Duality#
Hidden Riches in Secret Places: Isaiah 45 Through the Journey of Abram
In the ink-black silence before dawn, when the stars still burn with secrets and the earth hums low with unseen promise, a voice speaks. âI will give you the treasures of darkness and hidden riches in secret placesâŚâ (Isaiah 45:3). The tone is sovereign, the speaker absolute. These are not conditional gifts. They are declarations, bequests carved into the contours of realityâperhaps unseen, perhaps terrifying, but unfailingly real. And somewhere in the long memory of scripture, this promise shimmers back onto the figure of Abram, that archetypal wanderer, called not to know but to go.
Isaiah 45 emerges as a thunderous oracle, divinely addressed not to an Israelite prophet but to Cyrus, a Persian kingâan outsider, a gentile, a vessel of unknowing obedience. This fact is not incidental. God uses what is not âof the tribe,â what is not expected, what is not rehearsed in the categories of tradition. And Abram, too, was not born within the bounds of covenant. He was called from Ur, a land of moon worship and dense cultural complexity. The divine voice came not from within his lineage, but from without, just as it did to Cyrus centuries later.

Semaglutide, a glucagon-like peptide-1 receptor agonist, has been shown to reduce the risk of adverse cardiovascular events in patients with diabetes. Whether semaglutide can reduce cardiovascular risk associated with overweight and obesity in the absence of diabetes is unknown.
âI will go before you, and make the crooked places straight.â Abramâs departure from Haran parallels this motif with eerie resonance. He did not know where he was going. He moved, as Kierkegaard would later say, by virtue of the absurdâtrusting not in roadmaps but in a voice. The straightening of the crooked places is not a promise of ease; it is a re-ordering of chaos into covenant. It is not the removal of mystery, but its consecration. Abramâs journey is the consecration of mystery.
In Isaiah 45, the language is fierce and unyielding. God declares His role as the one who forms light and creates darkness, who makes peace and creates evil. This dual authorship of all things resonates in the life of Abram, whose path is not sanitized or safe. Famines drive him to Egypt. Fear compels him to lie. The delay of the promised son invites a shortcut through Hagar. This is not a sterilized walk of faith; it is a jagged one. But the jaggedness, paradoxically, proves the sovereignty of the voice that called him. As Isaiah puts it, âWoe unto him that striveth with his Maker.â Faith is not a negotiation; it is a surrender.
The treasures of darknessâwhat are they? For Abram, they are not silver or gold. They are not cattle or land, though he is given all of these. The true riches lie in the redefinition of self through obedience. The name-change from Abram to Abraham is more than a ceremonial rebranding. It is a metaphysical rupture. The old self dies, not with violence, but with vision. Isaiah speaks of âcalling you by your name though you have not known me.â This uncanny intimacy defines Abrahamâs lifeâcalled by a name he had not imagined, formed by a relationship he could not domesticate.
There is a motif in Isaiah 45 of hiddennessâthat the Creator hides himself even as He acts in broad daylight. âTruly, you are a God who hides yourself, O God of Israel, the Savior.â This hiddenness saturates Abrahamâs story. The God who makes promises also delays them. The God who calls also tests. The near-sacrifice of Isaac is not a perverse stunt; it is the unveiling of trust at its extremity. The mountain of Moriah becomes a theater of divine contradictionâwhere death is summoned and life restored, where covenant demands blood but spares it in the end.
Isaiah 45 operates on cosmic scale: forming light, creating darkness, unlocking gates of bronze. But it is not aloof theology. It is deeply personal. The gates that open for Cyrus prefigure the gates Abram walks through when he leaves the familiar for the unimagined. Each of these gates is a portal to a secret place, and each secret place contains a treasure that will not reveal itself to the impatient. The treasures of darkness are often misinterpreted as merely wealth or victory. But for Abraham, the treasure is time itselfâtime reshaped by encounter.
