Dancing in Chains#
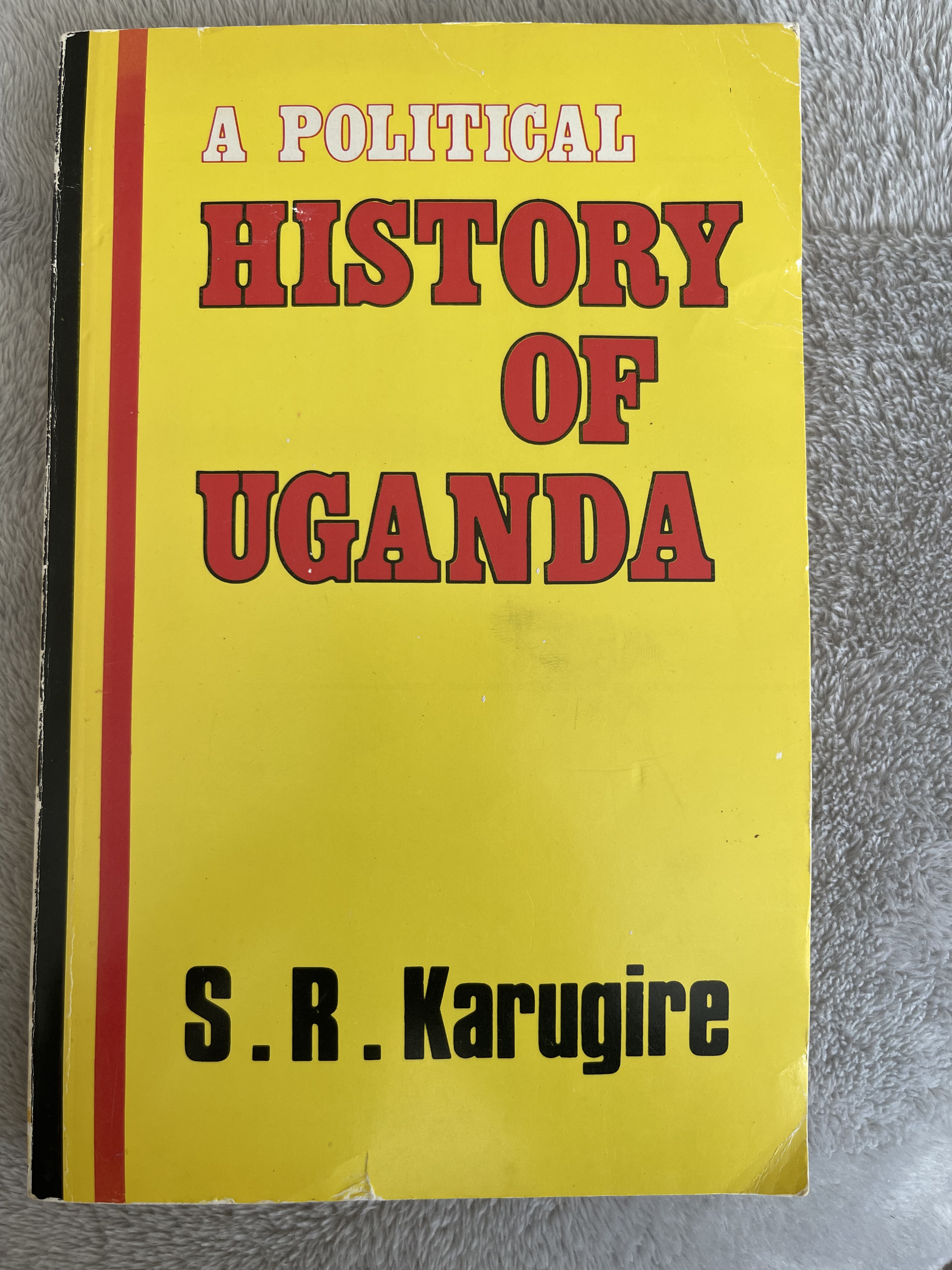
Uganda's postcolonial history is a fractal unfolding of the aesthetic imperative vs. moral imposition: really a question of agency!
Dear Alien, although you're not our brother: are you friend or foe (or other)?
