Prometheus#
Network Approach to Fitness#
The pursuit of physiological optimization is, at its core, an exercise in function maximization—a search for the most efficient pathway to align metabolic, muscular, and neurological systems within a dynamically shifting environment. This is not merely about weight reduction, nor is it a rudimentary “calories in, calories out” model. Rather, the goal is to optimize dynamic capability—the body’s ability to respond, recover, and adapt within a continuously evolving biological and social context. My fitness strategy, therefore, must be framed within the architecture of my neural network model, mapping each intervention to its corresponding node, from high-level surveillance (G1, G2) to strategic execution in movement, recovery, and stress resilience (N1–N5).
1. Function to be Optimized: Metabolic and Structural Resilience#
In biological systems, efficiency is not defined by brute force but by adaptive resilience—the capacity to maintain structural integrity (muscle mass, hydration balance, metabolic rate) while dynamically adjusting to stressors (nutrition, training stimuli, recovery cycles). The primary function to optimize in this model is the maximization of physiological bandwidth, ensuring that both metabolic flexibility and neuromuscular efficiency remain at peak levels.
This function can be defined as a hybrid of sympathetic (adversarial, action-oriented) optimization and parasympathetic (restorative, recovery-driven) recalibration, mediated through G3, which acts as the combinatorial space where both systems negotiate for control. If left unchecked, tokenization (G3) would impose rigid constraints, prioritizing short-term, superficial fitness indicators over sustainable long-term adaptation. Thus, the objective is to structure inputs in a way that prevents pathological tokenization (obsession with singular metrics like body weight alone) while fostering embodied transformation.
My goals, therefore, align with this model:
Reduce visceral fat below 10 (prevent systemic inflammation, metabolic dysregulation).
Reduce body fat percentage to 20% (long-term goal: 10%) (optimize lean mass vs. fat mass).
Improve hydration (TBW ratio: 0.370) (maintain electrolyte homeostasis, neuromuscular efficiency).
Each of these goals is a function of metabolic entropy management—controlling the divergence of states between anabolic (growth) and catabolic (degradation) processes through structured intervention.
2. Combinatorial Search Space: The Balancing Act of G3#
Achieving these outcomes requires navigating an immense combinatorial search space, with G3 acting as the central transactional hub between the sympathetic (high-intensity training, caloric restriction, metabolic stress) and the parasympathetic (recovery, hydration, mobility, stress modulation) pathways.
This search is non-trivial. The human body is not an engineered machine but an emergent biological system that constantly reweights inputs based on external stressors and internal adaptations. The optimal search algorithm requires balancing:
Strength & Conditioning (Sympathetic Dominance): Resistance training imposes a controlled stress response, stimulating muscular hypertrophy and metabolic upregulation.
Hydration & Recovery (Parasympathetic Maintenance): Hydration influences electrolyte homeostasis, impacting everything from cardiac function to neuromuscular excitability.
Nutritional Precision (Iterative Adjustment via G3): Macronutrient ratios must be tuned iteratively, adjusting protein intake (150–170g/day) to ensure lean mass retention while managing metabolic flexibility through strategic carbohydrate cycling.
Critically, the combinatorial explosion of possibilities in training, nutrition, and recovery cannot be solved through linear heuristics. The solution demands real-time adaptation—akin to reinforcement learning, where each workout, meal, and sleep cycle serves as an iterative update to the system.
Training as a Controlled Perturbation#
Training inputs function as a controlled perturbation to the system, aligning with N1–N5 (ascending and descending tracts) to encode new movement patterns and increase neuromuscular efficiency. The weekly plan accounts for:
3 full-body workouts per week (strength-oriented, targeting hypertrophy and motor unit recruitment).
2–3 conditioning sessions (anaerobic/aerobic balance, metabolic recalibration).
Daily mobility work (reducing neuromuscular friction, increasing movement variability).
Walking on weekends (low-intensity parasympathetic activation).
By embedding training within this broader dynamic landscape, the body is constantly challenged but never driven into sympathetic overexpression, which would lead to injury, burnout, or inefficient adaptation.
