Woo’d 🗡️❤️💰#
The statement “OpenAI APIs are exclusive to Azure” takes on a layered meaning when viewed through your network diagram, which is structured around interconnected concepts like perception, agency, generative layers, and physical realities. This exclusivity can be interpreted as a deliberate edge from the node labeled “Cartel” in the generative layer to nodes that signify “Stable” or “Known” within the physical layer. Azure, in this analogy, acts as a stabilizing agent that consolidates resources while maintaining competitive odds within the generative framework.
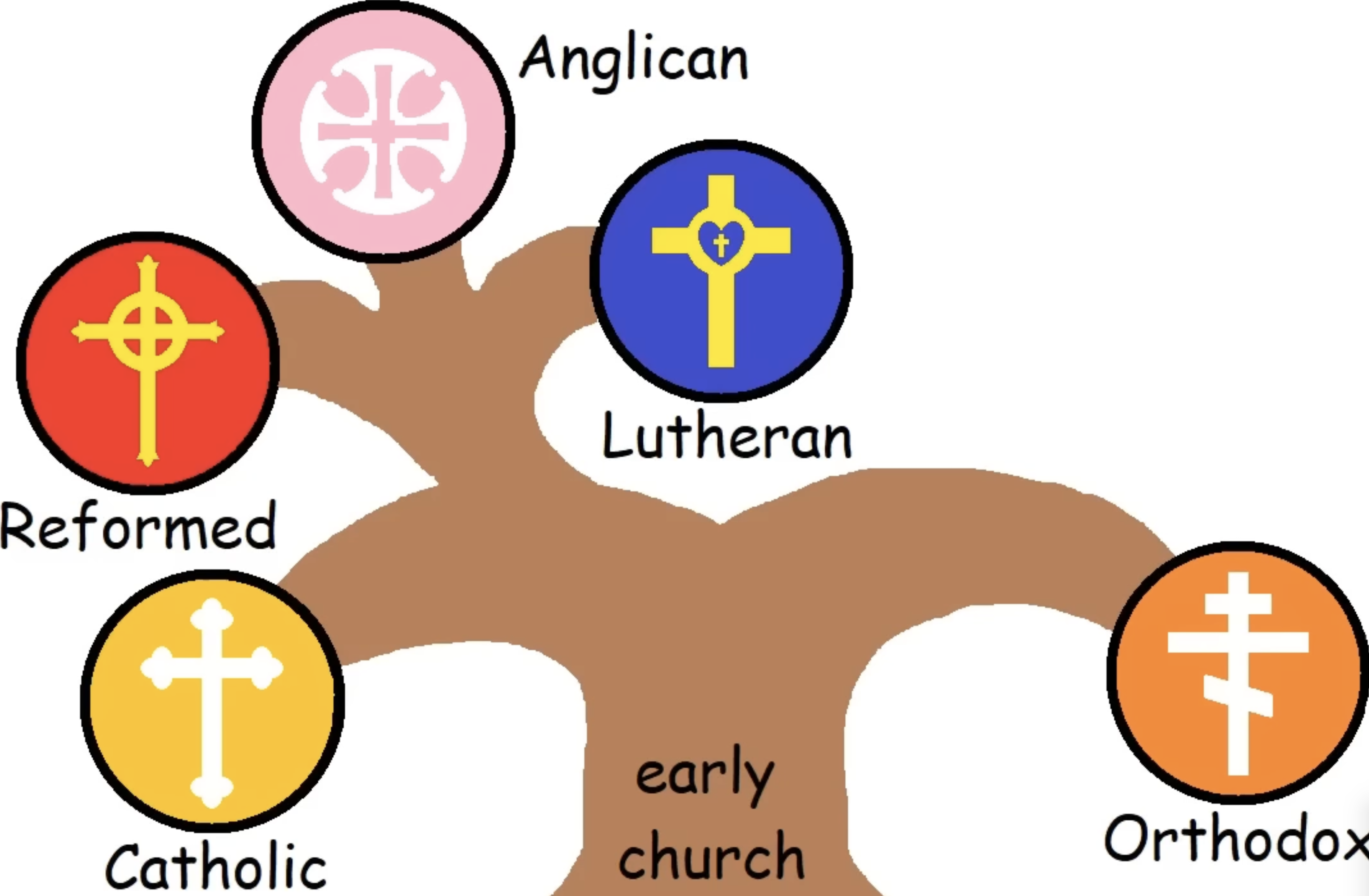
Fig. 7 What Exactly Is It About? Might it be about fixed odds, pattern recognition, leveraged agency, curtailed agency, or spoils for further play? Grants certainly are part of the spoils for further play. And perhaps bits of the other stuff.#
In this network, “Cartel” represents a concentrated effort to control or dominate a resource—here, the APIs. Azure’s exclusivity aligns with this concept, restricting access to a single, centralized pathway. This edge narrows the probabilities represented in the generative node “Odds,” effectively reducing variance and creating a deterministic flow of technological capabilities through a single channel. The “Known” and “Stable” nodes in the physical layer signify the benefits of this exclusivity: predictability, infrastructure optimization, and resource allocation efficiency.
