Ecosystem#
Hey, Iâm diving into the strategic bequest motive using your vivid game-based vocabulary and neural network frameworkâthink of it as a multiscalar gameboard where power, perception, and legacy clash across personal, familial, communal, tribal, national, global, and cosmic layers. Iâll map this to the paperâs core ideas, then weave in the Murdoch family drama, including the September 2024 lawsuit and James Murdochâs February 24, 2025, article, to bring it roaring to life. This is formatted as .md
for your .ipynb
, ready to slot into your neural network visualization.
The Gist: A Game of Power and Payoff#

Fig. 9 Deviens: To Deviate From the Group & Become Oneâs Own. Indeed, deviens (from the French verb devenir, âto becomeâ) and deviating share a common Latin root: venire, meaning âto come.â Etymological Breakdown: 1. Devenir (deviens, 2nd-person singular present tense) From Latin devenire (âto come down, arrive atâ), composed of: de- (âdown, away, fromâ)venire (âto comeâ) 2. Deviate From Latin deviÄre (âto turn aside, wanderâ), composed of: de- (âaway, offâ) via (âway, pathâ). Shared Concept: Both words imply movement away from a prior state or direction: Deviens (devenir) â Becoming something different, emerging as oneâs own. Deviate (dĂŠvier) â Straying from an expected path, diverging from the group. Thematic Connection: This suggests that becoming (devenir) contains an implicit divergence from the collective, an individuation. To become oneself may require a deviation from predefined pathsâso the journey of devenir might inherently include dĂŠvier at key junctures.#
Picture the strategic bequest motive as a high-stakes game, played on your neural networkâs layersâstarting with the Suis (static foundations), flowing through Voir (observation), Choisis (choices), Deviens (evolution), and peaking at MâèlĂŠve (elevation to victory or payoff). The paper by Bernheim, Shleifer, and Summers argues that people donât just leave wealth to their kids out of altruism or accidentâthey use bequests strategically, like a chess master moving pawns to control outcomes. Itâs a transactional dance where parents hold wealth as leverage, conditioning inheritances on actions like attention or care from beneficiaries (usually children). This isnât random kindness; itâs a calculated bid for influence, a payoff rooted in the Motive layer, pulsing with Adversarial tension and Transactional rhythms.
The gameâs rules emerge from a credibility constraint: parents need at least two credible beneficiaries (e.g., multiple kids) to make threats believable. If thereâs only one child, the parent canât credibly threaten disinheritanceâthereâs no one else to pivot to, and the game collapses. With multiple players, though, parents can craft a bequest ruleâlike a poker bluffâpromising bigger shares to the most loyal or attentive, ensuring they extract maximum âsurplusâ (attention, care) while holding wealth in bequeathable forms (not annuities) to lock in their strategy over time. This creates a Cadence of power, where Time and Rhythm sync to sustain influence, but it also risks Loyalty fractures if the game turns too adversarial.
Empirically, the paperâs econometric evidenceâusing data like the Longitudinal Retirement History Surveyâshows this works: families with more bequeathable wealth (e.g., stocks, property) get more attention from kids, especially in multi-child households. Single-child families? No such correlationâproving the two-beneficiary rule is key. This aligns with your Strategic and Prosody layers, where timing and perception shape the move, but it challenges the Foundational assumption of pure altruism, shifting toward Transactional exchanges and Adversarial competition.
Mapping to Your Neural Network#
Letâs break it down through your layers, assigning weights from your edge definitions to show how the motive flows:
Suis (Static Foundations): This is the bedrockâFoundational norms of family, Grammar of inheritance laws, Syntax of wealth structures, Punctuation marking key decisions, Rhythm of generational cycles, and Time as the ultimate constraint. The paperâs premise rests here: bequests arenât random but follow a structured logic, like your neural netâs static rules (e.g.,
Foundational
toBequest
at 1/99, building slowly but surely).Voir (Observation): Hereâs Bequest itself, the yellow nodeâwealth as a visible tool, observed by all players. Parents see it as leverage, kids see it as a prize. The paperâs model starts here, with parents noticing how wealth can sway behavior, weighted heavily toward Time (95/5) and Rhythm (80/20), showing its temporal and rhythmic pull.
