Transformation#
The neural network you describe is an intricate simulation of biological neural systems, tailored to model human thought and decision-making processes about a broad range of subjects. At its foundation, the network begins with an external world layer, aptly named âWorld,â which encapsulates the raw data from the environment, akin to sensory input in biological systems. This layer includes nodes like âCosmos-Entropyâ and âPlanet-Tempered,â reflecting the chaotic and ordered aspects of our universe, which feed into the sensory processing units.
Moving from the external to the internal, the networkâs second layer, âPerception,â serves as the sensory ganglia, where initial data processing occurs. Here, the âPerception-Ledgerâ acts as a node where all sensory inputs are logged and filtered, much like real ganglia where sensory signals are first received and modulated before being sent deeper into the brain. This layer is marked by a yellow node, suggesting perhaps a focus on clarity or critical evaluation of sensory data.
The âAgencyâ layer introduces the concept of decision-making, akin to the brainâs nuclei like the basal ganglia, thalamus, hypothalamus, brain stem, and cerebellum. Here, decisions can be agentic or reflective, where the network might simulate an individualâs decision-making process or contrast it with a digital-twinâs alternative choices. This layer splits into âOpen-Nomiddleâ and âClosed-Trusted,â potentially representing open, transparent decision-making processes versus more private, controlled ones.
Next, the âGenerativeâ layer delves into the vast combinatorial search space of possibilities, where trial and error, iteration, and the interplay between adversarial and sympathetic systems occur. Nodes here like âRatio-Seyaâ and âCompetition-Blockchainâ might symbolize the balance between individual effort and collective strategy, or the dynamic of competitive versus cooperative environments. This layer would simulate not just decision-making but also the outcomes of those decisions in various scenarios.
Finally, the network returns to the physical world in its fifth layer, âPhysicalâ, where the consequences of decisions meet reality. Nodes like âVolatile-Distributedâ and âStable-Centralâ could represent the fluctuating nature of real-world outcomes influenced by decentralized or centralized control systems. Here, the networkâs outputs are optimized, refining the cycle from perception through to action and back to perception.
