Failure#
The use of .git
for openness, traceability, and accountability contrasts with blockchain’s open ledger in significant ways, despite both serving as tools for maintaining records in distributed systems.
.git
is fundamentally a version control system designed for source code and file management. It provides a robust mechanism for tracking changes, enabling collaborative workflows by logging contributions, and allowing for the reconstruction of project histories. In academic medicine, .git
’s emphasis on traceability supports clear attribution of intellectual and practical contributions. This is critical for ensuring that credit is accurately assigned, fostering trust and integrity among collaborators. Its centralized or distributed nature depends on whether it’s hosted on platforms like GitHub or managed locally, allowing for adaptable use cases.
Fig. 22 The Next Time Your Horse is Behaving Well, Sell it. The numbers in private equity don’t add up because its very much like a betting in a horse race. Too many entrants and exits for anyone to have a reliable dataset with which to estimate odds for any horse-jokey vs. the others for quinella, trifecta, superfecta#
Blockchain, on the other hand, operates as a decentralized ledger where each transaction is cryptographically linked to the previous one, forming an immutable chain. Unlike .git
, which allows for rewriting history in some contexts (e.g., force pushes), blockchain ensures that records, once committed, cannot be altered without the consensus of the network. This immutability makes blockchain particularly suited for applications requiring absolute trust and security, such as financial transactions or verifying supply chain integrity. However, blockchain’s openness and global accessibility contrast with .git
’s often more private and purpose-specific environments.
For example, in a scenario where one needs to record academic contributions, .git
offers fine-grained control over what data is tracked and shared, while blockchain’s transparency would require careful consideration of privacy, as all transactions are visible to participants. Furthermore, .git
