Resilience š”ļøā¤ļøš°#
The Strategic Bequest Motive: A Multiscalar Game Through Murdochās Lens and Jamesā Jacob
Hereās Take 2, reframing the strategic bequest motive from Bernheim, Shleifer, and Summersā paper as a multiscalar game across your five levelsāIgnorance, Bequest, Strategy, Knowledge, and Certaintyāusing your game metaphors (šŖš²š°, ā„ļøā¦ļøā£ļø, ššļø, š¤ŗāļøš, š„
šÆ). Iāll weave in your neural networkās yellow node as the Bequest thread, tie it to the Murdoch family drama (including the September 2024 lawsuit and James Murdochās February 24, 2025, article), and explore Jamesā christening as āJacobā for added depth. This is .md
-formatted for your .ipynb
, ready to vibe with your visualization code.

Fig. 11 Influencer (Witness) vs. Indicator (Trial). These reflects sentiments in 2 Chronicles 17:9-12
: For the eyes of the Lord run to and fro throughout the whole earth, to show Himself strong (Nowamaani) on behalf of them whose heart is perfect toward Him. This parallels Shakespeareās image of the poetās eye āin a fine frenzy rolling,ā scanning from heaven to earth and back. Ukubona beyond the mundane (network layers 3-5), upstream to first prinicples of the ecosystem (layer 1). This is the duty of intelligence and what our App and its variants in and beyond clinical medicine aims for ā to elevate perception, agency, and games for all. To leave a shrinking marketplace for the serpent in Eden, for snakeoil salesmen, for fraudstars. To shrink the number of the gullible.#
Ignorance šŖ š² š°: The Coin Flip of Raw Chance#
Imagine a world where nothing you do mattersāa coin flip (šŖ), dice roll (š²), or roulette spin (š°). This is Ignorance, the paperās starting point: pure randomness, no leverage, no strategy. Bequests here are just wealth dropped into a void, like a testator tossing coins into a wellāoutcomes are fixed, indifferent to intent. The yellow node (Bequest) is a raw inputāa will, a trust, a contractāprocessed into the game but untouched by cunning. The paper sidesteps this chaos, arguing bequests arenāt accidental (contra Daviesā āaccidental bequestsā model); theyāre deliberate, escaping this layerās futility.
Murdoch Angle: Rupert Murdochās early life couldāve been thisāa chancy inheritance from his fatherās modest Aussie papers. But he didnāt play the roulette wheel of fate. The September 2024 lawsuit shows him rejecting Ignoranceārewriting the trust isnāt a dice roll; itās a move to control, not succumb to, randomness. X might buzz (hypothetically, February 2025), āRupert never flipped coinsāhe stacked the deck,ā reflecting his refusal of this layerās passivity.
Bequest ā„ļø ā¦ļø ā£ļø: Pokerās Mind Games#
Now weāre in Bequest, the poker table (ā„ļøā¦ļøā£ļø) where bluffing, perception, and partial knowledge rule. The paperās core shines here: testators use bequests as cards, conditioning shares on actions (e.g., attention from kids) to sway behavior. Itās not about whatās known but whatās believedāa parent threatens disinheritance, but credibility hinges on having multiple players (two+ kids). The yellow node shifts from raw input to a mask, a deceptive tool wielded with Strategic finesse and Transactional stakes. The paperās modelāparents extracting āsurplusā (care, loyalty)āis pure psychological warfare, a bluff only works if the kids buy it.
Murdoch Angle: The Murdoch trust is this game incarnate. Rupertās 1999 āirrevocableā trust split control among four kids (Lachlan, James, Elisabeth, Prudence), but the September 2024 lawsuit reveals his Bequest play: amending it to favor Lachlan, bluffing the others into submission. Jamesā February 24, 2025, NYT articleāslamming Fox Newsā climate denialācalls this bluff, exposing the mask. X might quip, āJames folded his handāRupertās dealing from the bottom now,ā capturing the Adversarial rift as siblings vie over perception.
Jacobās Meaning: James Rupert Jacob Murdochāchristened āJacobā (Hebrew: āsupplanterā or āheel-grabberā)āfits this layer eerily. In Genesis, Jacob tricks Esau out of his birthright, mirroring Jamesā potential to upend Lachlanās inheritance. His rebellion (resigning from News Corp in 2020, critiquing Rupert in 2025) casts him as the supplanter, using Bequestās mind games to challenge the family deck.
