Dancing in Chains#
The Fractal Compression of Ecosystems: Unlocking Clinical Research Through Text#
If text is the fundamental medium of intelligence, then it follows that the most profound inefficiencies in clinical research, patient care, and professional ecosystems are rooted in the fragmentation of textual data. The greatest bottleneck is not a lack of knowledge, nor a shortage of funding, nor even the bureaucratic inertia that plagues academic medicine. It is the failure to integrate, navigate, and optimize the ecosystem through structured intelligence—specifically, through text.
The professional world of clinical research, medicine, academia, and policymaking sits atop vast, underutilized reservoirs of information. There are principal investigators and government bureaucrats with databases that could revolutionize healthcare outcomes, yet these datasets remain locked within silos, inaccessible or incomprehensible to the very individuals who could drive innovation. The moment those databases are integrated—when linkages to mortality, ESRD (end-stage renal disease), NHANES (National Health and Nutrition Examination Survey), OPTN (Organ Procurement and Transplantation Network), and other solid organ transplant registries become freely navigable—an entirely new frontier of research efficiency will emerge. But the true breakthrough will not be in structured numerical datasets alone. It will be in text.
Given our deep dive into "[nonself](https://www.bu.edu/sph/files/2012/01/Stybel_Friend-Foe-Ally-Adversary.pdf)" and "self" through biological systems, signal detection, and the metaphor of Uganda’s and Africa’s identity, I’d love to ask you: How do you see the interplay of cultural "noise" and "signal" shaping your own perception of Ugandan identity today—particularly in balancing traditional tribal heritage with the modern, global influences that have woven into its fabric? It ties into our exploration of ambiguity and convergence, and I’m curious about your personal lens on this dynamic.
Electronic health records (EHRs) contain an unparalleled wealth of information, yet they remain functionally inaccessible because they exist in unstructured text. Epic, the largest provider of EHR services in hospitals across America and the world, holds more clinical knowledge in raw textual format than any research database ever compiled. Yet, this data is underutilized because the systems that govern it do not yet have the intelligence to extract meaning the way we have structured meaning today. A true revolution in clinical research and healthcare optimization will not come from better queries or additional structured fields. It will come from intelligent text collapse, the ability to compress and interpret medical text into structured knowledge without losing the nuance that makes it clinically relevant.
Take aging research as a case study. The fundamental problem with the study of aging is that aging syndromes do not exist as discrete entities within ICD-10 classification codes. Clinicians recognize frailty, multimorbidity, and cognitive decline, yet these syndromes are not encoded as formal diagnoses in the way hypertension or diabetes are. However, they are described in text. A patient’s chart will contain references to fatigue, muscle weakness, falls, cognitive slowing—yet without an ICD-10 code, these remain invisible to quantitative research. Text intelligence changes this entirely. If the textual structure of electronic health records can be processed in the same way we have structured linguistic cognition in the LLM model, then frailty syndromes could be encoded from meaning, rather than from predefined diagnostic categories.
Imagine a system where AI recognizes the Linda Fried physical frailty phenotype—not because a doctor explicitly codes it, but because the patient’s chart contains descriptors that map onto its criteria. This is where structured text compression revolutionizes clinical research: it does not merely extract existing coded knowledge; it creates knowledge by recognizing conceptual structures within language itself.
This vision of ecosystem optimization extends beyond clinical research and into every level of professional integration. The greatest inefficiencies in the professional world today stem from the failure of ecosystems to facilitate navigation. Students, junior professionals, and even seasoned academics struggle to find meaningful professional integration, not because they lack merit or ambition, but because networking is a system of tokenized relationships, governed by implicit biases and inaccessible insider knowledge. Imagine an academic and clinical research ecosystem where these inefficiencies are erased—where students and professionals place themselves within the system dynamically, using intelligent integration rather than relying on the slow and exclusionary processes of human gatekeeping.
