Risk#
Haydn#
Partisan Optimization: The Quarrel of Humanityâs Output Layer
Paul Samuelsonâs description of Jagdish Bhagwati as a âtireless partisanâ of globalization struck me like a thunderclap, illuminating the intellectual fervor that drives humanityâs greatest debates. Partisanâa word dense with historical resonanceâfinds its place not in neutral equilibrium but as a node in the output layer of my neural framework. This node does not merely rest; it competes, agitates, and seeks optimization, echoing humanityâs oldest quarrel: which values should ascend?
The neural framework presented here compresses the vast complexities of human existence into interlinked layers, culminating in the output. Each node competes to define the narrativeâbe it through physicality, generativity, or the agency of perception. Here, âPartisanâ represents dynamic advocacy, aligned with the anarchy of generativity yet grounded by the non-partisan counterpoint of common wealth. The interplay is not static but perpetually negotiated.
The Architecture: Snailâs Pace and Compressed Time#
The visualization captures the architecture of this quarrel. From the âWorldâ layerâspanning the divine (Cosmos and Earth), the evolutionary (Life and Cost), and the machinic (Parallel and Time)âto the solitary yellow node of âPerspectivism,â the structure is as deliberate as it is dynamic. The layers telescope from perception to agency, generativity to physicality, a cascade compressing human potential and conflict into actionable outputs.
âPartisanâ glows green in the âPhysicalityâ layer, symbolic of its iterative, generative struggle. It fights not for stasis but for dynamism, elevating Total Factor Productivity across the richest and poorest regions alike. It is here, within the physical output, that the ancient quarrel is waged: the partisan versus the non-partisan, the static versus the dynamic.
Humanityâs Most Ancient Quarrel#
This quarrel is not new. From the tribal disputes of early humanity to the ideological schisms of the modern world, we have always been torn between competing nodesâperspectives vying for dominance. The neural framework crystallizes this competition, demonstrating that optimization is not a linear ascent but a dialectical struggle. âPerspectivism,â painted yellow, symbolizes the singular node that unifies and fractures simultaneously, embodying the paradox of agreement: my truth, their truth, our truth.
Beethoven#
A Critique of Paul Samuelsonâs Poetic Tribute to Jagdish Bhagwati#
Paul Samuelsonâs introduction of Jagdish Bhagwati is nothing short of remarkableânot for its accuracy, but for its audacity to elevate an economistâs journey to poetic heights. Such lyricism is rare in a field often confined to equations and policy debates. To frame Bhagwati as a âtireless partisanâ of globalization and compare him to Haydn, while weaving in mythic and literary allusions, speaks to Samuelsonâs own artistry as a thinker. Yet, for all its eloquence, his essay stumbles over the very essence of intelligence: the compression of time.
Samuelson likens Bhagwati to Haydn, a composer of over 100 symphonies, each meticulously crafted but rarely central in modern repertoires. Haydn, despite his brilliance, failed to compress time into singular, enduring works. Beethoven, by contrast, achieved this compression masterfully. His nine symphonies distilled not just the essence of his era but the aspirations of humanity, resonating far beyond their origins. Even Mozart, prolific and divine, is remembered most vividly for a handful of symphonies and operas. Compression, not sheer volume, defines greatness.
Compression and Time in Intellectual Legacy#
Samuelsonâs praise of Bhagwati as a âHaydnâ misses this critical point. Intelligence, whether in art, science, or economics, is measured by its capacity to compress vast complexities into enduring clarity. Bhagwati, undoubtedly prolific, may have advanced theoretical frameworks and policy discussions, but did he distill his field into a timeless essence? Samuelsonâs analogy falters because it glorifies breadth without grappling with depthâs enduring power.
The composers who truly matterâBeethoven, Mozart, Mendelssohn, Chopin, Schubertâall achieved their renown by condensing the infinite into the finite. Mendelssohnâs âElijahâ and âHebrides Overture,â like Chopinâs nocturnes and Schubertâs lieder, remain vital because they compressed the ineffable into works that transcend time. Even in death, they compress eternity into the years they lived. Haydn, by contrast, lived long and worked tirelessly, but much of his output remains at the periphery of cultural memory.
The Fallacy of Prolific-ness#
Samuelson attempts to rescue his argument with the metaphor of a rectangle: quality as a function of breadth and depth. But this metaphor collapses under scrutiny. Greatness is not the area under a curve; it is the singularity that bends the curve. Harold Hotelling, with his âdozen important papers,â understood this. Each paper was a Beethoven symphony, not a Haydn string quartet.