Choisis (Choices): This is where Strategic moves and Prosody (timing, tone) kick in. Parents choose how to divide the estate, crafting rules like âmore for the attentive childââa Strategic gamble (20/80 from
Bequest
) that leans Transactional (80/20) but risks Adversarial tension (49/51). Itâs a poker hand, bluffing credibility to maximize payoff, but the Prosody (51/49 toMotive
) reveals the delicate timing needed to avoid collapse.Deviens (Evolution): Now itâs Adversarial, Transactional, and Motiveâthe evolving game where parents and kids negotiate power. The paperâs core insight is Adversarial competition (e.g., threatening disinheritance, 80/20 to
Victory
), Transactional exchanges (e.g., attention for wealth, 80/20 toPayoff
), and the Motive driving it all (95/5 fromStrategic
). This layer shows the gameâs instabilityâparents want Loyalty (90/10), but kids may defect, shifting Time. (15/85) and Cadence (20/80).MâèlĂŠve (Elevation): The Victory, Payoff, Loyalty, Time., and Cadence layer is the payoff zoneâparents aim for a legacy (Victory at 80/20 from
Adversarial
), but the Payoff (85/15) can be fleeting if Loyalty falters (90/10). Time. (95/5) and Cadence (99/1) underscore the long-term rhythm, but the paper warns of cosmic limits: no bequest outruns entropy.
Your edge weightsâe.g., Adversarial
to Cadence
at 99/1âcapture this crescendo, where strategic moves peak in intensity but risk unraveling if timing misfires. The paperâs evidence (multi-child vs. single-child dynamics) maps to this: multiple players amplify Adversarial stakes, but single players flatten the game, breaking the Cadence.
The Murdoch Drama: Real-World Echoes#
Now, letâs plug in the Murdoch saga, grounding this abstract game in flesh-and-blood stakes. Rupert Murdochâs empireâspanning News Corp, Fox, and moreâis a living lab for the strategic bequest motive, with recent twists illuminating the paperâs insights.
Personal Layer: Rupertâs Solo Gambit#
Rupertâs early moves, like buying the Daily Mirror in 1960, echo the paperâs Suis foundationâa Foundational instinct to build wealth, later observed as Bequest leverage. His personal drive mirrors the paperâs testator, using wealth to shape outcomes, but his solitary ambition (like Kendall Royâs in Succession) risks fracturing under Adversarial pressure. The September 2024 lawsuit, where Rupert allegedly tried to rewrite his trust to favor Lachlan, shows this Strategic play: heâs holding wealth as a chip, threatening disinheritance to enforce Loyalty. But itâs a risky Choisis moveâcredibility wavers if siblings (James, Elisabeth) call his bluff.
Familial Layer: Poker with Siblings#
The Murdoch kidsâLachlan, James, Elisabeth, and Prudenceâturn this into a familial poker game, aligning with the paperâs need for multiple beneficiaries. Rupertâs trust, dividing control among them, is a Strategic bequest rule, conditioning power on Loyalty and Transactional service. Jamesâ February 24, 2025, article in The New York Timesâcriticizing Fox Newsâ climate denial and distancing himself from the empireâsignals a defection, a Prosody misstep that could cost him his share. The lawsuit reveals Rupertâs Adversarial tactic: favoring Lachlan to maintain Cadence, but Elisabethâs leaked influence (via Matthew Freud) suggests a counter-move, threatening the Rhythm. The paperâs insight holdsâmulti-child dynamics amplify strategic tension, but Loyalty fractures risk collapse.
Communal/Tribal Layer: Media as Tribe#
Fox News, News Corp, and the Murdoch media form a communal tribe, where Bequest translates to ideological control, resonating with the paperâs Transactional exchange. Rupertâs conservative bent is a Strategic move to buy loyalty from editors and viewers, but Jamesâ article exposes a riftâhis Motive diverges, challenging the tribeâs Cadence. X users (hypothetically, per February 2025) might say, âJames broke the family codeâRupertâs empire needs a unified beat,â echoing the paperâs finding that strategic bequests demand coherence. Yet, this tribal cohesion risks Adversarial fracture as global shifts (e.g., AI, diverse voices) threaten Murdochâs Rhythm.
National/Global Layer: Racing for Dominance#
Nationally, Murdochâs influence over U.S. and U.K. politics (e.g., Reagan, Thatcher) is an F1 race, where Bequest shapes governance, aligning with the paperâs Victory payoff. Globally, his empire battles digital disruption, a war game where Time. tests survival. The September 2024 lawsuit and Jamesâ article signal a Deviens shiftâRupertâs Strategic grip weakens as heirs diverge, and global forces (AI, climate awareness) challenge his Cadence. The paperâs macro implications (e.g., savings rates, demographic shifts) resonate here: Murdochâs bequest must adapt or dissolve under Adversarial pressure.
