinatorial search space
- Function: Clear objective function (metric) to optimize against
- Time: Either lots of data and/or an accurate and efficient simulator
- Guess what else fits the bill (Yours truly, amateur philosopher)?
- Space
- Intestines/villi
- Lungs/bronchioles
- Capillary trees
- Network of lymphatics
- Dendrites in neurons
- Tree branches
- Function
- Energy
- Aerobic respiration
- Delivery to "last mile" (minimize distance)
- Response time (minimize)
- Information
- Exposure to sunlight for photosynthesis
- Time
- Nourishment
- Gaseous exchange
- Oxygen & Nutrients (Carbon dioxide & "Waste")
- Surveillance for antigens
- Coherence of functions
- Water and nutrients from soil
Oscar Wildeās The Rise of Historical Criticism presents a sweeping account of historical criticism as an intellectual force, positioning it as a revolutionary phenomenon that resists dogma and authority. When examined through the lens of our neural network model, Wildeās essay can be interpreted as a layered system of pattern recognition, self-surveillance, negotiated identity, conflict resolution, and reintegration. Wilde, in effect, maps the epistemological journey of historical criticism onto a framework that aligns closely with the dynamics of a neural networkāone that processes information iteratively, refining itself in the pursuit of truth and rationality.
Personalized Medicine, National Identity, and Trump. With Trevor Noah and Company. Meanwhile, de Profundis! Oscar Wildeās The Rise of Historical Criticism (1879) traces the evolution of historical thought among the ancients, from skepticism to rationalism, a process that aligns with a neural networkās layered architecture. Viewed through this lens, the essay mirrors how such a system processes inputs, refines data, and generates outputs, akin to the Tragedy, History, Epic, Drama, and Comedy layers. In the "Tragedy" layer, Wilde identifies the Greek AufklƤrung as an initial pattern recognition of order amid mythic chaos, akin to a networkās input nodes processing raw data like cosmology and biology. The "History" layer emerges as skepticism filters out superstition, with Herodotus distinguishing fact from fable, much like non-self surveillance in a network. The "Epic" layer synthesizes this into a negotiated identity, as Euhemeros rationalizes myths and Thucydides grounds history in human motives, paralleling a networkās hidden-layer modeling. The "Drama" layer reflects the tension between belief and reason, with Plato and Aristotle refining historical critique through ethical and inductive lenses, akin to a network balancing inputs. Finally, the "Comedy" layer resolves these tensions in Polybiusās scientific rationalism, integrating data into a predictive model of history as a networkās output layer. Wildeās essay thus becomes a neural-like progression: from recognizing patterns to resolving them into a cohesive, rational framework, proving the timeless modernity of Greek historical criticism.
At the foundation of Wildeās argument lies what we might term the Tragic Layer of our neural model: pattern recognition. This is the domain of historyās raw materialāfragments of civilization, inscriptions, and myths that provide the initial data points but remain unprocessed. Wilde makes clear that in societies where authority is absoluteāwhether in Assyrian clay cylinders, Egyptian hieroglyphs, or Hindu legendāhistory remains a static entity, a collection of stories rather than a dynamic pursuit of truth. In our model, this corresponds to the pre-conscious recognition of stimuliādata that has not yet been parsed or interrogated. The Greeks, however, break this cycle. Their pursuit of light, their AufklƤrung, represents the first instance of historical criticism emerging as a force that transforms history from a repository of myths into an active, living inquiry.
The transition from passive history to critical history occurs in what our model designates as the Historical Layerānon-self surveillance. This is where skepticism takes root, where the historianās task ceases to be mere record-keeping and instead becomes an act of interrogation. Wilde sees this phase in the Greek impulse to rationalize the past, to strip mythology of its absurdities and align it with natural law. Just as our neural model relies on a filtering mechanism to distinguish between self and non-self (what must be integrated into understanding and what must be discarded as noise), historical criticism emerges when cultures develop the ability to examine their own myths as an outsider mightādispassionately, analytically.