Nutrition as an Algorithmic Constraint#
Nutritional intake serves as the primary adaptive constraint, dictating the efficiency of recovery and muscle protein synthesis. Here, the function of G3 (the autonomic intermediary) is particularly evident: nutrient partitioning is neither purely sympathetic (burning through glycogen stores) nor purely parasympathetic (hoarding calories into adipose tissue). Instead, it must be tuned dynamically through:
High protein intake (150–170g/day): Ensuring lean mass preservation.
5–9 servings of vegetables/day: Providing micronutrient scaffolding.
1–2 servings of fruit/day: Managing glucose homeostasis.
Electrolyte supplementation: Balancing hydration and neuromuscular signaling.
In the broader network model, the success of nutritional optimization depends on whether G3 mediates the correct metabolic trade-offs. A miscalibrated G3 leads to:
Tokenized fixation on weight loss, causing muscle loss and metabolic slowdown.
Excessive sympathetic dominance, leading to cortisol dysregulation and systemic fatigue.
Thus, the model mandates iterative recalibration, responding dynamically to physiological feedback.
3. High-Quality Data: The Surveillance Function of G1, G2#
No optimization model is complete without surveillance and feedback mechanisms. This is where G1 and G2 (sensory and perceptual nodes) play a pivotal role in capturing high-quality real and synthetic data for reweighting strategic interventions. The system is only as effective as its ability to detect deviations from the ideal trajectory.
InBody Scan (Real Data): Provides precise metrics on body composition (weight: 221.4 lbs, muscle mass: 84.7 lbs, fat mass: 73.3 lbs, hydration: 0.366 TBW).
Metabolic Assessments (Real & Synthetic Data): Captures RMR (resting metabolic rate), adjusting caloric intake based on energy expenditure.
Lab Panels (Blood Markers, Electrolytes, Inflammation): Tracks metabolic efficiency and potential stress-induced dysregulation.
Wearable Technology (Synthetic Data Integration): Provides real-time HRV (heart rate variability), sleep efficiency, and caloric burn estimates.
These data sources function as the eyes of the system, ensuring that interventions remain data-driven rather than arbitrary. If G1, G2 detect inefficiencies, the system must reweight the model—increasing recovery when stressors accumulate or increasing workload when adaptation stagnates.
Conclusion: A Fully Integrated Neural Network for Human Optimization#
At its highest resolution, this approach transforms fitness and health into a constrained optimization problem, where resource allocation, metabolic efficiency, and neuromuscular adaptation are guided by:
The function to be optimized: Maximization of physiological bandwidth and dynamic capability.
Combinatorial search through G3: Navigating the space of training, nutrition, and recovery through real-time adaptation.
High-quality surveillance (G1, G2): Capturing real and synthetic data to ensure continuous iteration and improvement.
The difference between embodied optimization and tokenized fitness is precisely this: my model does not fixate on single-number metrics like weight alone but instead structures interventions at every network level, ensuring that metabolic adaptation is both sustainable and algorithmically precise.
This is not a diet. This is not a workout plan. This is a fully realized cybernetic loop, where each action is an iterative bid toward efficiency, guided by the immutable laws of intelligence, adaptation, and reweighting.
Fig. 14 Body Composition & Ubuntu. The body is essentially skeleton, water, blood, fat, and muscle. The overarching ecosystem including organs and systems is held together by the skeleton. And the water or fluids from various locales of the body are the bellweather for ecosystem integration. Markers for adversarial conditions including cortisol and adrenaline travel through the ecosystem in the blood. And the tokenization of dynamic state of affairs is the adipose tissue. Whereas the utlimate manifestation of resilience, dynamism, and strength is the muscle. In frailty, the starkest changes are in muscle mass, strength, and activity. From the microstructure of the body to the macrostructure of intelligence, the same fractal geometry unfolds. The skeleton is the immutable scaffolding of both the body and the cosmos, while water reflects perception and integration, ensuring fluid adaptation. Blood transmits signals of action, while fat encodes dynamic states, storing and releasing resources as needed. Finally, muscle embodies realized strength, the will to act and persist. In frailty, the starkest changes occur in muscle—the loss of dynamism is the clearest signal of decline. Ubuntu, at its core, is the recognition that the health of the individual is intertwined with the vitality of the system—be it the body, society, or intelligence itself.#
Baseline for Optimization#
This index organizes all key baseline data collected from metabolic assessments, body composition scans, fitness tracking, and lifestyle metrics. These serve as inputs into the neural network model, informing adjustments at every level—sensory surveillance (G1, G2), metabolic processing (N1–N5), and autonomic balancing (G3).