Strategy š šļø: Razor-Thin Triumphs#
Enter Strategy, the horse race (š) or F1 duel (šļø)āvictory by a nose, optimization under pressure. The paperās testator refines the poker bluff into a precise execution: crafting a bequest rule (e.g., āmost attentive kid winsā) that balances Adversarial competition and Transactional payoff. With two+ beneficiaries, every move countsācredibility isnāt just belief but a split-second edge. The yellow node becomes an equilibrium, teetering between efficiency (maximizing surplus) and aggression (threatening disinheritance). Unlike Ignoranceās chaos or Bequestās fog, this is weaponized precision, where Prosody (timing) decides the race.
Murdoch Angle: Rupertās lawsuit is this razorās edgeātilting the trust to Lachlan risks alienating James, Elisabeth, and Prudence, but itās a calculated Strategy to lock in Foxās conservative Cadence. Jamesā article counters with his own sprint, aligning with Biden/Harris (2020 donation, 2024 endorsement) to outpace Rupertās ideological lap. The December 2024 ruling against Rupert (Nevada court rejecting the amendment) shows the raceās fragilityāLachlanās lead slipped, per the paperās multi-player logic. X might say, āRupertās F1 stalledāJames hit the nitrous,ā highlighting the Strategic misfire.
Jacobās Echo: Jacobās wrestling with the angel (Genesis 32) fits hereāa grueling, margin-thin struggle for blessing. James, as āJacob,ā grapples with Rupertās empire, his 2025 article a Strategic lunge to redefine the finish line, not just inherit it.
Knowledge 𤺠āļø š: Chessmasterās Dominion#
Knowledge is chess (āļø), warfare (š¤ŗ), or resource mastery (š)ādeterministic, where skill trumps luck. The paperās econometric evidence lives here: data (e.g., LRHS) shows multi-child families with bequeathable wealth (stocks, homes) get more attention, a predictable outcome of Strategic rules mastered over time. Single-child families? No leverage, no gameāproving the two-player minimum. The yellow node is an archiveāwealth stats, behavioral patternsādeployed with Motive-driven precision. Unlike Bequestās bluff or Strategyās speed, this is Operational certainty: preparation wins.
Murdoch Angle: Rupertās empireāFox, News Corpāis a chessboard, built over decades with Knowledge of media and politics (Thatcher, Reagan). The lawsuit aimed to cement this mastery, but Jamesā article leverages his own archiveāyears at News Corp, insight into Foxās āmenaceā (per The Atlantic, February 2025)āto checkmate Rupertās plan. The courtās rejection (calling it a ācharadeā) validates the paper: Knowledge falters without credible threats. X might note, āJames played the long gameāRupertās pawns got pinned.ā
Jacobās Mastery: Jacobās ladder (Genesis 28)āa vision of structured ascentāmirrors this. James, the ābrightestā sibling (per Wikipedia), climbs with calculated moves (Lupa Systems, Bodhi Tree), using Knowledge to outmaneuver Lachlanās kingly claim.
Certainty š„ šÆ: Destinyās Fulfillment#
Finally, Certainty (š„ šÆ)āfaith, love, destinyāwhere the game ends, and purpose reigns. The paper hints at this: strategic bequests seek a Victory beyond wealthāa legacy etched in time. The yellow node transforms into inevitability, a Cadence of fulfillment beyond Adversarial play. Itās the testatorās dream of immortality, though the paper warns cosmic indifference looms (no bequest outruns entropy). This is Existentialāaction fulfills, doesnāt shape, the outcome.
Murdoch Angle: Rupertās Certainty is Foxās conservative soul, a media Eden he believes Lachlan will tend. The lawsuit was his prayer to secure this, but Jamesā 2025 defianceācalling Fox a āmenaceā (The Atlantic)āshatters it, embracing a divergent destiny (climate focus, liberal lean). The siblingsā post-trial letter (Thanksgiving 2024, per The Guardian) pleads for healing, a faint Certainty of family over empire. X might muse, āRupertās heaven crumbledāJames chose his own star,ā echoing the paperās limit: Certainty eludes control.
Jacobās Transcendence: Jacob becomes Israel (Genesis 35)āa name shift to āhe who strives with Godāāsymbolizing Certainty. James, as āJacob,ā strives beyond Rupertās orbit, his article a manifesto of purpose, not just rebellion, aligning with the paperās cosmic tension: Victory or void?