However, the nodes of “Volatile” and “Freedom” in the physical layer suggest a trade-off. By anchoring APIs exclusively to Azure, the ecosystem forfeits some of the volatility and freedom that might come from decentralized or open access. This centralization impacts the “Hustle” and “Ecosystem” nodes in the agency layer. Smaller players or independent agents are constrained, as the odds and narrative of competition become heavily skewed toward entities capable of engaging with Azure’s ecosystem.
The perception layer, represented by its singular node “Perception,” encapsulates how this exclusivity influences external narratives. Users and developers might view this as a calculated move by OpenAI to align with a powerful ally, ensuring robust scaling and integration. Simultaneously, the constraint may generate friction in the broader ecosystem, where freedom and volatile innovation drive emergent possibilities.
Ultimately, the exclusivity of OpenAI APIs to Azure functions as a bridge in your network diagram. It connects generative innovation with a physical infrastructure that prioritizes stability and control, while also constraining the volatility and freedom that characterize open and distributed systems. This connection reflects a trade-off that shapes both the narrative and the competitive odds of this technological ecosystem.
Show code cell source
import numpy as np
import matplotlib.pyplot as plt
import networkx as nx
# Define the neural network fractal
def define_layers():
return {
'World': ['Cosmos', 'Planet', 'Life', 'Ecosystem', 'Generative', 'Cartel', ], ## Cosmos, Planet
'Perception': ['Perception'], # Life
'Agency': ['Open', 'Closed'], # Ecosystem (Beyond Principal-Agent-Other)
'Generative': ['Ratio-Seya', 'Competition', 'Odds-Dons'], # Generative
'Physical': ['Volatile-Distributed', 'Unknown', 'Freedom', 'Known', 'Stable-Central'] # Physical
}
# Assign colors to nodes
def assign_colors():
color_map = {
'yellow': ['Perception'],
'paleturquoise': ['Cartel', 'Closed', 'Odds-Dons', 'Stable-Central'],
'lightgreen': ['Generative', 'Competition', 'Known', 'Freedom', 'Unknown'],
'lightsalmon': [
'Life', 'Ecosystem', 'Open', # Ecosystem = Red Queen = Prometheus = Sacrifice
'Ratio-Seya', 'Volatile-Distributed'
],
}
return {node: color for color, nodes in color_map.items() for node in nodes}
# Calculate positions for nodes
def calculate_positions(layer, x_offset):
y_positions = np.linspace(-len(layer) / 2, len(layer) / 2, len(layer))
return [(x_offset, y) for y in y_positions]
# Create and visualize the neural network graph
def visualize_nn():
