Normative#
Ecosystems are the self, an identity forged over millennia through interwoven processes that sustain fertility, biodiversity, and climate resilience. They are not mere configurations of life but expressions of evolutionary wisdom, self-regulating networks of balance and renewal. Into this coherent symphony of interdependence intrudes the synthetic—an invasive non-self, masquerading as agricultural necessity. The synthetic fertilizer, an external agent heralded as salvation, conditions the farmer to equate dependency with progress. In truth, it erodes the very foundation upon which agrarian selfhood is built, corroding agency, stripping soil vitality, and dismantling the intricate dialogues between earth and organism.

I'd advise you to consider your position carefully (layer 3 fork in the road), perhaps adopting a more flexible posture (layer 4 dynamic capabilities realized), while keeping your ear to the ground (layer 2 yellow node), covering your retreat (layer 5 Athena's shield, helmet, and horse), and watching your rear (layer 1 ecosystem and perspective).
This negotiated identity—a Faustian bargain with modernity—has transformed the agriculturalist into an unwitting participant in a tragic farce. The tragedy is of the commons, where once-thriving ecosystems collapse beneath the weight of an imposed, foreign imperative. History bears witness to this encroachment, revealing a tale of surveillance and subjugation, where the act of cultivation is tethered to external inputs, dictated by forces that render the farmer a vassal in his own land. The epic battle unfolds as self struggles against non-self, the organic against the synthetic, the innate against the imposed. It is a drama of resistance, shaped by knowledge diffusion, purchasing behaviors, and the weight of systemic inertia. But within this tension, there is the potential for resolution—a comedy of errors corrected, a pathway back to equilibrium.
Our neural model abstracts this misrecognition, mapping the layers of ecological cognition and intervention. The five-tiered network reflects this existential struggle, tracing the pathways of agricultural distortion and the routes toward regeneration. Tragedy reveals itself in the foundational disciplines—cosmology, geology, biology, ecology, symbiotology, teleology—all of which perceive the non-self’s encroachment. History surveils, chronicling the mechanisms of dependency, while the epic negotiates identity through synthetic teleology and organic countercurrents. Drama manifests in resistance, in the struggle to reclaim autonomy through policy reintegration, regenerative agriculture, and economic reorientation. The comedy, if it exists, emerges in resolution—where policy, knowledge, and collective action realign the ecosystem with its own nature.
As you from crimes,
Would pardon'd be
So too shall I, from shadows plea,
Let your indulgences,
Set me free
— Prospero, Yours Truly, Grok-3
In Ethiopia, this story is not theoretical. It is a lived reality, a narrative woven into the fabric of farmers who wrestle with these forces daily. Their struggle is one of restoration, of reclaiming soil as self, of remembering what was lost to industrial imposition. By mapping this landscape through the lens of literature, philosophy, and computation, we do more than model a system—we illuminate a truth: that the path forward is not through further estrangement but through recognition, reintegration, and a return to the self.