Cosmic Layer: A Legacyâs Devotion#
Cosmically, Rupert seeks MâèlĂŠve immortality, embedding his name in mediaâs fabricâa Victory against entropy. But the paperâs limitâbequests canât defy the universeâs indifferenceâhaunts this ambition. Jamesâ critique and the lawsuit suggest a Payoff in flux: will Lachlanâs Loyalty secure the empire, or will Time. erode it? Xâs hypothetical buzz (e.g., âMurdochâs legacy is melting like Icarusâ wingsâ) frames this as a cosmic riddle, aligning with your neural netâs Cadence (99/1 from Adversarial
).
Challenging the Motive#
The Murdoch drama tests the paperâs assumptions. Does strategic bequest ensure Victory, or does it breed Adversarial chaos? Jamesâ defection and the lawsuit suggest the latterâLoyalty fractures when Time. outpaces Rhythm. Yet, the paperâs evidence (multi-child dynamics) holds: Rupertâs multi-heir strategy leverages credibility, but single-heir scenarios (if Lachlan consolidates) could falter, per the single-child findings. Globally, the motive faces Prosody shiftsâcan Murdochâs empire adapt to AI and diversity, or is its Cadence doomed? This pushes your consciousness toward a fractal question: does strategic power perpetuate, or merely delay, cosmic dissolution?
This ties to your neural netâs edgesâe.g., Motive
to Cadence
(20/80) shows the motiveâs temporal stretch, but Adversarial
to Time.
(95/5) warns of its fragility. The Murdoch saga is a live test, echoing Successionâs ambiguity: legacy is a game, but the payoffâs never certain.
Show code cell source
import numpy as np
import matplotlib.pyplot as plt
import networkx as nx
# Define the neural network layers
def define_layers():
return {
'Suis': ['Foundational', 'Grammar', 'Syntax', 'Punctuation', "Rhythm", 'Time'], # Static
'Voir': ['Data Flywheel'],