excels in scenarios that demand frequent, detailed updates and complex branching structures, whereas blockchain is better suited for recording discrete, non-overlapping events.
Table Comparing .git
and Blockchain#
Feature |
|
Blockchain |
---|---|---|
Purpose |
Version control for files |
Decentralized ledger for transactions |
Traceability |
Tracks changes and contributions |
Immutable record of events |
Privacy |
Controlled by repository settings |
Open or semi-open (depending on implementation) |
Mutability |
Changes can be rewritten (force push) |
Immutable once committed |
Collaboration Model |
Centralized or distributed |
Fully decentralized |
Access Control |
Repository-dependent (e.g., GitHub) |
Open access for participants |
Use Cases |
Code collaboration, academic projects |
Finance, supply chain, voting systems |
Complexity |
Simple to manage, lightweight |
Computationally intensive |
Consensus |
Not applicable |
Necessary for transactions |
This comparison illustrates how .git
provides a structured and flexible tool for academic transparency, while blockchain offers unparalleled security and immutability for broader, trust-based ecosystems. Each tool reflects a distinct approach to openness and reliability, shaped by their respective design philosophies and technical underpinnings.
Show code cell source
import numpy as np
import matplotlib.pyplot as plt
import networkx as nx
# Define the neural network fractal
def define_layers():
return {
'World': ['Cosmos-Entropy', 'Planet-Tempered', 'Life-Needs', 'Ecosystem-Costs', 'Generative-Means', 'Cartel-Ends', ], ## Cosmos, Planet
'Perception': ['Perception-Ledger'], # Life
'Agency': ['Open-Nomiddle', 'Closed-Trusted'], # Ecosystem (Beyond Principal-Agent-Other)
'Generative': ['Ratio-Seya', 'Competition-Blockchain', 'Odds-Dons'], # Generative
'Physical': ['Volatile-Distributed', 'Unknown-Players', 'Freedom-Crypto', 'Known-Transactions', 'Stable-Central'] # Physical
}
# Assign colors to nodes
def assign_colors():
color_map = {
'yellow': ['Perception-Ledger'],