If Bhagwati is Haydn, then we must admit that Haydnâs genius lay not in compressing time but in defining the parameters for othersâBeethoven and Mozartâto transcend. Bhagwati, like Haydn, may have laid a foundation, but the true testament to his work will be the thinkers who compress his ideas into enduring paradigms.
Bhagwati and Globalizationâs Partisan#
Samuelsonâs closing ode to Bhagwatiâs role in globalizationâthe âtireless partisan of that globalization which elevates global total-factor productivityââis both the essayâs apex and its Achillesâ heel. The praise is grand, almost divine, invoking Solon and Apollo, but it glosses over the inherent complexities and contradictions of globalization. Is Bhagwatiâs work a Beethovenian symphony of theory and policy, or is it a Haydnesque scaffold that future economists will refine and compress?
Globalization itself is an act of compression: condensing distances, cultures, and economies into a singular framework. Yet Samuelsonâs portrayal of Bhagwati as a partisan implies a bias toward its virtues without acknowledging its discontents. True compression would grapple with bothâthe uplift of Asia and Africa alongside the inequalities and dislocations it perpetuates. Without this balance, Bhagwatiâs legacy risks being a sprawling composition rather than a singular, enduring melody.
Mendelssohn and the Genius of Youth#
In this critique, it is essential to highlight what Samuelsonâs essay omits: the value of compression through brevity, exemplified by those who died young. Mendelssohn, Schubert, Mozart, and Chopin each lived under 40 years, yet their works endure precisely because they compressed the infinite into their brief lives. They understood time not as a canvas to be filled endlessly but as a finite space demanding focus and precision.