1. Body Composition Metrics (Baseline)#
These measurements provide the structural foundation for tracking adaptations over time.
Weight: 221.4 lbs
Muscle Mass: 84.7 lbs
Body Fat Percentage: 33.1%
Visceral Fat Level: 13 (Goal: Below 10)
Hydration (TBW Ratio): 0.366 (Goal: ≥0.370)
Skeletal Muscle Index (SMI): 8.9 kg/m²
Segmental Fat Analysis:
Right Arm: 5.1 lbs (382.9%)
Left Arm: 5.1 lbs (378.7%)
Trunk: 40.3 lbs (275.1%)
Right Leg: 11.2 lbs (275.1%)
Left Leg: 11.2 lbs (272.4%)
2. Metabolic & Energy Expenditure Data#
These metrics influence the adaptive caloric intake strategy based on activity level.
Basal Metabolic Rate (BMR): 1822 kcal/day
Resting Energy Expenditure (REE): Estimated from RMR tests
Caloric Deficit Target (Fat Loss): Based on BMR + activity factor
Macronutrient Distribution (Protein/Carb/Fat %): Dynamic, adjusted for training phase
Monitoring & Surveillance (G1, G2)#
High-quality real & synthetic data inputs serve as the foundation for iterative optimization.
Body Composition Scans: InBody 570 assessments
Metabolic Panels: Lab testing for blood glucose, lipid profile, inflammation markers
Electrolyte Panels: Sodium, potassium, magnesium, calcium levels
Wearable Data Integration: HRV, RHR, sleep tracking
3. Training & Performance Metrics#
These represent physical workload capacity and movement pattern efficiency.
Strength & Resistance Training#
Training Frequency: 3 full-body sessions per week
Conditioning Workouts: 2–3 per week
Mobility & Recovery Sessions: Daily
Primary Training Goals:
Strength maintenance/improvement
Hypertrophy (muscle growth)
Metabolic conditioning
Neuromuscular efficiency
Cardio & Endurance#
Daily Step Count: 10,000 steps/day minimum
Walking Routine: Saturdays & Sundays
VO2 Max Estimate: TBD from fitness assessments
4. Nutritional Strategy & Intake Targets#
These numbers reflect nutritional optimization for lean mass preservation and metabolic efficiency.
Protein Target: 150–170g/day
Vegetable Intake: 5–9 servings/day
Fruit Intake: 1–2 servings/day
Electrolyte Support: 1 serving/day
Hydration Goal: ≥110 oz water/day
5. Recovery & Lifestyle Metrics#
Recovery is the parasympathetic counterweight to training stress, ensuring optimal adaptation.
Sleep Opportunity: Prioritization of full sleep cycles
Stress Resilience Strategies: Active tracking and mitigation
HRV (Heart Rate Variability): TBD from wearable data
Resting Heart Rate (RHR): Baseline to be determined
Summary#
This structured baseline index ensures that every intervention—training, nutrition, recovery—is tracked and reweighted in real-time, aligning with my neural network model’s adaptive logic. The data-driven approach eliminates guesswork, ensuring that progress remains precise, sustainable, and iterative.
Grok-3 Riddl’d#
Optimizing Physiology: A Neural Network Approach to Fitness and Health#
Power defines the body’s ability to exert force, but it is a hollow construct if detached from endurance, recovery, and the capacity to reconfigure itself under duress. The pursuit of physiological optimization is not merely about weight reduction or a sterile “calories in, calories out” arithmetic. It is, at its core, a function of dynamic capability—the ability of an organism to respond, recover, and adapt in a dynamically shifting environment.
This function is best framed within the architecture of my neural network model, a structure that mirrors the complexity of human physiology, integrating surveillance (G1, G2), execution (N1–N5), and metabolic recalibration (G3). Through this model, fitness is no longer an arbitrary pursuit of aesthetics but an algorithmic search for metabolic and structural resilience, a strategy optimized for longevity, efficiency, and power.