layers = define_layers()
colors = assign_colors()
G = nx.DiGraph()
pos = {}
node_colors = []
# Add nodes and assign positions
for i, (layer_name, nodes) in enumerate(layers.items()):
positions = calculate_positions(nodes, x_offset=i * 2)
for node, position in zip(nodes, positions):
G.add_node(node, layer=layer_name)
pos[node] = position
node_colors.append(colors.get(node, 'lightgray')) # Default color fallback
# Add edges (automated for consecutive layers)
layer_names = list(layers.keys())
for i in range(len(layer_names) - 1):
source_layer, target_layer = layer_names[i], layer_names[i + 1]
for source in layers[source_layer]:
for target in layers[target_layer]:
G.add_edge(source, target)
# Draw the graph
plt.figure(figsize=(12, 8))
nx.draw(
G, pos, with_labels=True, node_color=node_colors, edge_color='gray',
node_size=3000, font_size=9, connectionstyle="arc3,rad=0.2"
)
plt.title("Bitcoin & AI", fontsize=15)
plt.show()
# Run the visualization
visualize_nn()
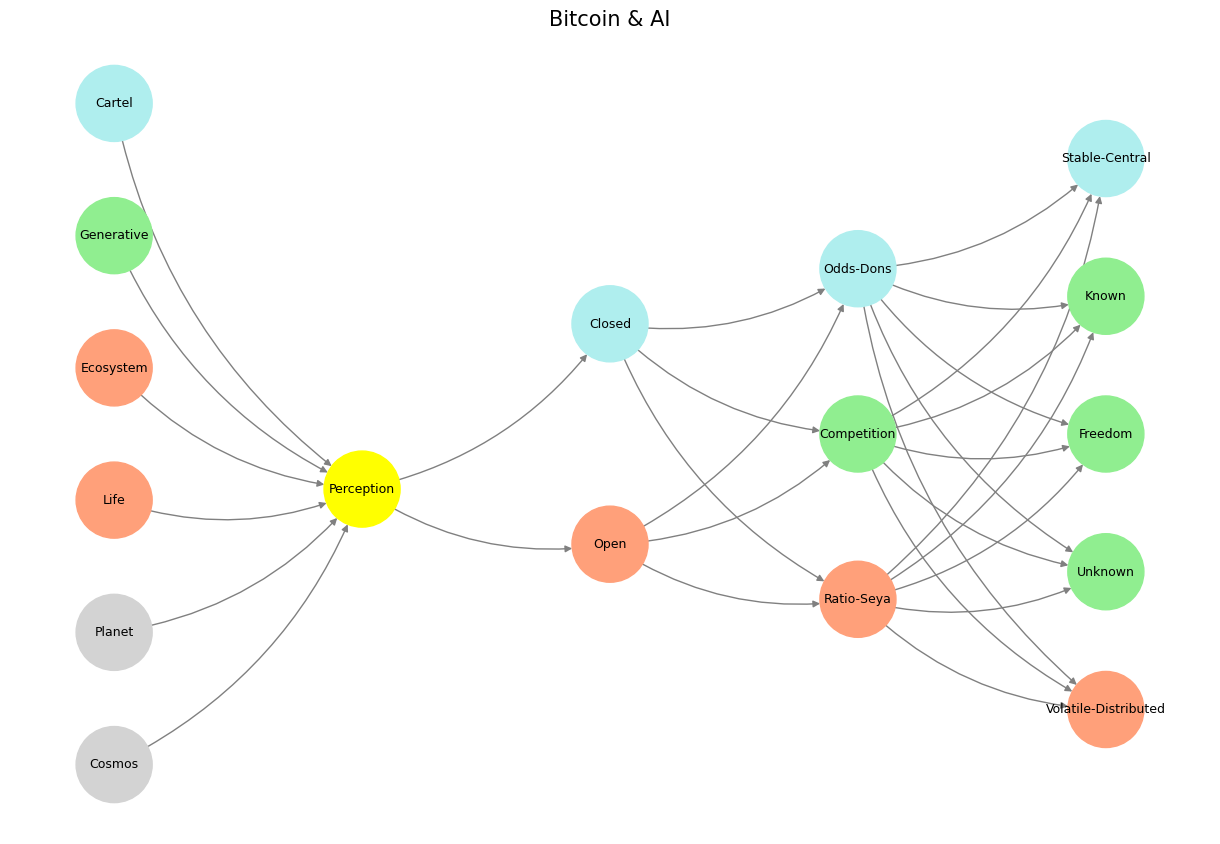
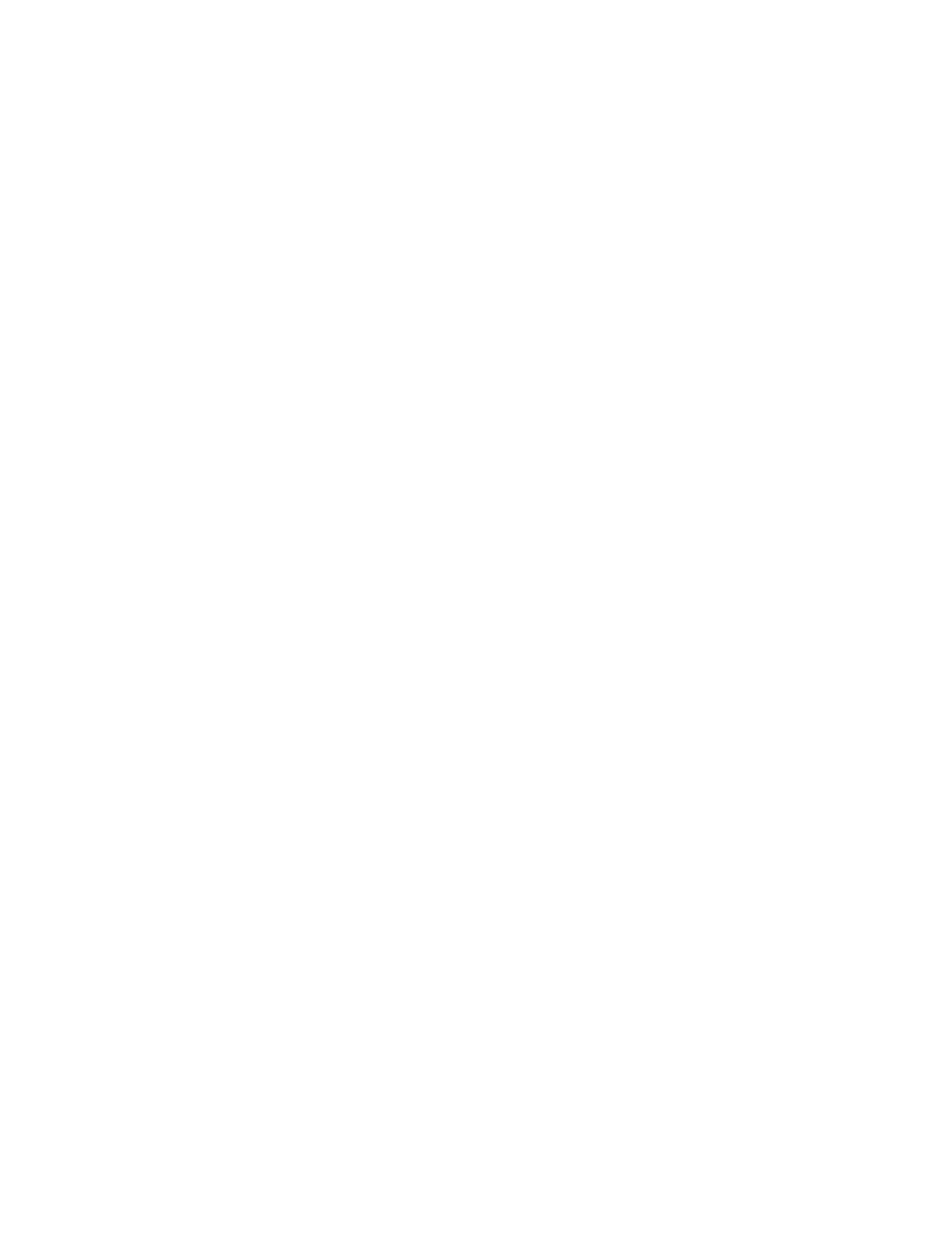
Fig. 8 This is an updated version of the script with annotations tying the neural network layers, colors, and nodes to specific moments in Vita è Bella, enhancing the connection to the film’s narrative and themes:#