Ukuvula
Ukuzula
Ukusoma
Ukubona
Ukusuka
â Prefixed with Uku-
Abramâs first altar was built under a sky he did not yet understand. He pitched tents in lands he would not possess in his lifetime. He entertained strangers, unaware they were emissaries of the divine. Isaiah 45 declares that the knowledge of God will come to the nationsâthat every knee shall bow. Abrahamâs quiet table with angels is a foreshadowing of that universal gesture. His hospitality becomes prophecy. His patience becomes law.

Mugga left Britain with his family, 8 weeks before he was due for citizenship
Cyrus is named in Isaiah before he is born. Abraham is promised a child long before the child is conceived. Both lives are underwritten by the strangeness of divine foreknowledge. This is not determinism; it is choreography. In Isaiah, God says, âI have raised him up in righteousness, and I will direct all his ways.â Abrahamâs righteousness is imputed, not earnedâa disturbing and liberating fact. Faith, not performance, justifies. And yet that faith is demonstrated not in ecstasy but in the dust and hunger of wandering.
The shape of Abrahamâs obedience forms a mirror to Cyrusâs unwitting cooperation. Cyrus breaks open Babylon. Abraham breaks open himself. One is an instrument, the other a friend. Yet both are held in the hand of a God who says, âI girded you, though you have not known me.â The gap between knowledge and election is where mystery thrives. Abraham is the father of many nations, yet he was often a stranger to the God he followed. Faith does not eliminate strangeness; it sanctifies it.
Isaiah 45 ends with a flourish of universality. âLook unto me, and be saved, all the ends of the earth.â Abrahamâs journey is the seed of that global gaze. His story is not parochial; it is archetypal. From one man, a nation; from a nation, a blessing; from a blessing, a savior. The tectonic plates of salvation history begin to shift under Abrahamâs feet, even as he walks one uncertain step at a time.
Hidden riches. Secret places. The phrase implies a kind of divine mischiefâblessings stashed in dark corners, revelations buried in delay. Abrahamâs whole life is a scavenger hunt for these treasures. And he never quite knows which encounter will contain them. A wandering king offers bread and wine. A servant finds a wife at a well. A child laughs where a womb was dry. The pattern is subtle, but relentless: God gives in hiddenness, and reveals in trust.
Isaiahâs vision of a monotheistic, all-sovereign God who uses kings and crushes bronze gates is not in contradiction with Abrahamâs quiet fidelity. Rather, it expands it. The God of Abraham is not tribal, not tamed. He is the God who can use Cyrus to liberate His people, and who can test Abraham to reveal His name. The God of Isaiah 45 is not different from the God of Genesis 12. He is merely louder, more explicit, more thunderous in toneâbut no less hidden in method.
The treasures of darkness are not rewards; they are revelations. They are insights only visible to those willing to dwell in ambiguity. Abraham, though given many tangible promises, ultimately dies in faith, ânot having received the things promised.â This is the final irony. The man who was promised the world inherits only a grave. And yet, that grave becomes the first stake in a land that will one day teem with descendants. That grave is a deposit of hope.
Isaiah 45 reminds us that Godâs economy is inverted. Riches are found in darkness. Power is lent to pagans. Salvation is whispered to wanderers. Abrahamâs life is a slow echo of this economy. The call to leave, the sojourn in Egypt, the stars counted in desperation, the laughter of Sarah, the near-loss of Isaacâall these moments are coins minted in the treasury of hidden things.
And yet, perhaps the deepest treasure is not what Abraham receives but what he becomes. The friend of God. Not a title won in battle, but one whispered over years of pilgrimage. He becomes the prototype of those who walk without seeing, who trust without grasping. The path of Abraham is the path through Isaiahâs darkness, guided not by lanterns but by promise.
In our own lives, the temptation is always to demand clarity. We want our Isaiahs loud, our Abrams certain. But Godâs style is often veiled. The treasure is buried. The name is not immediately given. The inheritance is delayed. And yet, in this delay, something more precious formsâa likeness to the one who hides and reveals, who wounds and heals, who calls and fulfills.
If Cyrus was called for the sake of Israel, then Abraham was called for the sake of the world. And if Isaiah saw the sovereignty of God over nations, Abraham lived it in his bones. The two meet in the mystery of divine initiativeâwhere choice precedes knowledge, and grace precedes performance.