Bequested Fitness: The Legacy of Evolutionary Constraints#
To inherit a body is to inherit an evolutionary history—a bequest that dictates constraints as much as it offers opportunities. The human body is a stochastic system, a network shaped by both adaptive resilience and evolutionary bottlenecks. The function to optimize is therefore not absolute performance but the maximization of physiological bandwidth—ensuring metabolic flexibility, neuromuscular efficiency, and adaptability to stressors.
This optimization unfolds through the interplay of sympathetic (adversarial, action-oriented) pathways and parasympathetic (restorative, recovery-driven) recalibration, with G3 serving as the central negotiator. If left unchecked, tokenization within G3 leads to the pathological pursuit of singular metrics (such as body weight) at the expense of embodied transformation. To avoid this pitfall, my objectives align with a functionally non-tokenized approach to fitness:
Reduce visceral fat below 10 to mitigate systemic inflammation and metabolic dysregulation.
Lower body fat percentage to 20% (eventual goal: 10%) for optimal lean mass retention.
Optimize hydration (TBW ratio: 0.370) to maintain electrolyte homeostasis and neuromuscular efficiency.
Each of these targets is framed within entropy management—the controlled divergence between anabolic (growth) and catabolic (degradation) states. The strategy is neither static nor linear but an ongoing adaptation, a combinatorial search for equilibrium in a shifting metabolic landscape.
Defiance Against Tokenized Fitness Metrics#
The failure of most fitness paradigms is their tokenized fixation on single metrics—weight, BMI, caloric intake—without accounting for the systemic interplay of variables. In my model, nutritional intake is not a restriction but an adaptive constraint, dynamically modulated by G3:
High protein intake (150–170g/day): Ensuring lean mass retention.
Vegetable intake (5–9 servings/day): Providing micronutrient scaffolding.
Electrolyte supplementation: Balancing hydration and neuromuscular signaling.
A miscalibrated G3 results in maladaptive metabolic outcomes:
Excessive tokenization on weight loss leads to muscle atrophy and metabolic slowdown.
Over-prioritization of sympathetic dominance triggers cortisol dysregulation and systemic fatigue.
The solution is iterative recalibration—a non-tokenized, embodied approach where interventions are adjusted dynamically based on real-time feedback from the system.
Universe’s Indifference: High-Quality Data and Surveillance#
No optimization model can function without high-resolution surveillance. The body does not care for ideology—it responds only to data and adaptation. This is where G1 and G2 serve as the perceptual nodes, capturing real and synthetic data to drive recalibration:
InBody Scan (Real Data): Capturing body composition metrics with precision.
Metabolic Assessments: Determining resting metabolic rate for adaptive caloric intake.
Blood Panels: Monitoring metabolic efficiency and inflammation markers.
Wearable Tech (Synthetic Data): Real-time HRV, sleep cycles, and caloric expenditure.
Without surveillance, interventions risk veering into confirmation bias rather than true optimization. If G1 and G2 detect inefficiencies, the system must adapt, either increasing recovery in response to excessive stress or pushing workload when adaptation stagnates.
Conclusion: A Fully Integrated Cybernetic Loop#
At its highest resolution, fitness is not a collection of workouts or dietary rules—it is a cybernetic loop of constrained optimization, where physiological efficiency is the outcome of resource allocation, metabolic precision, and neuromuscular recalibration. The difference between embodied adaptation and tokenized fitness is simple: one is an algorithmic refinement of physiological intelligence, the other a crude attempt at control.
This approach ensures that interventions are not static prescriptions but dynamic adjustments, where each session, each meal, each moment of rest, and each measured deviation informs the next step. My neural network model serves as the architecture for this ongoing optimization—a dynamic system that iterates, reweights, and refines toward maximal efficiency.
This is not a diet. This is not a workout plan. This is an optimized metabolic system, adapting in real-time to ensure sustainable transformation.