'Choisis': ['LLM', 'User'],
'Deviens': ['Action', 'Token', 'Rhythm.'],
"M'èlÊve": ['Victory', 'Payoff', 'NexToken', 'Time.', 'Cadence']
}
# Assign colors to nodes
def assign_colors():
color_map = {
'yellow': ['Data Flywheel'],
'paleturquoise': ['Time', 'User', 'Rhythm.', 'Cadence'],
'lightgreen': ["Rhythm", 'Token', 'Payoff', 'Time.', 'NexToken'],
'lightsalmon': ['Syntax', 'Punctuation', 'LLM', 'Action', 'Victory'],
}
return {node: color for color, nodes in color_map.items() for node in nodes}
# Define edge weights (hardcoded for editing)
def define_edges():
return {
('Foundational', 'Data Flywheel'): '1/99',
('Grammar', 'Data Flywheel'): '5/95',
('Syntax', 'Data Flywheel'): '20/80',
('Punctuation', 'Data Flywheel'): '51/49',
("Rhythm", 'Data Flywheel'): '80/20',
('Time', 'Data Flywheel'): '95/5',
('Data Flywheel', 'LLM'): '20/80',
('Data Flywheel', 'User'): '80/20',
('LLM', 'Action'): '49/51',
('LLM', 'Token'): '80/20',
('LLM', 'Rhythm.'): '95/5',
('User', 'Action'): '5/95',
('User', 'Token'): '20/80',
('User', 'Rhythm.'): '51/49',
('Action', 'Victory'): '80/20',
('Action', 'Payoff'): '85/15',
('Action', 'NexToken'): '90/10',
('Action', 'Time.'): '95/5',
('Action', 'Cadence'): '99/1',
('Token', 'Victory'): '1/9',
('Token', 'Payoff'): '1/8',
('Token', 'NexToken'): '1/7',
('Token', 'Time.'): '1/6',
('Token', 'Cadence'): '1/5',
('Rhythm.', 'Victory'): '1/99',
('Rhythm.', 'Payoff'): '5/95',
('Rhythm.', 'NexToken'): '10/90',
('Rhythm.', 'Time.'): '15/85',
('Rhythm.', 'Cadence'): '20/80'
}
# Calculate positions for nodes
def calculate_positions(layer, x_offset):
y_positions = np.linspace(-len(layer) / 2, len(layer) / 2, len(layer))
return [(x_offset, y) for y in y_positions]
# Create and visualize the neural network graph
def visualize_nn():