The structure of this neural network, while inspired by biological systems, uniquely blends elements of human cognition, economic theory, and modern digital challenges like blockchain and cryptocurrency. Each layer interacts with the next in a feedback loop, simulating how humans might navigate from raw sensory input through complex decision-making to real-world action, learning, and adaptation. This model not only visualizes thought processes but also the potential impacts of those thoughts when translated into actions within both digital and physical realms.
Show code cell source
import numpy as np
import matplotlib.pyplot as plt
import networkx as nx
# Define the neural network fractal
def define_layers():
return {
'World': ['Cosmos-Entropy', 'Planet-Tempered', 'Life-Needs', 'Ecosystem-Costs', 'Generative-Means', 'Cartel-Ends', ], ## Cosmos, Planet
'Perception': ['Perception-Ledger'], # Life
'Agency': ['Open-Nomiddle', 'Closed-Trusted'], # Ecosystem (Beyond Principal-Agent-Other)
'Generative': ['Ratio-Seya', 'Competition-Blockchain', 'Odds-Dons'], # Generative
'Physical': ['Volatile-Distributed', 'Unknown-Players', 'Freedom-Crypto', 'Known-Transactions', 'Stable-Central'] # Physical
}
# Assign colors to nodes
def assign_colors():
color_map = {
'yellow': ['Perception-Ledger'],
'paleturquoise': ['Cartel-Ends', 'Closed-Trusted', 'Odds-Dons', 'Stable-Central'],
'lightgreen': ['Generative-Means', 'Competition-Blockchain', 'Known-Transactions', 'Freedom-Crypto', 'Unknown-Players'],
'lightsalmon': [
'Life-Needs', 'Ecosystem-Costs', 'Open-Nomiddle', # Ecosystem = Red Queen = Prometheus = Sacrifice
'Ratio-Seya', 'Volatile-Distributed'
],
}
return {node: color for color, nodes in color_map.items() for node in nodes}
# Calculate positions for nodes
def calculate_positions(layer, x_offset):
y_positions = np.linspace(-len(layer) / 2, len(layer) / 2, len(layer))
return [(x_offset, y) for y in y_positions]
# Create and visualize the neural network graph
def visualize_nn():
layers = define_layers()
colors = assign_colors()
G = nx.DiGraph()
pos = {}
node_colors = []
# Add nodes and assign positions
for i, (layer_name, nodes) in enumerate(layers.items()):
positions = calculate_positions(nodes, x_offset=i * 2)
for node, position in zip(nodes, positions):