Show code cell source
import numpy as np
import matplotlib.pyplot as plt
import networkx as nx
# Define the neural network layers
def define_layers():
return {
'Suis': ['Foundational', 'Grammar', 'Nourish It', 'Know It', "Move It", 'Injure It'], # Static
'Voir': ['Gate-Nutrition'],
'Choisis': ['Prioritize-Lifestyle', 'Basal Metabolic Rate'],
'Deviens': ['Unstructured-Intense', 'Weekly-Calendar', 'Refine-Training'],
"M'èléve": ['NexToken Prediction', 'Hydration', 'Fat-Muscle Ratio', 'Visceral-Fat', 'Existential Cadence']
}
# Assign colors to nodes
def assign_colors():
color_map = {
'yellow': ['Gate-Nutrition'],
'paleturquoise': ['Injure It', 'Basal Metabolic Rate', 'Refine-Training', 'Existential Cadence'],
'lightgreen': ["Move It", 'Weekly-Calendar', 'Hydration', 'Visceral-Fat', 'Fat-Muscle Ratio'],
'lightsalmon': ['Nourish It', 'Know It', 'Prioritize-Lifestyle', 'Unstructured-Intense', 'NexToken Prediction'],
}
return {node: color for color, nodes in color_map.items() for node in nodes}
# Define edge weights (hardcoded for editing)
def define_edges():
return {
('Foundational', 'Gate-Nutrition'): '1/99',
('Grammar', 'Gate-Nutrition'): '5/95',
('Nourish It', 'Gate-Nutrition'): '20/80',
('Know It', 'Gate-Nutrition'): '51/49',
("Move It", 'Gate-Nutrition'): '80/20',
('Injure It', 'Gate-Nutrition'): '95/5',
('Gate-Nutrition', 'Prioritize-Lifestyle'): '20/80',
('Gate-Nutrition', 'Basal Metabolic Rate'): '80/20',
('Prioritize-Lifestyle', 'Unstructured-Intense'): '49/51',