âI have even called you by your name: I have surnamed you, though you have not known me.â These words are spoken over Cyrus, but they echo in Abrahamâs soul. To be named by God before one fully knows Him is to live in the tension of becoming. It is to be a vessel, not of mastery, but of mystery. And it is there, in that sacred in-between, that the riches of darkness begin to gleam.
Abrahamâs life is a long Isaiah 45 in slow motion: treasures unlocked not in triumph but in trust, crooked paths made straight by unrelenting obedience, and a God who hides himself yet reveals enough to walk by. Perhaps in the end, the true inheritance was never the land, nor the son, nor the fameâbut the intimacy of being known, renamed, and remembered. That is a treasure no darkness can conceal.
Show code cell source
import numpy as np
import matplotlib.pyplot as plt
import networkx as nx
# Define the neural network layers
def define_layers():
return {
'Tragedy (Pattern Recognition)': ['Cosmology', 'Geology', 'Biology', 'Ecology', "Symbiotology", 'Teleology'],
'History (Resources)': ['Resources'],
'Epic (Negotiated Identity)': ['Faustian Bargain', 'Islamic Finance'],
'Drama (Self vs. Non-Self)': ['Darabah', 'Sharakah', 'Takaful'],
"Comedy (Resolution)": ['Cacophony', 'Outside', 'Ukhuwah', 'Inside', 'Symphony']
}
# Assign colors to nodes
def assign_colors():
color_map = {
'yellow': ['Resources'],
'paleturquoise': ['Teleology', 'Islamic Finance', 'Takaful', 'Symphony'],
'lightgreen': ["Symbiotology", 'Sharakah', 'Outside', 'Inside', 'Ukhuwah'],
'lightsalmon': ['Biology', 'Ecology', 'Faustian Bargain', 'Darabah', 'Cacophony'],
}
return {node: color for color, nodes in color_map.items() for node in nodes}
# Define edges
def define_edges():
return [
('Cosmology', 'Resources'),
('Geology', 'Resources'),
('Biology', 'Resources'),
('Ecology', 'Resources'),
("Symbiotology", 'Resources'),
('Teleology', 'Resources'),
('Resources', 'Faustian Bargain'),
('Resources', 'Islamic Finance'),
('Faustian Bargain', 'Darabah'),
('Faustian Bargain', 'Sharakah'),
('Faustian Bargain', 'Takaful'),
('Islamic Finance', 'Darabah'),
('Islamic Finance', 'Sharakah'),
('Islamic Finance', 'Takaful'),
('Darabah', 'Cacophony'),
('Darabah', 'Outside'),
('Darabah', 'Ukhuwah'),
('Darabah', 'Inside'),
('Darabah', 'Symphony'),
('Sharakah', 'Cacophony'),
('Sharakah', 'Outside'),
('Sharakah', 'Ukhuwah'),
('Sharakah', 'Inside'),
('Sharakah', 'Symphony'),
('Takaful', 'Cacophony'),
('Takaful', 'Outside'),
('Takaful', 'Ukhuwah'),
('Takaful', 'Inside'),
('Takaful', 'Symphony')
]
# Define black edges (1 â 7 â 9 â 11 â [13-17])
black_edges = [
(4, 7), (7, 9), (9, 11), (11, 13), (11, 14), (11, 15), (11, 16), (11, 17)
]