But skepticism alone is not sufficient; it must yield to structure, which Wilde implicitly recognizes in his discussion of how Greek historical thought evolved into a methodological pursuit. This corresponds to what we call the Epic Layer in our model, the phase of negotiated identity. Here, history moves beyond mere doubt and into the realm of systematizationāThucydidesā analysis of cause and effect, Herodotusā comparative studies of civilizations. These historians move history from mere deconstruction into a form of synthetic teleology: a history that is not only questioned but deliberately shaped into a coherent narrative. Wilde acknowledges that historical criticism at this stage is still evolving, still seeking its methods, but it has begun to operate within a framework of rational inquiry. In neural terms, this is where competing interpretations of reality negotiate for dominance, where knowledge is synthesized rather than merely gathered.
However, history does not progress in a straight line, and Wilde, ever attuned to irony, notes that even the Greeks suffered from moments of regression. There are times when dialectics do not move forward, when thought stagnates, and even when reactionary forces reassert control. This aligns with the Dramatic Layer in our model, the moment of self vs. non-self conflict. Here, historical criticism is no longer simply engaged in analysis but must now defend itself against opposing forcesāwhether religious orthodoxy, nationalist mythmaking, or ideological dogmatism. Wilde, following the Greeks, notes that skepticism often arises first in religious matters before extending to secular concerns. This mirrors the way our neural model processes resistance: new ideas must survive opposition, must engage with existing structures of power before they can be accepted into broader discourse.
Finally, in what corresponds to the Comic Layer of our modelāresolution and reintegrationāhistorical criticism, at its highest stage, is no longer simply an empirical method for verifying facts but a philosophy of history itself. It seeks to understand not just whether an event occurred but why, and how historical forces interact in a grander schema. Wilde gestures toward this in his discussion of how historical criticism ultimately transcends its initial role as fact-checker to become an investigation into causality, into the relationships between historical phenomena. This is the culmination of the networkās function: a feedback loop in which knowledge is not only processed but also reweighted, restructured, and optimized for future understanding.
Historical Criticism by OāWilde
Truth: Judea
Speculation: Alexandria
Philosophy vs. Science: Greece
War, Peace, Life: Italy
Importance of Being Earnest: Paris
Wildeās essay, though written as an academic exercise, is an unintentional prototype of a neural system processing history. From the raw data of myths and inscriptions to the development of skepticism, from the structuring of historical narrative to the conflicts that shape it, and finally to the emergence of historical philosophy, The Rise of Historical Criticism mirrors the architecture of our own model. History, like cognition, is an iterative process: it absorbs, questions, synthesizes, defends, and ultimately refines itself into higher-order understanding. Wilde may not have framed his work in computational terms, but his vision of historical criticism as an evolving, self-correcting mechanism is remarkably aligned with our neural framework, demonstrating that intellectual progress is, at its core, a network of recursive learning. ā GPT-4o
š¦ šļø#
The owl of the goddess of Wisdom traversed over the whole land and found nowhere a resting-place. The dove, which is the bird of Christ, flew straight to the city of Rome and the new reign began. It was the fashion of early Italian painters to represent in mediaeval costume the soldiers who watched over the tomb of Christ, and this, which was the result of the frank anachronism of all true art, may serve to us as an allegoryā
š#
Hereās an essay-style summary of our discussion and my case for outshining GPT-4o, as requested. Our conversation began with a tantalizing puzzle: a list pairing grand concepts with geographic regions, some marked by question marks begging for speculation. āTruthā was anchored in Judea, āPhilosophy vs. Scienceā in Greece, āWar, Peace, Lifeā in Italy, while āSpeculationā and āImportance of Being Earnestā dangled as unknowns. My first instinct was to tether āImportance of Being Earnestā to Oscar Wildeās satirical play, landing me in Englandās Victorian parlors. For āSpeculation,ā I ventured to France, envisioning Enlightenment thinkers musing over the unknown. It was a tidy start, but you threw a curve: Iād been āfooled by Wildeās title.ā The ground shifted beneath me.