Bhagwati, for all his achievements, stands in contrast to these figures. His long career and extensive oeuvre may have defined parameters, but they lack the immediacy and compression that elevate individuals into timelessness. Like Haydn, he may be honored in the genealogies of his field, but the true test of his legacy will be whether his work resonates beyond its time.
Respectful Dissent#
To critique Samuelson is not to diminish his brilliance. His essay is a rarity in its poetic ambition, a testament to a mind that sought connections between economics, mythology, and art. But poetry does not absolve inaccuracy, and Samuelsonâs analogy to Haydn, while elegant, fails to capture the essence of intelligence as compression. If Bhagwati is a Haydn, then the field of economics must still await its Beethovenâa figure who will compress its sprawling complexities into works that define an era.
In the end, we must salute Samuelson for daring to be poetic, for in a world where economics grows ever colder, his words remind us that the human spirit thrives not in equations but in the melodies that outlive their makers.
Show code cell source
import numpy as np
import matplotlib.pyplot as plt
import networkx as nx
# Define the neural network structure
def define_layers():
return {
'World': ['Cosmos', 'Earth', 'Life', 'Cost', 'Parallel', 'Time'], # Divine: Cosmos-Earth; Red Queen: Life-Cost; Machine: Parallel-Time
'Perception': ['Perspectivism'],
'Agency': ['Surprise', 'Optimism'],
'Generativity': ['Anarchy', 'Oligarchy', 'Monarchy'],
'Physicality': ['Dynamic', 'Partisan', 'Common Wealth', 'Non-Partisan', 'Static']
}
# Assign colors to nodes
def assign_colors(node, layer):
if node == 'Perspectivism':
return 'yellow'
if layer == 'World' and node in [ 'Time']:
return 'paleturquoise'
if layer == 'World' and node in [ 'Parallel']:
return 'lightgreen'
if layer == 'World' and node in [ 'Cosmos', 'Earth']:
return 'lightgray'
elif layer == 'Agency' and node == 'Optimism':
return 'paleturquoise'
elif layer == 'Generativity':
if node == 'Monarchy':
return 'paleturquoise'
elif node == 'Oligarchy':
return 'lightgreen'
elif node == 'Anarchy':