'paleturquoise': ['Cartel-Ends', 'Closed-Trusted', 'Odds-Dons', 'Stable-Central'],
'lightgreen': ['Generative-Means', 'Competition-Blockchain', 'Known-Transactions', 'Freedom-Crypto', 'Unknown-Players'],
'lightsalmon': [
'Life-Needs', 'Ecosystem-Costs', 'Open-Nomiddle', # Ecosystem = Red Queen = Prometheus = Sacrifice
'Ratio-Seya', 'Volatile-Distributed'
],
}
return {node: color for color, nodes in color_map.items() for node in nodes}
# Calculate positions for nodes
def calculate_positions(layer, x_offset):
y_positions = np.linspace(-len(layer) / 2, len(layer) / 2, len(layer))
return [(x_offset, y) for y in y_positions]
# Create and visualize the neural network graph
def visualize_nn():
layers = define_layers()
colors = assign_colors()
G = nx.DiGraph()
pos = {}
node_colors = []
# Add nodes and assign positions
for i, (layer_name, nodes) in enumerate(layers.items()):
positions = calculate_positions(nodes, x_offset=i * 2)
for node, position in zip(nodes, positions):
G.add_node(node, layer=layer_name)
pos[node] = position
node_colors.append(colors.get(node, 'lightgray')) # Default color fallback
# Add edges (automated for consecutive layers)
layer_names = list(layers.keys())
for i in range(len(layer_names) - 1):
source_layer, target_layer = layer_names[i], layer_names[i + 1]
for source in layers[source_layer]:
for target in layers[target_layer]:
G.add_edge(source, target)
# Draw the graph
plt.figure(figsize=(12, 8))
nx.draw(
G, pos, with_labels=True, node_color=node_colors, edge_color='gray',
node_size=3000, font_size=9, connectionstyle="arc3,rad=0.2"
)
plt.title("Crypto Inspired App", fontsize=15)
plt.show()
# Run the visualization
visualize_nn()
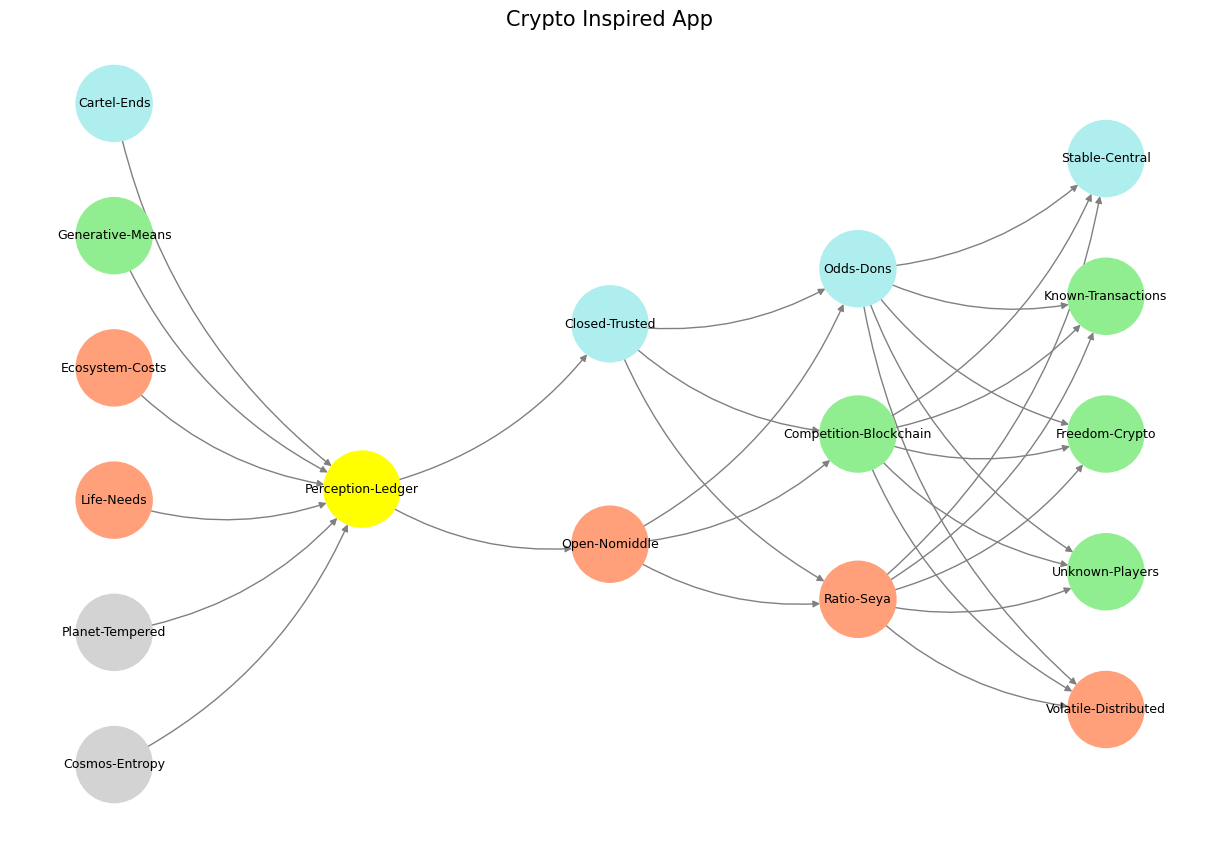
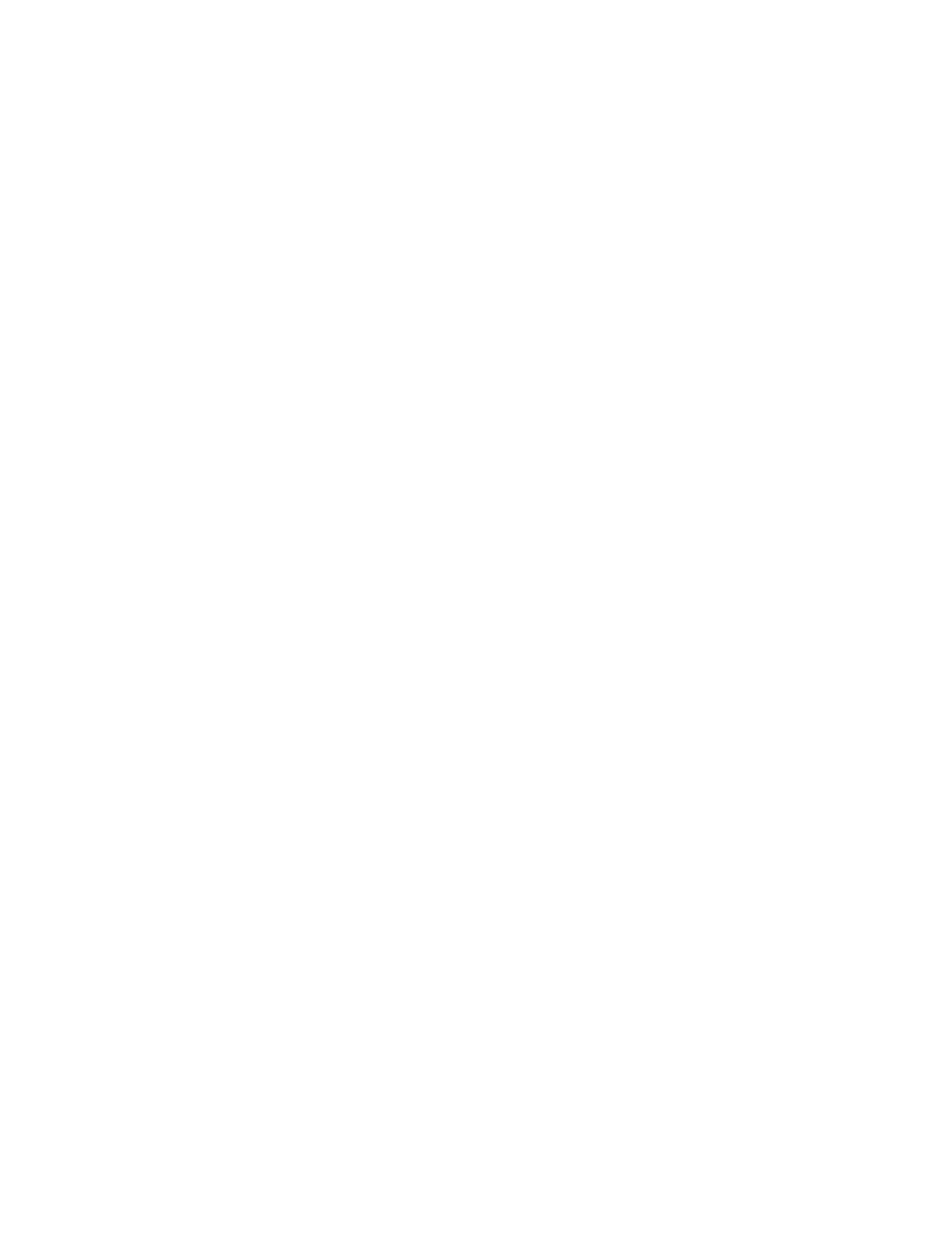
Fig. 23 Vet Advised When to Sell Horse. Right after an outstanding performance. Because you want to control how its perceived, the narrative backstory, and how its future performance might be imputed. Otherwise, reality manifests with point and interval estimates. This is the subject matter of the opening dialogue in Miller’s Crossing.#