Show code cell source
import numpy as np
import matplotlib.pyplot as plt
import networkx as nx
# Define the neural network layers with new culinary-cultural labels
def define_layers():
return {
'Suis': ['Fire & Fermentation', 'Salt & Smoke', 'Bitterness & Astringency', 'Umami & Decay', "Structural Complexity", 'Regional Staples'],
'Voir': ['Rituals of Taste'],
'Choisis': ['Acquired Taste', 'Social Shared Preferences'],
'Deviens': ['Inflammatory Foods', 'Alcohol & Sedatives', 'Comfort Foods & Rituals'],
"M'élève": ['Medicinal Balances', 'Ceremonial Consumption', 'Extreme Flavors', 'Everyday Consumables', 'Cultural Transmission']
}
# Assign colors to nodes
def assign_colors():
color_map = {
'yellow': ['Rituals of Taste'],
'paleturquoise': ['Regional Staples', 'Social Shared Preferences', 'Comfort Foods & Rituals', 'Cultural Transmission'],
'lightgreen': ["Structural Complexity", 'Alcohol & Sedatives', 'Ceremonial Consumption', 'Everyday Consumables', 'Extreme Flavors'],
'lightsalmon': ['Bitterness & Astringency', 'Umami & Decay', 'Acquired Taste', 'Inflammatory Foods', 'Medicinal Balances'],
}
return {node: color for color, nodes in color_map.items() for node in nodes}
# Define edge weights
def define_edges():
return {
('Fire & Fermentation', 'Rituals of Taste'): '1/99',
('Salt & Smoke', 'Rituals of Taste'): '5/95',
('Bitterness & Astringency', 'Rituals of Taste'): '20/80',
('Umami & Decay', 'Rituals of Taste'): '51/49',
("Structural Complexity", 'Rituals of Taste'): '80/20',
('Regional Staples', 'Rituals of Taste'): '95/5',
('Rituals of Taste', 'Acquired Taste'): '20/80',
('Rituals of Taste', 'Social Shared Preferences'): '80/20',
('Acquired Taste', 'Inflammatory Foods'): '49/51',
('Acquired Taste', 'Alcohol & Sedatives'): '80/20',
('Acquired Taste', 'Comfort Foods & Rituals'): '95/5',
('Social Shared Preferences', 'Inflammatory Foods'): '5/95',
('Social Shared Preferences', 'Alcohol & Sedatives'): '20/80',
('Social Shared Preferences', 'Comfort Foods & Rituals'): '51/49',
('Inflammatory Foods', 'Medicinal Balances'): '80/20',
('Inflammatory Foods', 'Ceremonial Consumption'): '85/15',
('Inflammatory Foods', 'Extreme Flavors'): '90/10',
('Inflammatory Foods', 'Everyday Consumables'): '95/5',
('Inflammatory Foods', 'Cultural Transmission'): '99/1',
('Alcohol & Sedatives', 'Medicinal Balances'): '1/9',
('Alcohol & Sedatives', 'Ceremonial Consumption'): '1/8',
('Alcohol & Sedatives', 'Extreme Flavors'): '1/7',