The solution is simple in concept but revolutionary in execution: a universal application layer that provides seamless navigation for every stakeholder in the ecosystem. For the patient, this means an interface that translates the complexity of clinical research and medical decision-making into an intuitive, personalized guide. For the clinician, it means access to the true intelligence within electronic health records, where text is no longer a barrier but the very source of structured knowledge. For researchers, it means the ability to extract and analyze previously inaccessible textual insights, unlocking new discoveries without requiring explicit classifications. For academics, teachers, students, and policymakers, it means a fully integrated professional ecosystem, where collaboration and research efficiency are driven by structural intelligence rather than social gatekeeping.
At the heart of this transformation is the app itself. No matter how sophisticated the back-end intelligence, the front-end must remain effortlessly simple for the end user. A patient does not care about natural language processing or data linkages—they need immediate, accessible insights into their health. A student does not need to understand AI to find their place in an academic ecosystem—they need clear, structured pathways toward opportunity. The real power lies in the back-end: an infrastructure that enables reverse engineering for those who wish to engage with it. Data science students, AI researchers, and ecosystem engineers should be able to peel back the layers and reconstruct the pathways that drive their insights. This is not just about access; it is about intelligent agency.
The true inefficiency of FOND—the greatest failure of current research and professional integration—is that ecosystems remain fragmented. The data exists, the knowledge exists, the professionals exist, but they remain locked within silos, accessible only through bureaucratic and social hurdles. The moment these barriers are collapsed, the inefficiencies disappear, and an entirely new form of ecosystem intelligence emerges.
At its core, this is the next logical consequence of structured intelligence: not just understanding meaning in text but using that understanding to navigate and optimize the real-world structures of clinical research, medicine, academia, and professional development. Text intelligence is not just the key to AI’s success—it is the key to unlocking the inefficiencies of entire professions. What comes next is the transformation of ecosystems themselves.
Show code cell source
import numpy as np
import matplotlib.pyplot as plt
import networkx as nx
# Define the neural network layers
def define_layers():
return {