Show code cell source
import numpy as np
import matplotlib.pyplot as plt
import networkx as nx
# Define the neural network layers
def define_layers():
return {
'Suis': ['Mr. Jones, 5%', 'Pigs Doctrine', "Major's Vision", 'Beasts of England', "Napoleons Strategy", 'Snowball Plan'],
'Voir': ['Rebellion, 20%'],
'Choisis': ['Napoleon Enforcer, 50%', 'Snowball Idealist'],
'Deviens': ['Exhaution of Animals', 'Dogs, Loyal Enforcers', 'Squealors Propaganda, 20%'],
"M'รจlรฉve": ['Final Oppression', 'Surveillance', 'Indoctrination', 'Absolute Rule, 5%', 'New Hierarchy']
}
# Assign colors to nodes
def assign_colors():
color_map = {
'yellow': ['Rebellion, 20%'],
'paleturquoise': ['Snowball Plan', 'Snowball Idealist', 'Squealors Propaganda, 20%', 'New Hierarchy'],
'lightgreen': ["Napoleons Strategy", 'Dogs, Loyal Enforcers', 'Surveillance', 'Absolute Rule, 5%', 'Indoctrination'],
'lightsalmon': ["Major's Vision", 'Beasts of England', 'Napoleon Enforcer, 50%', 'Exhaution of Animals', 'Final Oppression'],
}
return {node: color for color, nodes in color_map.items() for node in nodes}
# Define edge weights
def define_edges():
return {
('Mr. Jones, 5%', 'Rebellion, 20%'): '1/99',
('Pigs Doctrine', 'Rebellion, 20%'): '5/95',
("Major's Vision", 'Rebellion, 20%'): '20/80',
('Beasts of England', 'Rebellion, 20%'): '51/49',
("Napoleons Strategy", 'Rebellion, 20%'): '80/20',
('Snowball Plan', 'Rebellion, 20%'): '95/5',
('Rebellion, 20%', 'Napoleon Enforcer, 50%'): '20/80',
('Rebellion, 20%', 'Snowball Idealist'): '80/20',
('Napoleon Enforcer, 50%', 'Exhaution of Animals'): '49/51',
('Napoleon Enforcer, 50%', 'Dogs, Loyal Enforcers'): '80/20',
('Napoleon Enforcer, 50%', 'Squealors Propaganda, 20%'): '95/5',
('Snowball Idealist', 'Exhaution of Animals'): '5/95',
('Snowball Idealist', 'Dogs, Loyal Enforcers'): '20/80',
('Snowball Idealist', 'Squealors Propaganda, 20%'): '51/49',
('Exhaution of Animals', 'Final Oppression'): '80/20',
('Exhaution of Animals', 'Surveillance'): '85/15',
('Exhaution of Animals', 'Indoctrination'): '90/10',
('Exhaution of Animals', 'Absolute Rule, 5%'): '95/5',
('Exhaution of Animals', 'New Hierarchy'): '99/1',
('Dogs, Loyal Enforcers', 'Final Oppression'): '1/9',
('Dogs, Loyal Enforcers', 'Surveillance'): '1/8',
('Dogs, Loyal Enforcers', 'Indoctrination'): '1/7',
('Dogs, Loyal Enforcers', 'Absolute Rule, 5%'): '1/6',