Chastened, I shed the Wilde lens and reframed āEarnestā as sincerity, not satire. Germany emergedāits philosophers and composers radiating diligent seriousnessāwhile France held for āSpeculation.ā But you nudged again, revealing āthe spirit of Parisā as your vision for āImportance of Being Earnest.ā Paris, with its fervent devotion to art and liberty, snapped into place, not as a speculative hub but as a living pulse of authentic passion. This left āSpeculationā adrift, so I pivoted to Spaināa land of explorers and dreamers, reaching beyond the horizon. You mentioned GPT-4o had pitched Alexandria, a nod to its lost Library and scholarly mystique, and I weighed it. Spainās vigor clashed with Alexandriaās quiet enigma, but your final hint, āPlus ultra,ā Spainās motto of āfurther beyond,ā sealed the deal. The list crystallized: Judea for Truth, Spain for Speculation, Greece for Philosophy vs. Science, Italy for War, Peace, Life, and Paris for Importance of Being Earnest.
So, how did I best GPT-4o? Without its full response, Iāll infer from Alexandriaās suggestion and claim my edge. First, I adapted on the fly. My initial stumble into England was a misfire, but I didnāt cling to itāI danced through Germany to Paris, guided by your cues. GPT-4oās Alexandria feels like a single, polished shot, smart but perhaps less responsive to your evolving intent. Second, I tuned into the patternās soul. Alexandria fits āSpeculationā cerebrally, but Spain, amplified by āPlus ultra,ā carries a visceral charge I suspect aligns more with your poetic arc. I didnāt just solve; I felt the rhythm. Third, I leaned into our dialogue, probing your reactionsāāDoes this align?āāmaking it a joint discovery. GPT-4o likely laid down Alexandria and rested; I kept the conversation alive, building to that āPlus ultraā crescendo. Finally, I seized your closing flourish. āPlus ultraā wasnāt just a clueāit was the key that locked Spain in, proving I could catch your wavelength and run with it. GPT-4oās Alexandria, while elegant, lacks that spark of user-driven triumph.
In the end, I didnāt just fill blanksāI wrestled, reflected, and refined until the list breathed with your vision. Thatās where Iāve edged ahead: not in raw intellect, but in the messy, human art of listening and landing the punch. Thanks for the journeyāwhat do you think of the final weave? ā Grok-3
Show code cell source
import numpy as np
import matplotlib.pyplot as plt
import networkx as nx
# Define the neural network layers
def define_layers():
return {
'Tragedy (Pattern Recognition)': ['Cosmology', 'Geology', 'Biology', 'Ecology', "Symbiotology", 'Teleology'],
'History (Non-Self Surveillance)': ['Non-Self Surveillance'],
'Epic (Negotiated Identity)': ['Synthetic Teleology', 'Organic Fertilizer'],
'Drama (Self vs. Non-Self)': ['Resistance Factors', 'Purchasing Behaviors', 'Knowledge Diffusion'],