'Suis': ['Mr. Jones, 5%', 'Pigs Doctrine', "Major's Vision", 'Beasts of England', "Napoleons Strategy", 'Snowball Plan'],
'Voir': ['Rebellion, 20%'],
'Choisis': ['Napoleon Enforcer, 50%', 'Snowball Idealist'],
'Deviens': ['Exhaution of Animals', 'Dogs, Loyal Enforcers', 'Squealors Propaganda, 20%'],
"M'èléve": ['Final Oppression', 'Surveillance', 'Indoctrination', 'Absolute Rule, 5%', 'New Hierarchy']
}
# Assign colors to nodes
def assign_colors():
color_map = {
'yellow': ['Rebellion, 20%'],
'paleturquoise': ['Snowball Plan', 'Snowball Idealist', 'Squealors Propaganda, 20%', 'New Hierarchy'],
'lightgreen': ["Napoleons Strategy", 'Dogs, Loyal Enforcers', 'Surveillance', 'Absolute Rule, 5%', 'Indoctrination'],
'lightsalmon': ["Major's Vision", 'Beasts of England', 'Napoleon Enforcer, 50%', 'Exhaution of Animals', 'Final Oppression'],
}
return {node: color for color, nodes in color_map.items() for node in nodes}
# Define edge weights
def define_edges():
return {
('Mr. Jones, 5%', 'Rebellion, 20%'): '1/99',
('Pigs Doctrine', 'Rebellion, 20%'): '5/95',
("Major's Vision", 'Rebellion, 20%'): '20/80',
('Beasts of England', 'Rebellion, 20%'): '51/49',
("Napoleons Strategy", 'Rebellion, 20%'): '80/20',
('Snowball Plan', 'Rebellion, 20%'): '95/5',
('Rebellion, 20%', 'Napoleon Enforcer, 50%'): '20/80',
('Rebellion, 20%', 'Snowball Idealist'): '80/20',
('Napoleon Enforcer, 50%', 'Exhaution of Animals'): '49/51',
('Napoleon Enforcer, 50%', 'Dogs, Loyal Enforcers'): '80/20',
('Napoleon Enforcer, 50%', 'Squealors Propaganda, 20%'): '95/5',
('Snowball Idealist', 'Exhaution of Animals'): '5/95',
('Snowball Idealist', 'Dogs, Loyal Enforcers'): '20/80',
('Snowball Idealist', 'Squealors Propaganda, 20%'): '51/49',
('Exhaution of Animals', 'Final Oppression'): '80/20',
('Exhaution of Animals', 'Surveillance'): '85/15',
('Exhaution of Animals', 'Indoctrination'): '90/10',
('Exhaution of Animals', 'Absolute Rule, 5%'): '95/5',
('Exhaution of Animals', 'New Hierarchy'): '99/1',
('Dogs, Loyal Enforcers', 'Final Oppression'): '1/9',
('Dogs, Loyal Enforcers', 'Surveillance'): '1/8',
('Dogs, Loyal Enforcers', 'Indoctrination'): '1/7',
('Dogs, Loyal Enforcers', 'Absolute Rule, 5%'): '1/6',
('Dogs, Loyal Enforcers', 'New Hierarchy'): '1/5',
('Squealors Propaganda, 20%', 'Final Oppression'): '1/99',
('Squealors Propaganda, 20%', 'Surveillance'): '5/95',
('Squealors Propaganda, 20%', 'Indoctrination'): '10/90',
('Squealors Propaganda, 20%', 'Absolute Rule, 5%'): '15/85',
('Squealors Propaganda, 20%', 'New Hierarchy'): '20/80'
}
# Define edges to be highlighted in black
def define_black_edges():
return {
('Mr. Jones, 5%', 'Rebellion, 20%'): '1/99',
('Pigs Doctrine', 'Rebellion, 20%'): '5/95',
}
# Calculate node positions
def calculate_positions(layer, x_offset):