('Dogs, Loyal Enforcers', 'New Hierarchy'): '1/5',
('Squealors Propaganda, 20%', 'Final Oppression'): '1/99',
('Squealors Propaganda, 20%', 'Surveillance'): '5/95',
('Squealors Propaganda, 20%', 'Indoctrination'): '10/90',
('Squealors Propaganda, 20%', 'Absolute Rule, 5%'): '15/85',
('Squealors Propaganda, 20%', 'New Hierarchy'): '20/80'
}
# Define edges to be highlighted in black
def define_black_edges():
return {
('Mr. Jones, 5%', 'Rebellion, 20%'): '1/99',
('Pigs Doctrine', 'Rebellion, 20%'): '5/95',
}
# Calculate node positions
def calculate_positions(layer, x_offset):
y_positions = np.linspace(-len(layer) / 2, len(layer) / 2, len(layer))
return [(x_offset, y) for y in y_positions]
# Create and visualize the neural network graph
def visualize_nn():
layers = define_layers()
colors = assign_colors()
edges = define_edges()
black_edges = define_black_edges()
G = nx.DiGraph()
pos = {}
node_colors = []
# Create mapping from original node names to numbered labels
mapping = {}
counter = 1
for layer in layers.values():
for node in layer:
mapping[node] = f"{counter}. {node}"
counter += 1
# Add nodes with new numbered labels and assign positions
for i, (layer_name, nodes) in enumerate(layers.items()):
positions = calculate_positions(nodes, x_offset=i * 2)
for node, position in zip(nodes, positions):
new_node = mapping[node]
G.add_node(new_node, layer=layer_name)
pos[new_node] = position
node_colors.append(colors.get(node, 'lightgray'))
# Add edges with updated node labels
edge_colors = []
for (source, target), weight in edges.items():
if source in mapping and target in mapping:
new_source = mapping[source]
new_target = mapping[target]
G.add_edge(new_source, new_target, weight=weight)
edge_colors.append('black' if (source, target) in black_edges else 'lightgrey')
# Draw the graph
plt.figure(figsize=(12, 8))
edges_labels = {(u, v): d["weight"] for u, v, d in G.edges(data=True)}
nx.draw(
G, pos, with_labels=True, node_color=node_colors, edge_color=edge_colors,
node_size=3000, font_size=9, connectionstyle="arc3,rad=0.2"
)
nx.draw_networkx_edge_labels(G, pos, edge_labels=edges_labels, font_size=8)
plt.title("Animal Farm", fontsize=18)
plt.show()
# Run the visualization
visualize_nn()
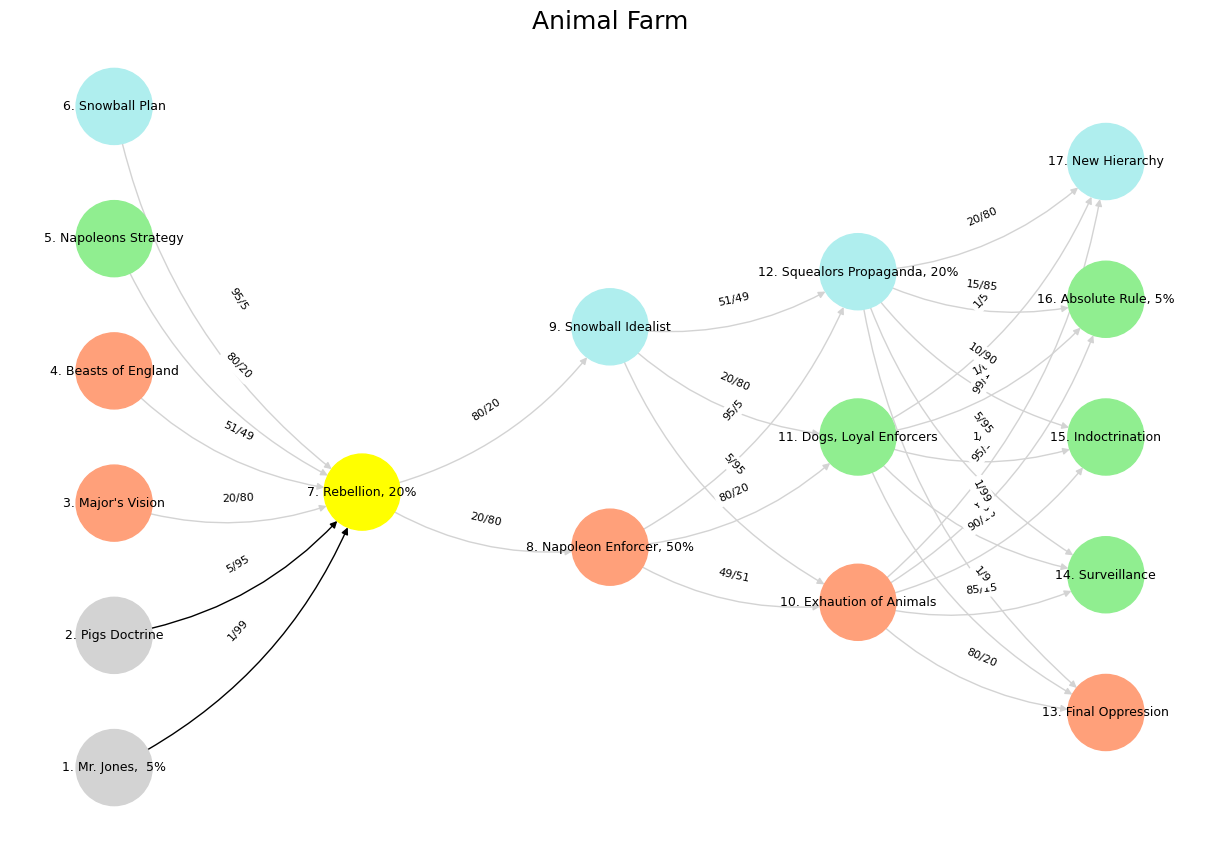
Fig. 33 Does our narrative herein transcent agent-space-time? How does it with a Tsar in 20th century Russia?#