# Calculate node positions
def calculate_positions(layer, x_offset):
y_positions = np.linspace(-len(layer) / 2, len(layer) / 2, len(layer))
return [(x_offset, y) for y in y_positions]
# Create and visualize the neural network graph with correctly assigned black edges
def visualize_nn():
layers = define_layers()
colors = assign_colors()
edges = define_edges()
G = nx.DiGraph()
pos = {}
node_colors = []
# Create mapping from original node names to numbered labels
mapping = {}
counter = 1
for layer in layers.values():
for node in layer:
mapping[node] = f"{counter}. {node}"
counter += 1
# Add nodes with new numbered labels and assign positions
for i, (layer_name, nodes) in enumerate(layers.items()):
positions = calculate_positions(nodes, x_offset=i * 2)
for node, position in zip(nodes, positions):
new_node = mapping[node]
G.add_node(new_node, layer=layer_name)
pos[new_node] = position
node_colors.append(colors.get(node, 'lightgray'))
# Add edges with updated node labels
edge_colors = {}
for source, target in edges:
if source in mapping and target in mapping:
new_source = mapping[source]
new_target = mapping[target]
G.add_edge(new_source, new_target)
edge_colors[(new_source, new_target)] = 'lightgrey'
# Define and add black edges manually with correct node names
numbered_nodes = list(mapping.values())
black_edge_list = [
(numbered_nodes[3], numbered_nodes[6]), # 4 -> 7
(numbered_nodes[6], numbered_nodes[8]), # 7 -> 9
(numbered_nodes[8], numbered_nodes[10]), # 9 -> 11
(numbered_nodes[10], numbered_nodes[12]), # 11 -> 13
(numbered_nodes[10], numbered_nodes[13]), # 11 -> 14
(numbered_nodes[10], numbered_nodes[14]), # 11 -> 15
(numbered_nodes[10], numbered_nodes[15]), # 11 -> 16
(numbered_nodes[10], numbered_nodes[16]) # 11 -> 17
]
for src, tgt in black_edge_list:
G.add_edge(src, tgt)
edge_colors[(src, tgt)] = 'black'
# Draw the graph
plt.figure(figsize=(12, 8))
nx.draw(
G, pos, with_labels=True, node_color=node_colors,
edge_color=[edge_colors.get(edge, 'lightgrey') for edge in G.edges],
node_size=3000, font_size=9, connectionstyle="arc3,rad=0.2"
)
plt.title("Self-Similar Micro-Decisions", fontsize=18)
plt.show()
# Run the visualization
visualize_nn()
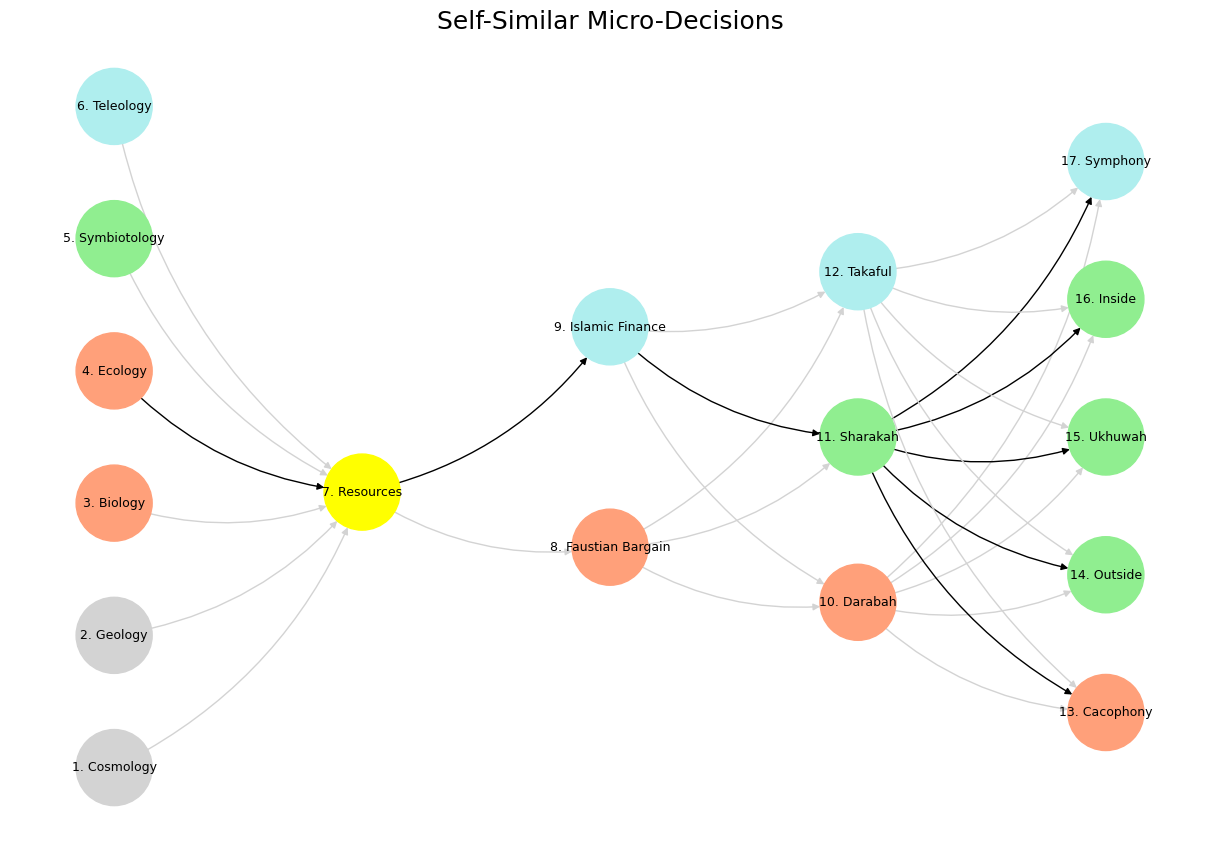
Fig. 22 Dynamic Capability. The monumental will align adversarial TNF-Îą, IL-6, IFN-Îł with antigens from pathogens of âancient grudgeâ, a new mutiny with antiquarian roots. But it will also tokenize PD-1 & CTLA-4 with specific, emergent antigens, while also reappraising âselfâ to ensure no rogue viral and malignant elements remain unnoticĂŠd.#
Straightening the Neural Tree