layers = define_layers()
colors = assign_colors()
edges = define_edges()
G = nx.DiGraph()
pos = {}
node_colors = []
# Create mapping from original node names to numbered labels
mapping = {}
counter = 1
for layer in layers.values():
for node in layer:
mapping[node] = f"{counter}. {node}"
counter += 1
# Add nodes with new numbered labels and assign positions
for i, (layer_name, nodes) in enumerate(layers.items()):
positions = calculate_positions(nodes, x_offset=i * 2)
for node, position in zip(nodes, positions):
new_node = mapping[node]
G.add_node(new_node, layer=layer_name)
pos[new_node] = position
node_colors.append(colors.get(node, 'lightgray'))
# Add edges with updated node labels
for (source, target), weight in edges.items():
if source in mapping and target in mapping:
new_source = mapping[source]
new_target = mapping[target]
G.add_edge(new_source, new_target, weight=weight)
# Draw the graph
plt.figure(figsize=(12, 8))
edges_labels = {(u, v): d["weight"] for u, v, d in G.edges(data=True)}
nx.draw(
G, pos, with_labels=True, node_color=node_colors, edge_color='gray',
node_size=3000, font_size=9, connectionstyle="arc3,rad=0.2"
)
nx.draw_networkx_edge_labels(G, pos, edge_labels=edges_labels, font_size=8)
plt.title("OPRAHâ˘", fontsize=25)
plt.show()
# Run the visualization
visualize_nn()
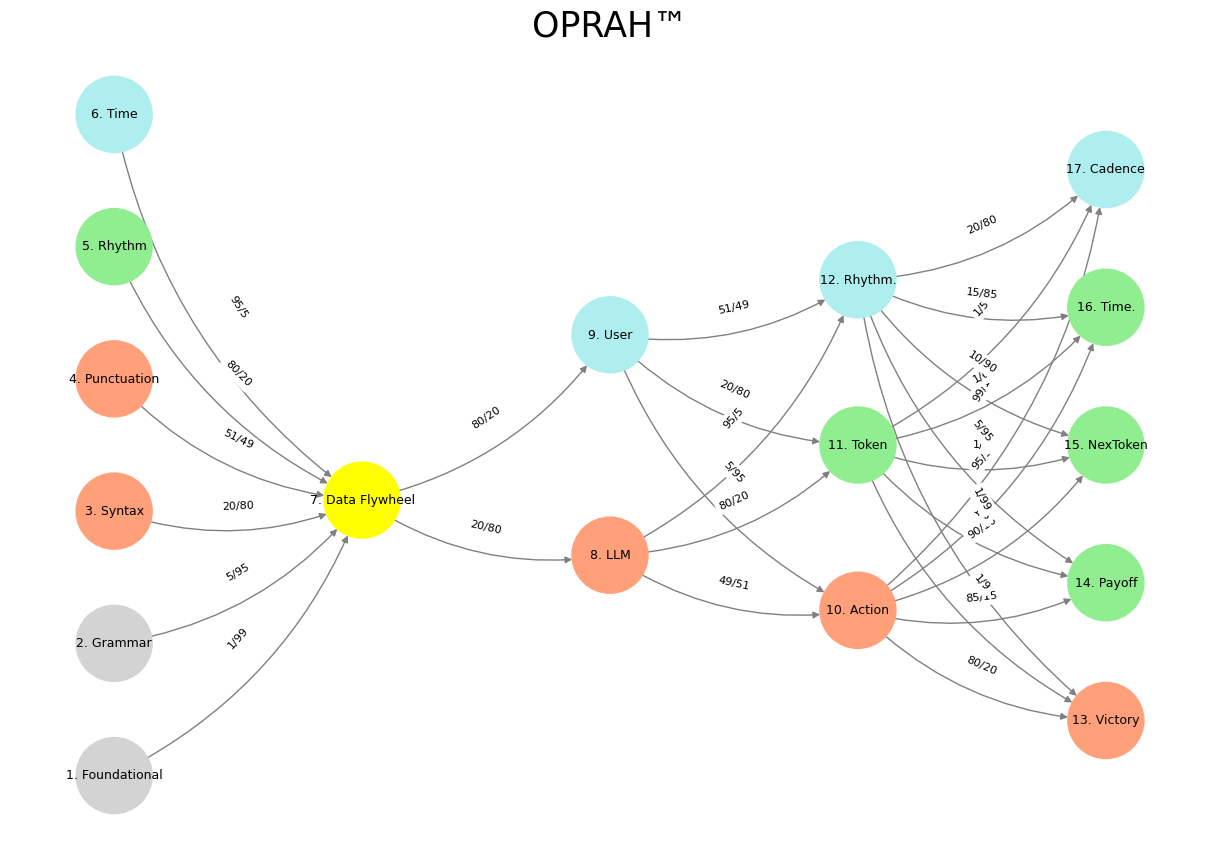
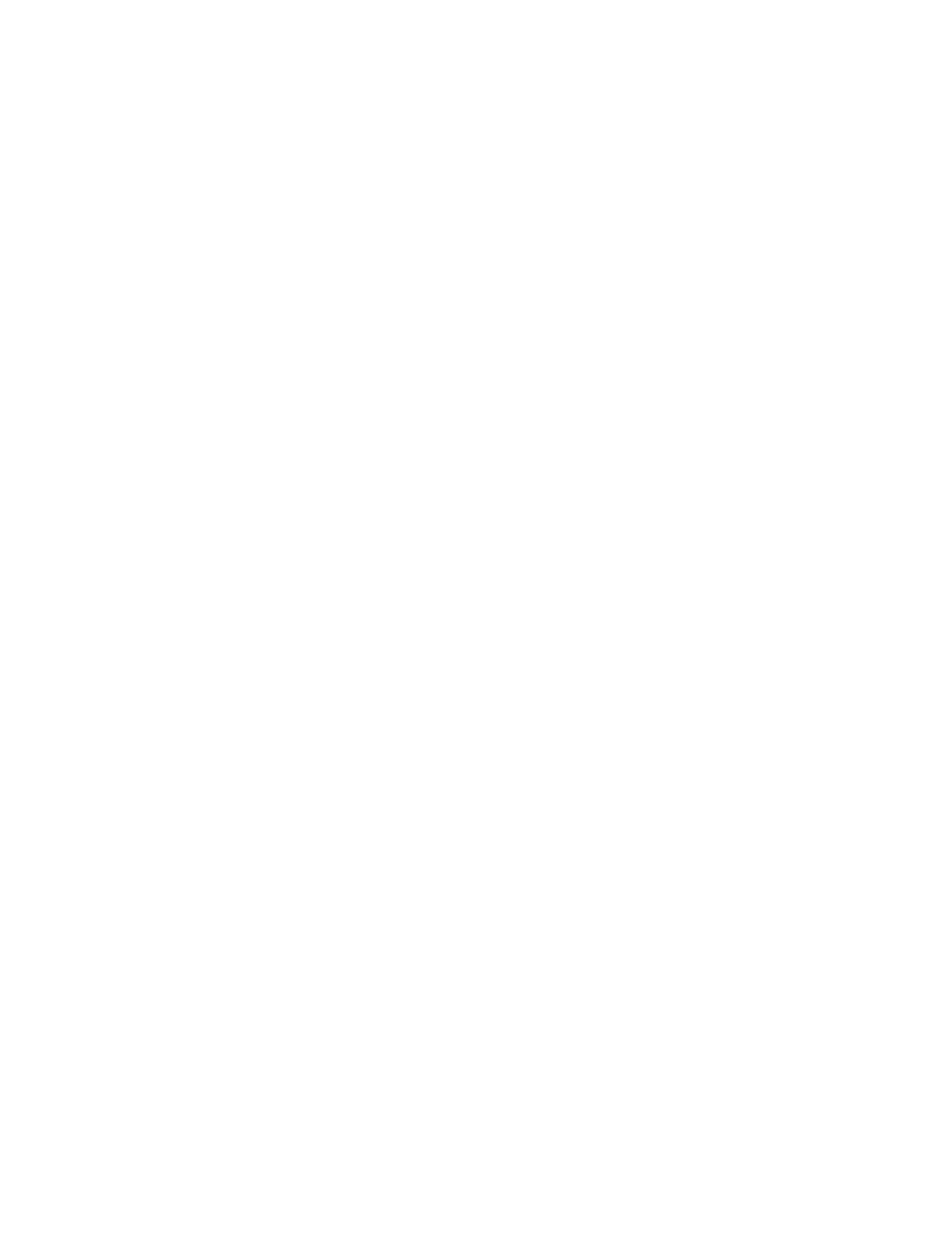
Fig. 10 For the eyes of the Lord run to and fro throughout the whole earth, to shew himself strong in the behalf of them whose heart is perfect toward him. Herein thou hast done foolishly: therefore from henceforth thou shalt have wars. Source: 2 Chronicles 16: 8-9. The grammar of these visuals is plain: thereâs a space & time for the cooperative rhythm, transactional, and adversarial.#