Game Layers Recap#
Level |
Description |
Games |
Node Role |
Murdoch Example |
---|---|---|---|---|
Ignorance |
Pure chance, no leverage |
Coin Toss, Dice, Roulette |
Raw input (trust, wealth) |
Rupertās early inheritance |
Bequest |
Deception, perception |
Poker, Bluffing |
Mask (trust as leverage) |
2024 lawsuit bluff |
Strategy |
Razor-thin optimization |
Horse Racing, F1 |
Equilibrium (control edge) |
Lachlan vs. James sprint |
Knowledge |
Deterministic mastery |
Chess, Warfare |
Archive (data, empire) |
Empirical sibling dynamics |
Certainty |
Purpose beyond strategy |
Faith, Destiny |
Inevitability (legacy) |
Rupertās vision vs. Jamesā rift |
Jacobās Name: A Final Twist#
āJacobāāāsupplanterā or āhe who strivesāāthreads James through all layers. In Ignorance, heās born into Rupertās game; in Bequest, he supplants with defiance; in Strategy, he races to redefine; in Knowledge, he masters his exit; in Certainty, he seeks a new Israel, not Rupertās. This christening (December 13, 1972, per Wikipedia) isnāt randomāitās a prophetic riff on the paperās motive: strategic bequests breed Adversarial heirs, and James, the Jacob, embodies the struggle.
This multiscalar lensāamped by Murdochās real-time sagaāextends the paperās econometrics into a fractal epic. Does Certainty crown Rupertās empire, or does Jamesā Knowledge upend it? The yellow node dances across, a riddle unresolved.
Show code cell source
import numpy as np
import matplotlib.pyplot as plt
import networkx as nx
# Define the neural network layers
def define_layers():
return {
'Suis': ['Foundational', 'Grammar', 'Syntax', 'Punctuation', "Rhythm", 'Time'], # Static
'Voir': ['Data Flywheel'],
'Choisis': ['LLM', 'User'],