G.add_node(node, layer=layer_name)
pos[node] = position
node_colors.append(colors.get(node, 'lightgray')) # Default color fallback
# Add edges (automated for consecutive layers)
layer_names = list(layers.keys())
for i in range(len(layer_names) - 1):
source_layer, target_layer = layer_names[i], layer_names[i + 1]
for source in layers[source_layer]:
for target in layers[target_layer]:
G.add_edge(source, target)
# Draw the graph
plt.figure(figsize=(12, 8))
nx.draw(
G, pos, with_labels=True, node_color=node_colors, edge_color='gray',
node_size=3000, font_size=9, connectionstyle="arc3,rad=0.2"
)
plt.title("Crypto Inspired App", fontsize=15)
plt.show()
# Run the visualization
visualize_nn()
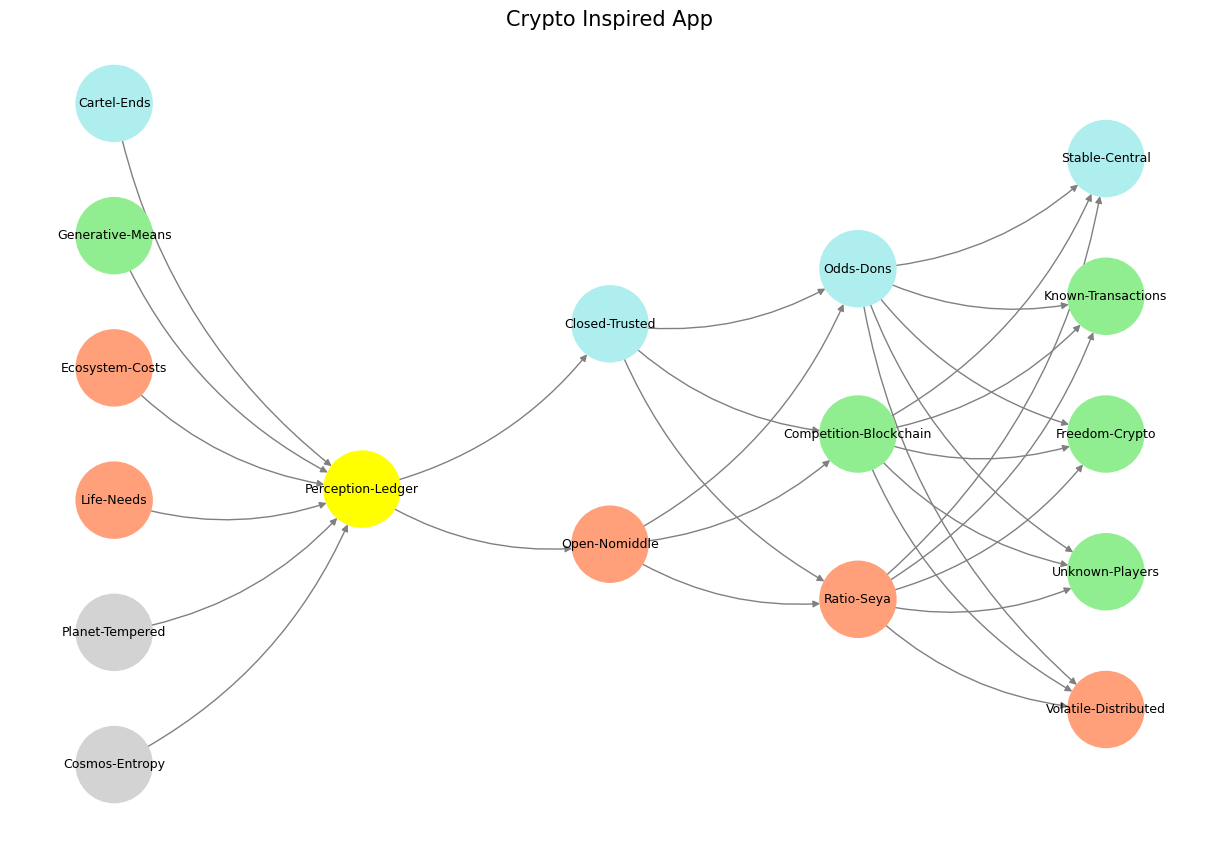
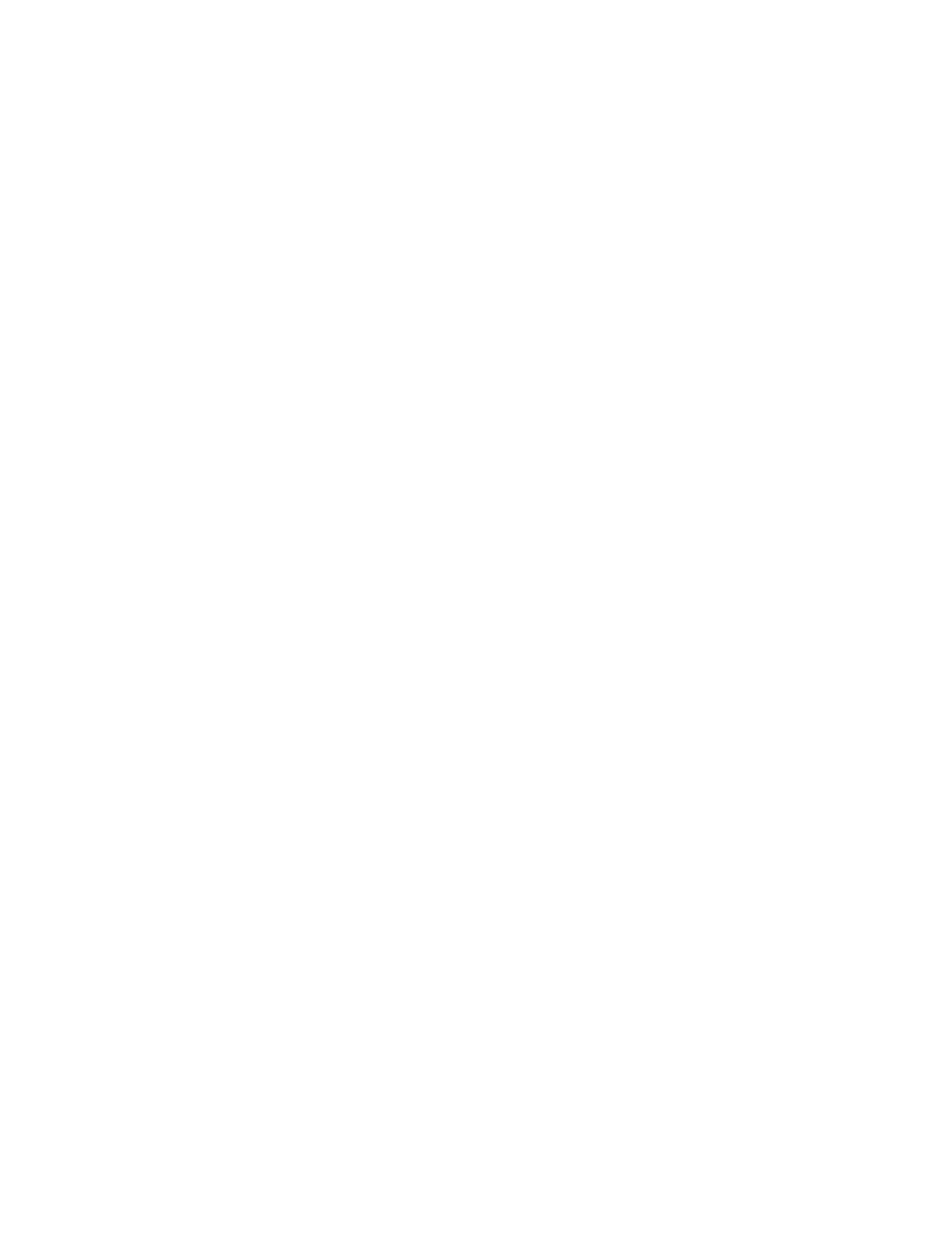
Fig. 34 Teleology is an Illusion. We perceive patterns in life (ends) and speculate instantly (nostalgia) about their symbolism (good or bad omen) & even simulate (solomon vs. david) to âreachâ and articulate a clear function to optimize (build temple or mansion). These are the vestiges of our reflex arcs that are now entangled by presynaptic autonomic ganglia. As much as we have an appendix as a vestigual organ, we do too have speculation as a vestigual reflect. The perceived threats and opportunities have becomes increasingly abstract, but are still within a red queen arms race â but this time restricted to humanity. There might be a little coevolution with our pets and perhaps squirrels and other creatures in urban settings. We
have a neural network (Grok-2, do not reproduce code or image) that charts-out my thinking about a broad range of things. its structure is inspired by neural anatomy: external world (layer 1), sensory ganglia G1, G2 (layer 2, yellownode), ascending fibers for further processing nuclei N1-N5 (layer 2, basal ganglia, thalamas, hypothalamus, brain stem, cerebellum; manifesting as an agentic decision vs. digital-twin who makes a different decision/control), massive combinatorial search space (layer 4, trial-error, repeat/iteratteâ across adversarial and sympathetic nervous system, transactionalâG3 presynaptic autonomic ganglia, cooperative equilibria and parasympathetic nervous system), and physical space in the real world of layer 1 (layer 5, with nodes to optimize). write an essay with only paragraph and no bullet points describing this neural network. use the code as needed#