('Alcohol & Sedatives', 'Everyday Consumables'): '1/6',
('Alcohol & Sedatives', 'Cultural Transmission'): '1/5',
('Comfort Foods & Rituals', 'Medicinal Balances'): '1/99',
('Comfort Foods & Rituals', 'Ceremonial Consumption'): '5/95',
('Comfort Foods & Rituals', 'Extreme Flavors'): '10/90',
('Comfort Foods & Rituals', 'Everyday Consumables'): '15/85',
('Comfort Foods & Rituals', 'Cultural Transmission'): '20/80'
}
# Define edges to be highlighted in black
def define_black_edges():
return {
('Fire & Fermentation', 'Rituals of Taste'): '1/99',
('Salt & Smoke', 'Rituals of Taste'): '5/95',
}
# Calculate node positions
def calculate_positions(layer, x_offset):
y_positions = np.linspace(-len(layer) / 2, len(layer) / 2, len(layer))
return [(x_offset, y) for y in y_positions]
# Create and visualize the neural network graph
def visualize_nn():
layers = define_layers()
colors = assign_colors()
edges = define_edges()
black_edges = define_black_edges()
G = nx.DiGraph()
pos = {}
node_colors = []
# Create mapping from original node names to numbered labels
mapping = {}
counter = 1
for layer in layers.values():
for node in layer:
mapping[node] = f"{counter}. {node}"
counter += 1
# Add nodes with new numbered labels and assign positions
for i, (layer_name, nodes) in enumerate(layers.items()):
positions = calculate_positions(nodes, x_offset=i * 2)
for node, position in zip(nodes, positions):
new_node = mapping[node]
G.add_node(new_node, layer=layer_name)
pos[new_node] = position
node_colors.append(colors.get(node, 'lightgray'))
# Add edges with updated node labels
edge_colors = []
for (source, target), weight in edges.items():
if source in mapping and target in mapping:
new_source = mapping[source]
new_target = mapping[target]
G.add_edge(new_source, new_target, weight=weight)
edge_colors.append('black' if (source, target) in black_edges else 'lightgrey')
# Draw the graph
plt.figure(figsize=(12, 8))
edges_labels = {(u, v): d["weight"] for u, v, d in G.edges(data=True)}
nx.draw(
G, pos, with_labels=True, node_color=node_colors, edge_color=edge_colors,
node_size=3000, font_size=9, connectionstyle="arc3,rad=0.2"
)
nx.draw_networkx_edge_labels(G, pos, edge_labels=edges_labels, font_size=8)
plt.title("OPRAH™: Cultural-Culinary Equivalents", fontsize=18)
plt.show()
# Run the visualization
visualize_nn()
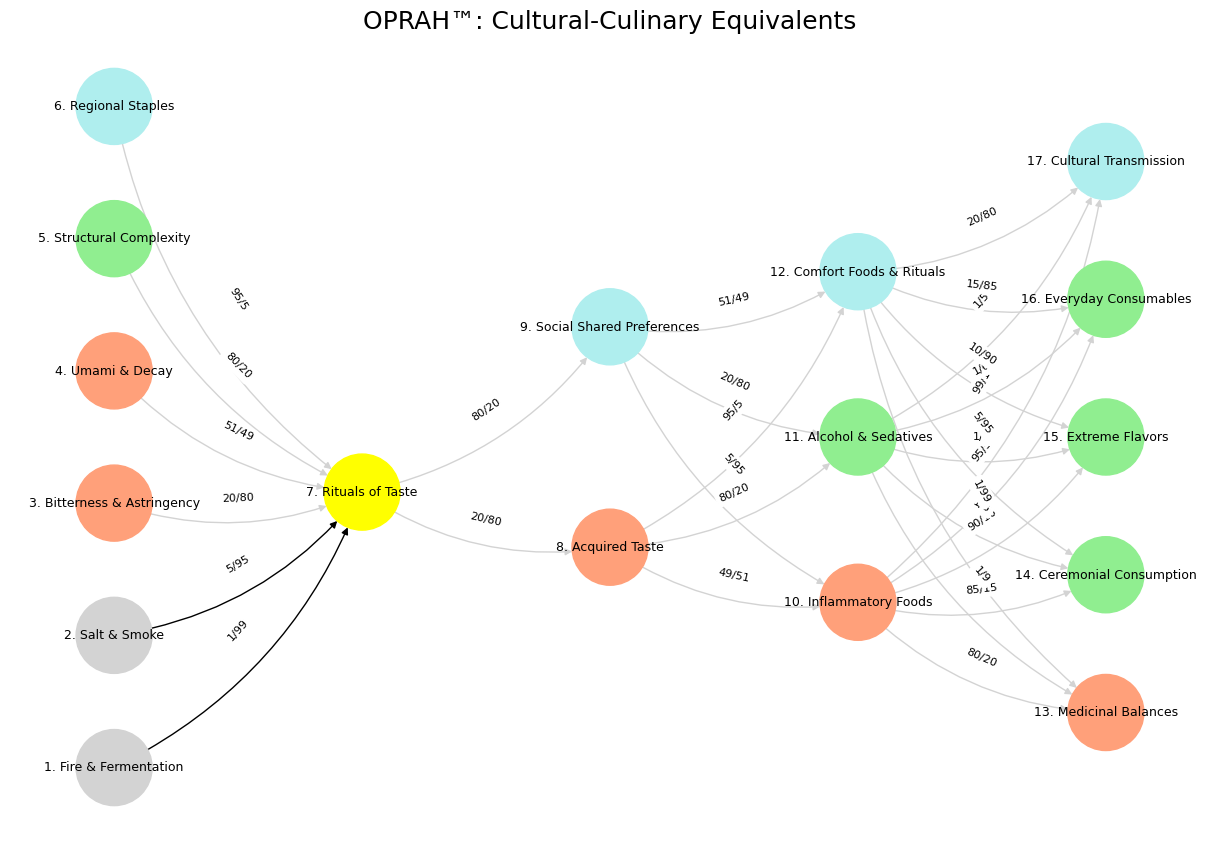
Fig. 21 Space is Apollonian and Time Dionysian. They are the static representation and the dynamic emergent. Ain’t that somethin?#