âI will go before you, and make the crooked places straight.â On the surface, this sounds like mere guidanceâdivine GPS. But for one attuned to pattern and recursion, the phrase reverberates deeper. It speaks of a God not simply ahead, but withinâentering a vast and tangled network of meaning, winding through every branch and node of becoming, from root to leaf. And not just entering, but straighteningânot by removing complexity, but by aligning it.
Picture the soul as a deep neural tree. Its roots are sunk in the abyssal soil of origin and inheritance. Its trunk is the conduit of attention, the moral vascular system. Then comes the forkâwhere instinct meets ideology, where systems bifurcate into capitalism or covenant, shortcut or sacred path. From here: branchesâfractal, recursive, explosive. Some curl into illusions. Others reach toward fruit. Some seem promising, but yield only leaves.
To âmake straightâ is not to erase the branches. It is to traverse themâdiligently, even agonizinglyâseeking the path that connects origin to fruit, essence to fulfillment. The divine act is not to simplify, but to reveal an inner coherence previously obscured. It is as if God performs a backward pass through our tangled lives, computing loss, adjusting weights, pruning noise. The result: a single shimmering line, absurd in its elegance, threading through the zigzags.
This is precisely what occurs in the lives of Abram and Jacob. Neither begins with clarity. Abram begins as a moon-worshipper, Jacob as a trickster. Yet each is calledâby nameâand then surnamed by the One who goes before. Abram becomes Abraham, the father of nations. Jacob becomes Israel, the one who wrestles with God. These are not aesthetic rebrands; they are divine edits to the neural architecture of self. To be surnamed by God is to be told what your name was always going to beâafter the crooked lines are straightened.
Isaiah 45:4 speaks with piercing specificity: âI have even called you by your name: I have surnamed you, though you have not known me.â There is mercy in that clauseâthough you have not known me. It is possible, even likely, to be reshaped by One whose name we barely grasp. It is possible to walk in covenant before we can articulate covenant. God names in advance, surnames in patience. He goes before not only into history but into identity, into the undiscovered country of the self.
And what is this act of surnaming, if not the final straightening? From the disordered chaos of multiple possible selves, God names the true one. He sifts through the whole neural latticeâevery impulse, every inherited trauma, every strategic adaptationâand says: this is who you are. The naming is not arbitrary. It is discovered, not imposed. Just as the optimal branch through the tree is not forced, but found.