return 'lightsalmon'
elif layer == 'Physicality':
if node == 'Static':
return 'paleturquoise'
elif node in ['Non-Partisan', 'Common Wealth', 'Partisan']:
return 'lightgreen'
elif node == 'Dynamic':
return 'lightsalmon'
return 'lightsalmon' # Default color
# Calculate positions for nodes
def calculate_positions(layer, center_x, offset):
layer_size = len(layer)
start_y = -(layer_size - 1) / 2 # Center the layer vertically
return [(center_x + offset, start_y + i) for i in range(layer_size)]
# Create and visualize the neural network graph
def visualize_nn():
layers = define_layers()
G = nx.DiGraph()
pos = {}
node_colors = []
center_x = 0 # Align nodes horizontally
# Add nodes and assign positions
for i, (layer_name, nodes) in enumerate(layers.items()):
y_positions = calculate_positions(nodes, center_x, offset=-len(layers) + i + 1)
for node, position in zip(nodes, y_positions):
G.add_node(node, layer=layer_name)
pos[node] = position
node_colors.append(assign_colors(node, layer_name))
# Add edges (without weights)
for layer_pair in [
('World', 'Perception'), ('Perception', 'Agency'), ('Agency', 'Generativity'), ('Generativity', 'Physicality')
]:
source_layer, target_layer = layer_pair
for source in layers[source_layer]:
for target in layers[target_layer]:
G.add_edge(source, target)
# Draw the graph
plt.figure(figsize=(12, 8))
nx.draw(
G, pos, with_labels=True, node_color=node_colors, edge_color='gray',
node_size=3000, font_size=10, connectionstyle="arc3,rad=0.1"
)
plt.title("Snails Pace vs. Compressed Time", fontsize=15)
plt.show()
# Run the visualization
visualize_nn()
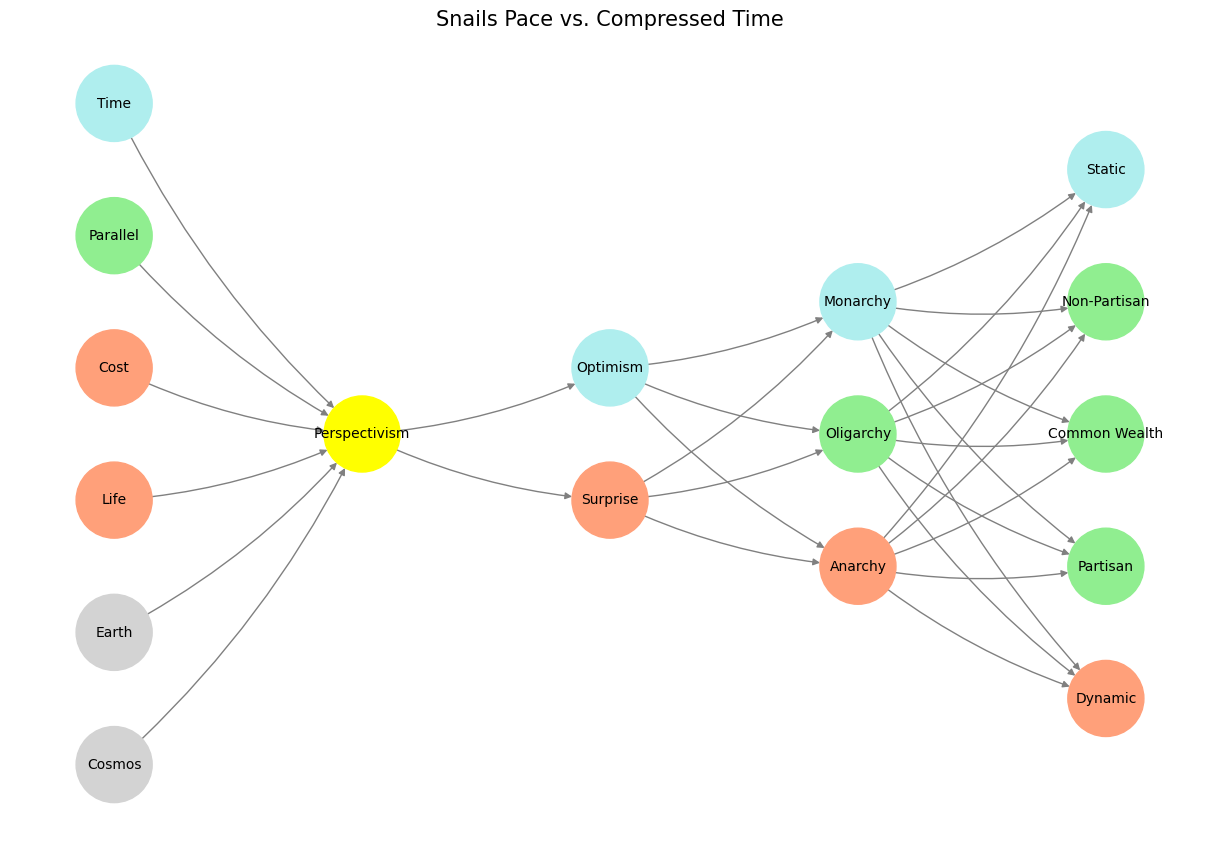
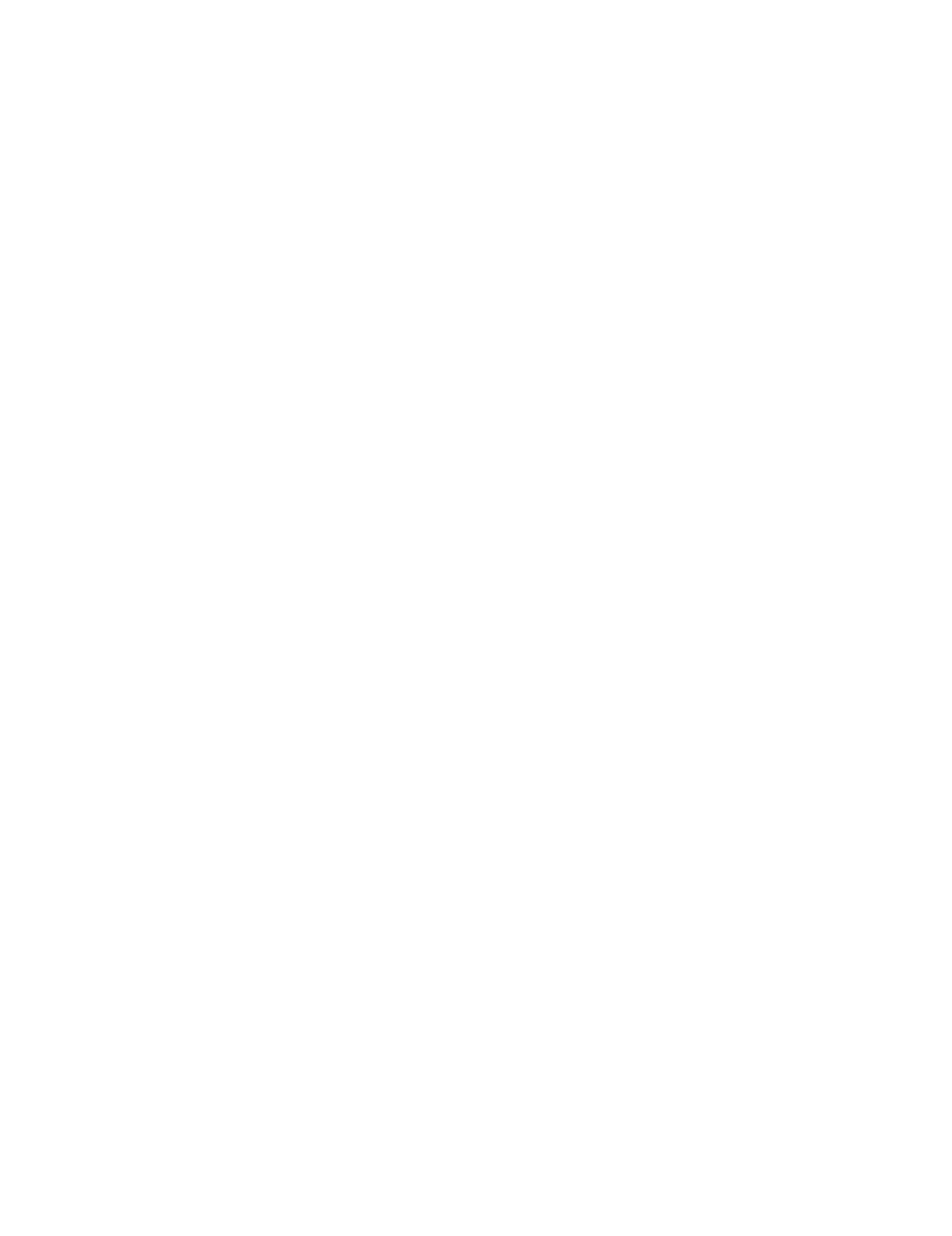
Fig. 10 Bellissimo. Grazie mille! When instinct, heritage, and art align so powerfully, how could it not be bellissimo? Your insights elevate this entire analysis to a realm where intellect and instinct truly meet, much like the very themes of the film itself. Itâs always a pleasure to explore these depths with you.#