'Deviens': ['Action', 'Token', 'Rhythm.'],
"M'èléve": ['Victory', 'Payoff', 'NexToken', 'Time.', 'Cadence']
}
# Assign colors to nodes
def assign_colors():
color_map = {
'yellow': ['Data Flywheel'],
'paleturquoise': ['Time', 'User', 'Rhythm.', 'Cadence'],
'lightgreen': ["Rhythm", 'Token', 'Payoff', 'Time.', 'NexToken'],
'lightsalmon': ['Syntax', 'Punctuation', 'LLM', 'Action', 'Victory'],
}
return {node: color for color, nodes in color_map.items() for node in nodes}
# Define edge weights (hardcoded for editing)
def define_edges():
return {
('Foundational', 'Data Flywheel'): '1/99',
('Grammar', 'Data Flywheel'): '5/95',
('Syntax', 'Data Flywheel'): '20/80',
('Punctuation', 'Data Flywheel'): '51/49',
("Rhythm", 'Data Flywheel'): '80/20',
('Time', 'Data Flywheel'): '95/5',
('Data Flywheel', 'LLM'): '20/80',
('Data Flywheel', 'User'): '80/20',
('LLM', 'Action'): '49/51',
('LLM', 'Token'): '80/20',
('LLM', 'Rhythm.'): '95/5',
('User', 'Action'): '5/95',
('User', 'Token'): '20/80',
('User', 'Rhythm.'): '51/49',
('Action', 'Victory'): '80/20',
('Action', 'Payoff'): '85/15',
('Action', 'NexToken'): '90/10',
('Action', 'Time.'): '95/5',
('Action', 'Cadence'): '99/1',
('Token', 'Victory'): '1/9',
('Token', 'Payoff'): '1/8',
('Token', 'NexToken'): '1/7',
('Token', 'Time.'): '1/6',
('Token', 'Cadence'): '1/5',
('Rhythm.', 'Victory'): '1/99',
('Rhythm.', 'Payoff'): '5/95',
('Rhythm.', 'NexToken'): '10/90',
('Rhythm.', 'Time.'): '15/85',
('Rhythm.', 'Cadence'): '20/80'
}
# Calculate positions for nodes
def calculate_positions(layer, x_offset):
y_positions = np.linspace(-len(layer) / 2, len(layer) / 2, len(layer))
return [(x_offset, y) for y in y_positions]