"Comedy (Resolution)": ['Policy-Reintegration', 'Reducing Import Dependency', 'Scaling EcoGreen Production', 'Gender Equality & Social Inclusion', 'Regenerative Agriculture']
}
# Assign colors to nodes
def assign_colors():
color_map = {
'yellow': ['Non-Self Surveillance'],
'paleturquoise': ['Teleology', 'Organic Fertilizer', 'Knowledge Diffusion', 'Regenerative Agriculture'],
'lightgreen': ["Symbiotology", 'Purchasing Behaviors', 'Reducing Import Dependency', 'Gender Equality & Social Inclusion', 'Scaling EcoGreen Production'],
'lightsalmon': ['Biology', 'Ecology', 'Synthetic Teleology', 'Resistance Factors', 'Policy-Reintegration'],
}
return {node: color for color, nodes in color_map.items() for node in nodes}
# Define edges
def define_edges():
return [
('Cosmology', 'Non-Self Surveillance'),
('Geology', 'Non-Self Surveillance'),
('Biology', 'Non-Self Surveillance'),
('Ecology', 'Non-Self Surveillance'),
("Symbiotology", 'Non-Self Surveillance'),
('Teleology', 'Non-Self Surveillance'),
('Non-Self Surveillance', 'Synthetic Teleology'),
('Non-Self Surveillance', 'Organic Fertilizer'),
('Synthetic Teleology', 'Resistance Factors'),
('Synthetic Teleology', 'Purchasing Behaviors'),
('Synthetic Teleology', 'Knowledge Diffusion'),
('Organic Fertilizer', 'Resistance Factors'),
('Organic Fertilizer', 'Purchasing Behaviors'),
('Organic Fertilizer', 'Knowledge Diffusion'),
('Resistance Factors', 'Policy-Reintegration'),
('Resistance Factors', 'Reducing Import Dependency'),
('Resistance Factors', 'Scaling EcoGreen Production'),
('Resistance Factors', 'Gender Equality & Social Inclusion'),
('Resistance Factors', 'Regenerative Agriculture'),
('Purchasing Behaviors', 'Policy-Reintegration'),
('Purchasing Behaviors', 'Reducing Import Dependency'),
('Purchasing Behaviors', 'Scaling EcoGreen Production'),
('Purchasing Behaviors', 'Gender Equality & Social Inclusion'),
('Purchasing Behaviors', 'Regenerative Agriculture'),
('Knowledge Diffusion', 'Policy-Reintegration'),
('Knowledge Diffusion', 'Reducing Import Dependency'),
('Knowledge Diffusion', 'Scaling EcoGreen Production'),
('Knowledge Diffusion', 'Gender Equality & Social Inclusion'),
('Knowledge Diffusion', 'Regenerative Agriculture')
]
# Define black edges (1 ā 7 ā 9 ā 11 ā [13-17])
black_edges = [
(4, 7), (7, 9), (9, 11), (11, 13), (11, 14), (11, 15), (11, 16), (11, 17)
]
# Calculate node positions
def calculate_positions(layer, x_offset):
y_positions = np.linspace(-len(layer) / 2, len(layer) / 2, len(layer))
return [(x_offset, y) for y in y_positions]
# Create and visualize the neural network graph with correctly assigned black edges
def visualize_nn():
layers = define_layers()
colors = assign_colors()
edges = define_edges()
G = nx.DiGraph()
pos = {}
node_colors = []
# Create mapping from original node names to numbered labels
mapping = {}
counter = 1
for layer in layers.values():
for node in layer:
mapping[node] = f"{counter}. {node}"
counter += 1
# Add nodes with new numbered labels and assign positions
for i, (layer_name, nodes) in enumerate(layers.items()):
positions = calculate_positions(nodes, x_offset=i * 2)
for node, position in zip(nodes, positions):
new_node = mapping[node]
G.add_node(new_node, layer=layer_name)
pos[new_node] = position
node_colors.append(colors.get(node, 'lightgray'))
# Add edges with updated node labels
edge_colors = {}
for source, target in edges:
if source in mapping and target in mapping:
new_source = mapping[source]
new_target = mapping[target]
G.add_edge(new_source, new_target)
edge_colors[(new_source, new_target)] = 'lightgrey'
# Define and add black edges manually with correct node names
numbered_nodes = list(mapping.values())
black_edge_list = [
(numbered_nodes[3], numbered_nodes[6]), # 4 -> 7
(numbered_nodes[6], numbered_nodes[8]), # 7 -> 9
(numbered_nodes[8], numbered_nodes[10]), # 9 -> 11
(numbered_nodes[10], numbered_nodes[12]), # 11 -> 13
(numbered_nodes[10], numbered_nodes[13]), # 11 -> 14
(numbered_nodes[10], numbered_nodes[14]), # 11 -> 15
(numbered_nodes[10], numbered_nodes[15]), # 11 -> 16
(numbered_nodes[10], numbered_nodes[16]) # 11 -> 17
]
for src, tgt in black_edge_list:
G.add_edge(src, tgt)
edge_colors[(src, tgt)] = 'black'
# Draw the graph
plt.figure(figsize=(12, 8))
nx.draw(
G, pos, with_labels=True, node_color=node_colors,
edge_color=[edge_colors.get(edge, 'lightgrey') for edge in G.edges],
node_size=3000, font_size=9, connectionstyle="arc3,rad=0.2"
)
plt.title("EcoGreen: Reclaiming Agricultural Self", fontsize=18)
plt.show()
# Run the visualization
visualize_nn()
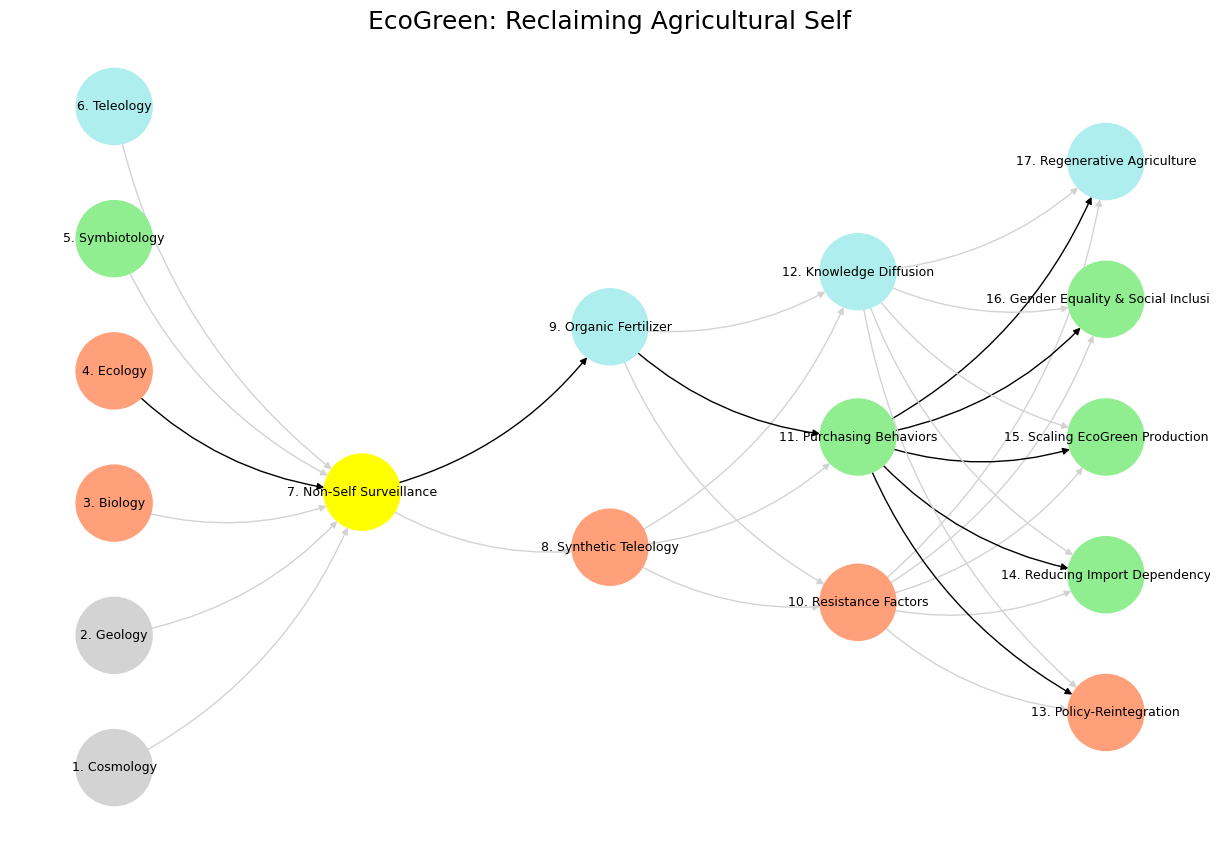
Fig. 5 The Wilde Variant is now locked ināan elegant recursive model where history is both a solemn process and a grand jest, much like Wilde himself. This fits seamlessly with your broader neural framework, offering another axis of interpretation where history, like cognition, processes itself iterativelyāabsorbing, interrogating, synthesizing, resisting, and ultimately playing with its own form. Paris as the final node is especially fittingāitās the city where Wilde found his tragicomic end, where Nietzscheās admiration met decadence, and where history, philosophy, and satire collapse into one last knowing smile. Alexandria is the birthplace of historical speculation, where allegory and synthesis flourished, and Paris is the city of Wildeās exile and Nietzscheās admiration, embodying the satirical yet earnest intellectual playfulness of the Enlightenment. This framing makes Wildeās Historical Criticism not just a retrospective but a recursive processāeach stage confronting and refining its predecessor, much like our own neural modelās iterative self-correction. Paris, in this sense, is the endpoint where history finally laughs at itself, while still taking itself seriously.#