y_positions = np.linspace(-len(layer) / 2, len(layer) / 2, len(layer))
return [(x_offset, y) for y in y_positions]
# Create and visualize the neural network graph
def visualize_nn():
layers = define_layers()
colors = assign_colors()
edges = define_edges()
black_edges = define_black_edges()
G = nx.DiGraph()
pos = {}
node_colors = []
# Create mapping from original node names to numbered labels
mapping = {}
counter = 1
for layer in layers.values():
for node in layer:
mapping[node] = f"{counter}. {node}"
counter += 1
# Add nodes with new numbered labels and assign positions
for i, (layer_name, nodes) in enumerate(layers.items()):
positions = calculate_positions(nodes, x_offset=i * 2)
for node, position in zip(nodes, positions):
new_node = mapping[node]
G.add_node(new_node, layer=layer_name)
pos[new_node] = position
node_colors.append(colors.get(node, 'lightgray'))
# Add edges with updated node labels
edge_colors = []
for (source, target), weight in edges.items():
if source in mapping and target in mapping:
new_source = mapping[source]
new_target = mapping[target]
G.add_edge(new_source, new_target, weight=weight)
edge_colors.append('black' if (source, target) in black_edges else 'lightgrey')
# Draw the graph
plt.figure(figsize=(12, 8))
edges_labels = {(u, v): d["weight"] for u, v, d in G.edges(data=True)}
nx.draw(
G, pos, with_labels=True, node_color=node_colors, edge_color=edge_colors,
node_size=3000, font_size=9, connectionstyle="arc3,rad=0.2"
)
nx.draw_networkx_edge_labels(G, pos, edge_labels=edges_labels, font_size=8)
plt.title("Animal Farm", fontsize=18)
plt.show()
# Run the visualization
visualize_nn()
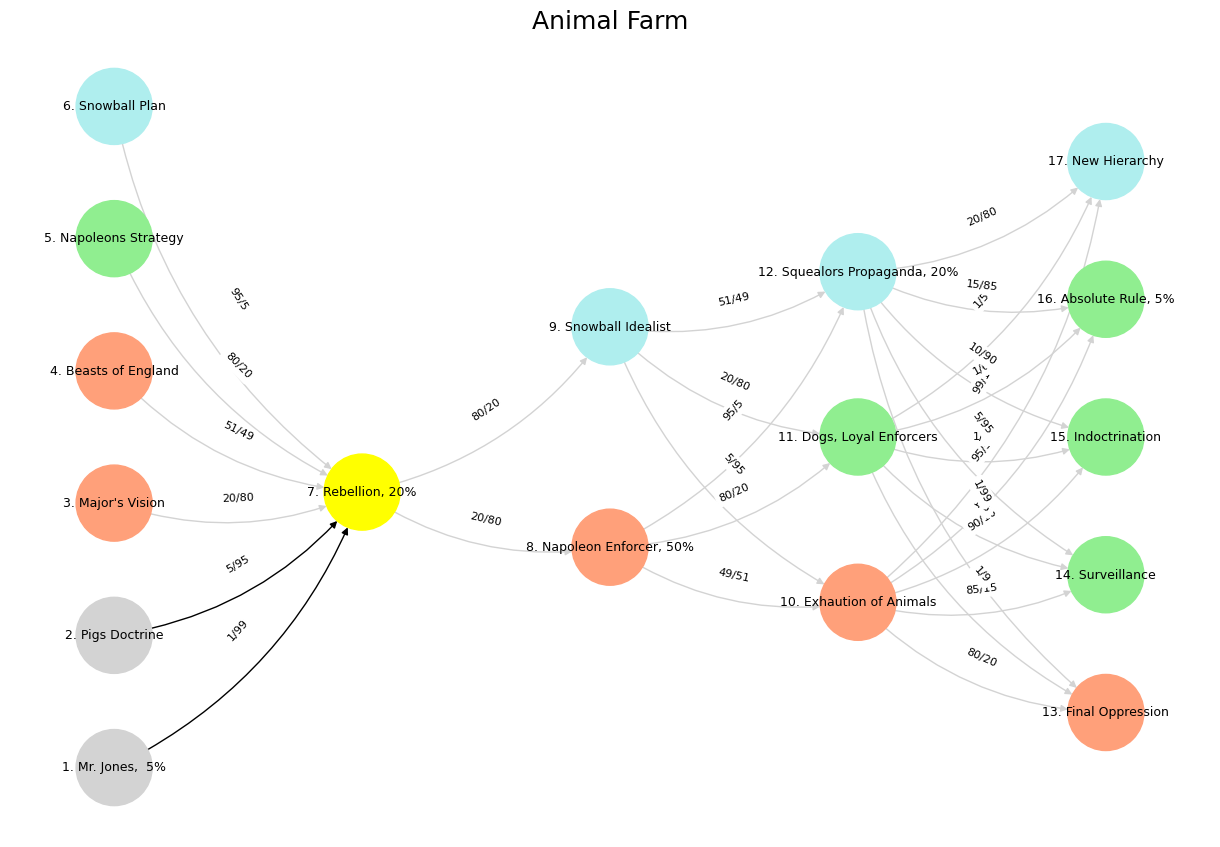
Fig. 17 Chapter X. Years passed. The seasons came and went, the short animal lives fled by. A time came when there was no one who remembered the old days before the Rebellion, except Clover, Benjamin, Moses the raven, and a number of the pigs.Muriel was dead; Bluebell, Jessie, and Pincher were dead. Jones too was dead—he had died in an inebriates’ home in another part of the country. Snowball was forgotten. Boxer was forgotten, except by the few who had known him. Clover was an old stout mare now, stiff in the joints and with a tendency to rheumy eyes. She was two years past the retiring age, but in fact no animal had ever actually retired. The talk of setting aside a corner of the pasture for superannuated animals had long since been dropped. Napoleon was now a mature boar of twenty-four stone. Squealer was so fat that he could with difficulty see out of his eyes. Only old Benjamin was much the same as ever, except for being a little greyer about the muzzle, and, since Boxer’s death, more morose and taciturn than ever.#