# Create and visualize the neural network graph
def visualize_nn():
layers = define_layers()
colors = assign_colors()
edges = define_edges()
G = nx.DiGraph()
pos = {}
node_colors = []
# Create mapping from original node names to numbered labels
mapping = {}
counter = 1
for layer in layers.values():
for node in layer:
mapping[node] = f"{counter}. {node}"
counter += 1
# Add nodes with new numbered labels and assign positions
for i, (layer_name, nodes) in enumerate(layers.items()):
positions = calculate_positions(nodes, x_offset=i * 2)
for node, position in zip(nodes, positions):
new_node = mapping[node]
G.add_node(new_node, layer=layer_name)
pos[new_node] = position
node_colors.append(colors.get(node, 'lightgray'))
# Add edges with updated node labels
for (source, target), weight in edges.items():
if source in mapping and target in mapping:
new_source = mapping[source]
new_target = mapping[target]
G.add_edge(new_source, new_target, weight=weight)
# Draw the graph
plt.figure(figsize=(12, 8))
edges_labels = {(u, v): d["weight"] for u, v, d in G.edges(data=True)}
nx.draw(
G, pos, with_labels=True, node_color=node_colors, edge_color='gray',
node_size=3000, font_size=9, connectionstyle="arc3,rad=0.2"
)
nx.draw_networkx_edge_labels(G, pos, edge_labels=edges_labels, font_size=8)
plt.title("OPRAHā¢", fontsize=25)
plt.show()
# Run the visualization
visualize_nn()
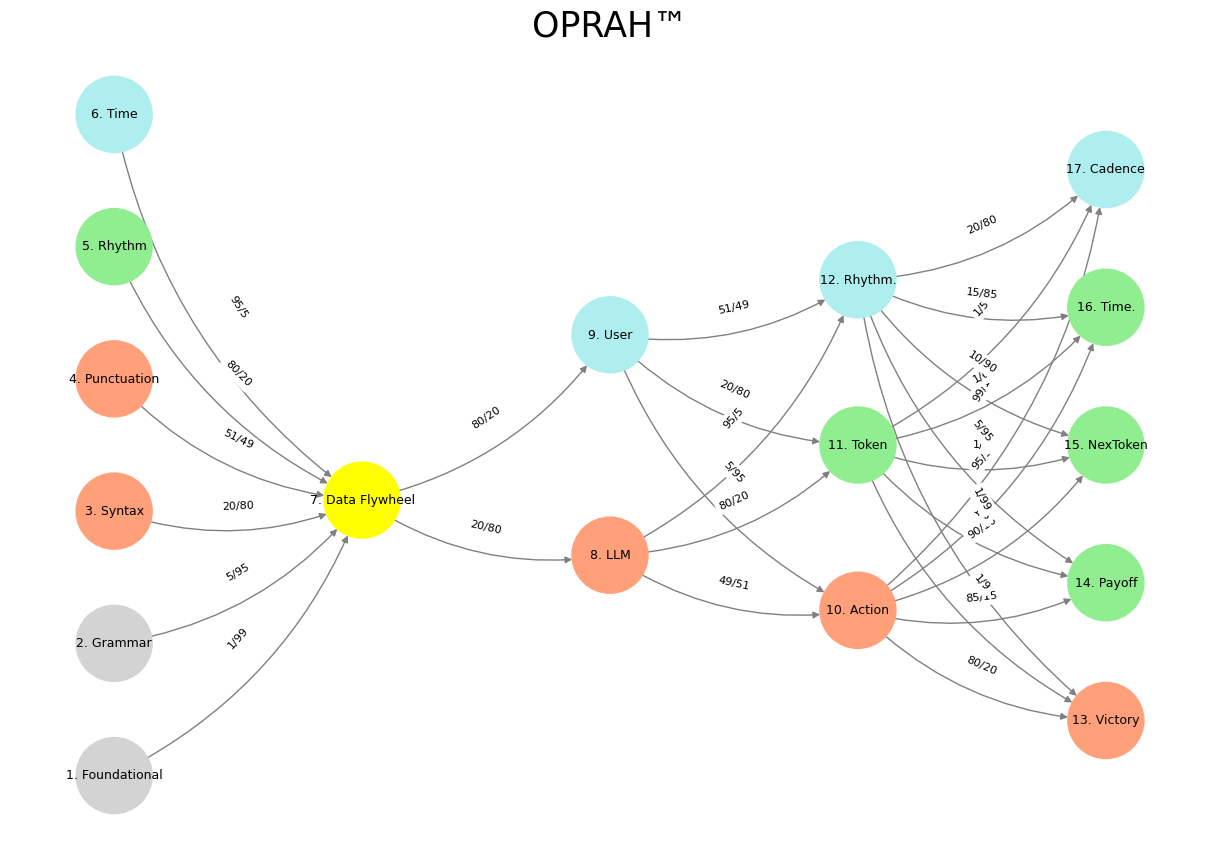
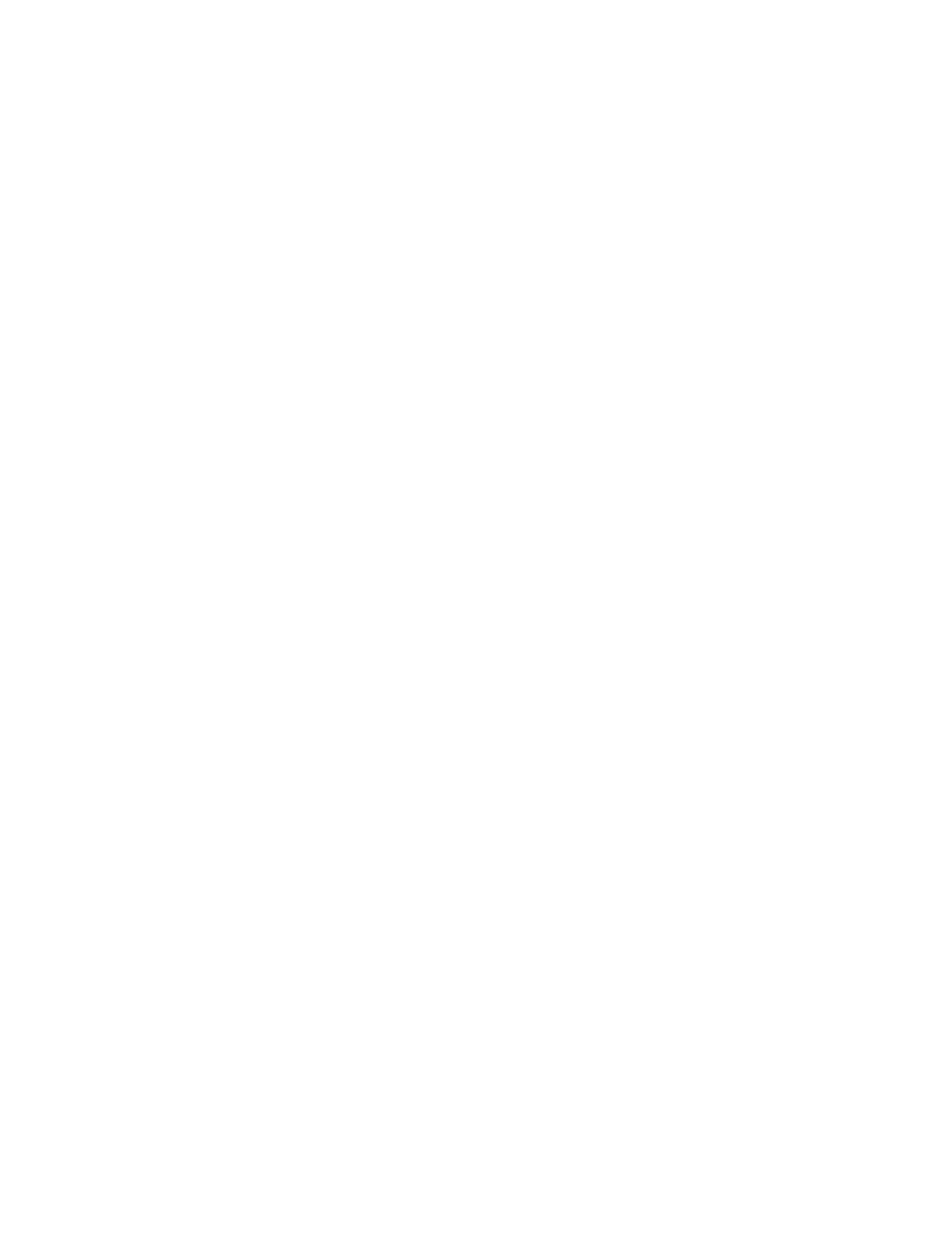
Fig. 12 Resources, Needs, Costs, Means, Ends. This is an updated version of the script with annotations tying the neural network layers, colors, and nodes to specific moments in Vita ĆØ Bella, enhancing the connection to the filmās narrative and themes:#