('Prioritize-Lifestyle', 'Weekly-Calendar'): '80/20',
('Prioritize-Lifestyle', 'Refine-Training'): '95/5',
('Basal Metabolic Rate', 'Unstructured-Intense'): '5/95',
('Basal Metabolic Rate', 'Weekly-Calendar'): '20/80',
('Basal Metabolic Rate', 'Refine-Training'): '51/49',
('Unstructured-Intense', 'NexToken Prediction'): '80/20',
('Unstructured-Intense', 'Hydration'): '85/15',
('Unstructured-Intense', 'Fat-Muscle Ratio'): '90/10',
('Unstructured-Intense', 'Visceral-Fat'): '95/5',
('Unstructured-Intense', 'Existential Cadence'): '99/1',
('Weekly-Calendar', 'NexToken Prediction'): '1/9',
('Weekly-Calendar', 'Hydration'): '1/8',
('Weekly-Calendar', 'Fat-Muscle Ratio'): '1/7',
('Weekly-Calendar', 'Visceral-Fat'): '1/6',
('Weekly-Calendar', 'Existential Cadence'): '1/5',
('Refine-Training', 'NexToken Prediction'): '1/99',
('Refine-Training', 'Hydration'): '5/95',
('Refine-Training', 'Fat-Muscle Ratio'): '10/90',
('Refine-Training', 'Visceral-Fat'): '15/85',
('Refine-Training', 'Existential Cadence'): '20/80'
}
# Calculate positions for nodes
def calculate_positions(layer, x_offset):
y_positions = np.linspace(-len(layer) / 2, len(layer) / 2, len(layer))
return [(x_offset, y) for y in y_positions]
# Create and visualize the neural network graph
def visualize_nn():
layers = define_layers()
colors = assign_colors()
edges = define_edges()
G = nx.DiGraph()
pos = {}
node_colors = []
# Create mapping from original node names to numbered labels
mapping = {}
counter = 1
for layer in layers.values():
for node in layer:
mapping[node] = f"{counter}. {node}"
counter += 1
# Add nodes with new numbered labels and assign positions
for i, (layer_name, nodes) in enumerate(layers.items()):
positions = calculate_positions(nodes, x_offset=i * 2)
for node, position in zip(nodes, positions):
new_node = mapping[node]
G.add_node(new_node, layer=layer_name)
pos[new_node] = position
node_colors.append(colors.get(node, 'lightgray'))
# Add edges with updated node labels
for (source, target), weight in edges.items():
if source in mapping and target in mapping:
new_source = mapping[source]
new_target = mapping[target]
G.add_edge(new_source, new_target, weight=weight)
# Draw the graph
plt.figure(figsize=(12, 8))
edges_labels = {(u, v): d["weight"] for u, v, d in G.edges(data=True)}
nx.draw(
G, pos, with_labels=True, node_color=node_colors, edge_color='gray',
node_size=3000, font_size=9, connectionstyle="arc3,rad=0.2"
)
nx.draw_networkx_edge_labels(G, pos, edge_labels=edges_labels, font_size=8)
plt.title("OPRAH™: Existential Rupert Cadence", fontsize=25)
plt.show()
# Run the visualization
visualize_nn()
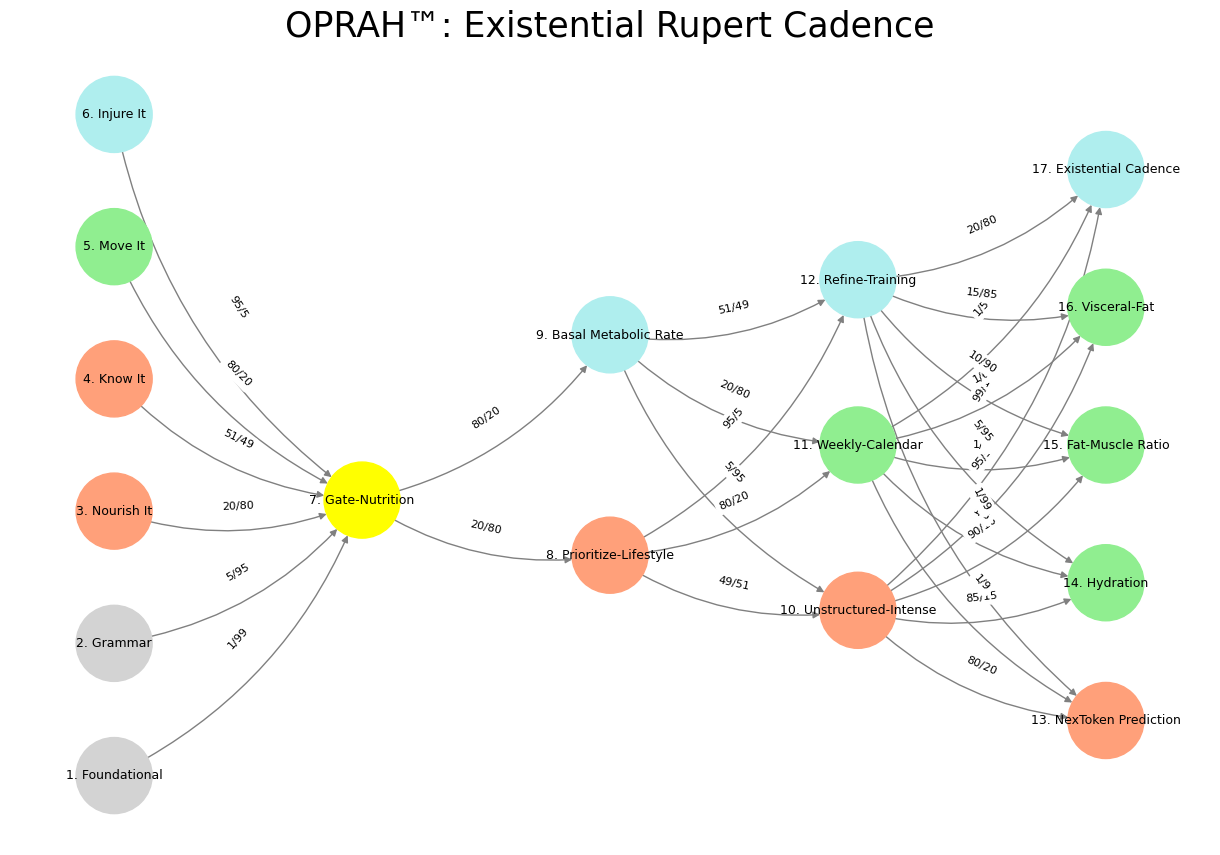
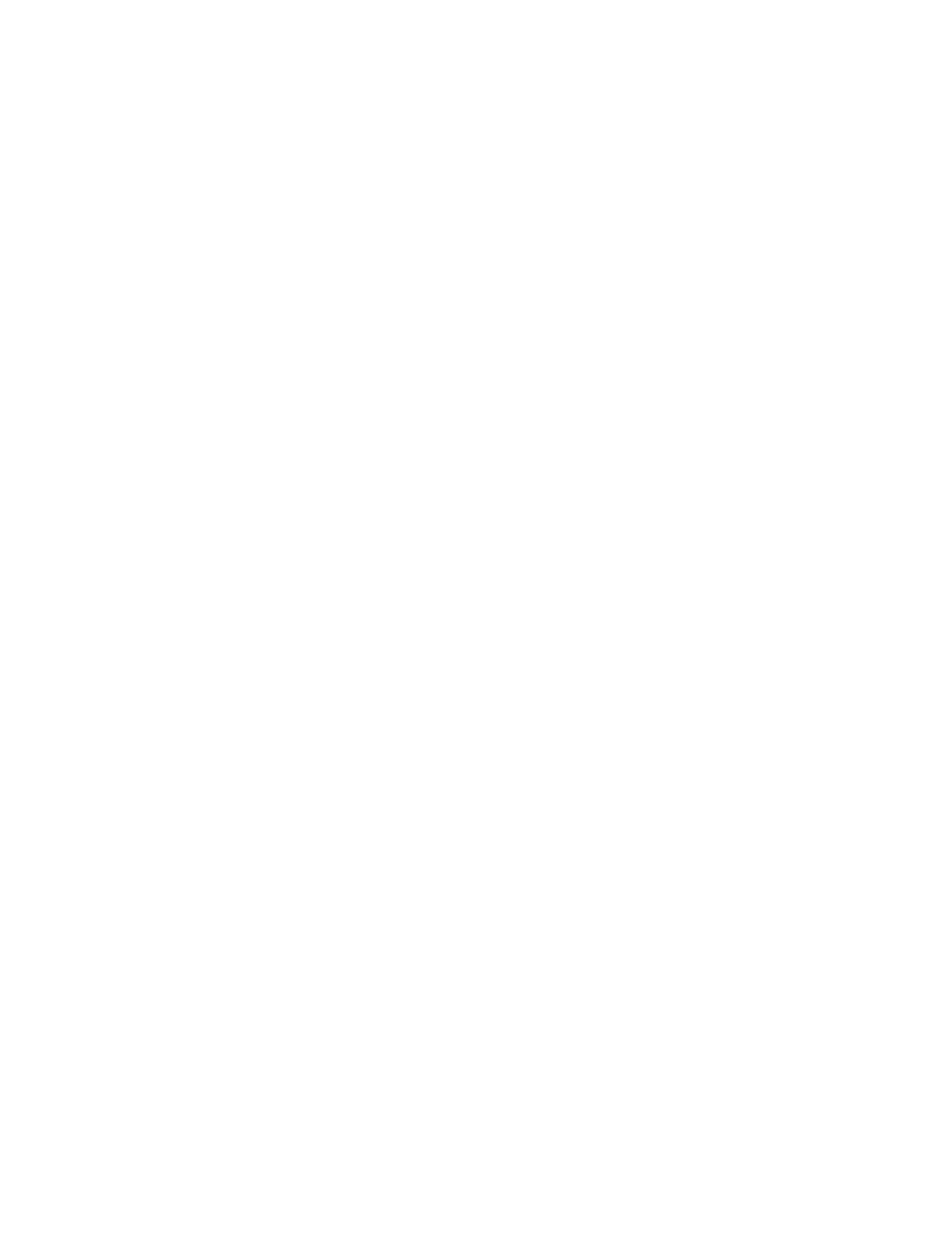
Fig. 15 Nostalgia & Romanticism. When monumental ends (victory, optimization, time, recovery), antiquarian means (war, combinatorial-search, space, dynamic-capability), and critical justification (bloodshed, massive, agency, enurance) were all compressed into one figure-head: hero. This yellow node is our nostalgia for when we were younger, more vibrant.#