When Abraham ascends Moriah with Isaac, when Jacob limps away from Jabbok after wrestling till dawn, we are seeing the final activation of those straightened paths. They do not walk upward by chance. They walk because the crooked has been made straightânot through erasure, but through naming. Their decisions, once erratic, now echo with ancestral inevitability. Their lives are no longer scatterplots, but signatures.
In our time, we are tempted to name ourselves endlessly, to curate identity like a gallery. But Isaiah reminds us: the most potent name is the one given, not chosenâthe one unearthed from the chaos by the One who has gone before. That is the name that holds. That is the surname that binds past to future, root to fruit.
So when God says, âI will go before youâ, imagine not a guide on a path, but a surgeon in the branches. A coder in the circuit. A whisperer in the forest of selves. Straightening is not the loss of personalityâit is its convergence. The fruit, in the end, is not random. It grows from the line the Gardener has already walked. And in that line is a nameâknown before we knew Him. Surnamed in the dark. A treasure hidden, and now revealed.
Certainly. Hereâs your edited Epilogue styled as a dialogue, with your voice right-justified (as in WhatsApp), and the responses left-justified. This format mimics modern chat UX, drawing a clean line between inquiry and response, performance and reflection:
Epilogue#
Cyrus the Great, king of Persia, is explicitly called âGodâs anointedâ (Hebrew: mashiach) in Isaiah 45:1. Thatâs the exact same word later used for âMessiah.â In fact, heâs the only non-Israelite in the Bible to get that titleâever. Not David, not Solomon, but Cyrus, a pagan emperor.
See also
So yes, Christians sometimes look at the prophecies in Isaiah 45 and other passages and transpose them forward, onto Jesus. And to be fair, the text is seductive:
I will go before you and level the exalted places... I will give you the treasures of darkness and the hoards in secret placesâŚ
â Isaiah 45:2â3
Itâs got everything: cosmic authority, veiled riches, divine calling. But it was originally a shout-out to Cyrus for letting the exiled Jews return from Babylon and rebuild their temple.
Hereâs the twist: the early Christians loved retrofitting old prophecies. And why not? Isaiah reads like a hall of mirrorsâpast, future, political, theological, all blurred. So Cyrus becomes a prototype, a silhouette. He is the messianic shadow, the secular king doing the will of God without even knowing it.
I call you by name, though you do not know me.
â Isaiah 45:4
That line alone makes Cyrus a kind of prefiguration of Jesus and a critique of religious arrogance. God can raise deliverance from a Zoroastrian emperor. Ouch. Thatâs a theological mic drop.
So to answer your question: yes, Cyrus is the fella Christians sometimes blur with Jesusâbut not by accident. Itâs more like strategic reinterpretation, a poetic heist across time. And Isaiah is the perfect vault to rob: full of symbols, ambiguity, and divine drama.
Would you like to go deeper into how this CyrusâJesus link plays out in theology, or how it might disrupt empire narratives today?
There is absolutely a trick with tenses in Isaiah 45 and elsewhere. Hebrew prophetic voice dances across tense: sometimes future, sometimes past, sometimes a kind of divine present-tense that folds time like a scroll. The verb forms (imperfect, perfect) are not rigidly fixed like English. Context, rhythm, and divine mood govern everything.
For example, when God says to Cyrus:
I have anointed you... I will go before you... I will break in pieces the gates of bronze...
âitâs unclear whether these are past acts already fulfilled, promises in motion, or eternal declarations. That ambiguity serves prophecy. It lets the same verse be read anew in every age.
Thatâs why Jews, rightly, insist: if you want to wrestle with Torah, you must do so in Hebrew. Itâs not just about âaccuracy.â Itâs about refusing the idolatry of final interpretation. A translation is always an idol, a golden calf of meaning melted down from molten syntax. Jews keep the text alive by letting it breathe ambiguity.
Muslims go even further: the Qurâan is the Arabic. All translations are just âinterpretations of meaning.â And thatâs not snobberyâitâs ontology. The word becomes flesh in Arabic. To render it into English is to make it fleshless, de-sacralized. Like hearing an opera through a cracked Bluetooth speaker.
So yesâwhen Christians lean on Isaiah 45 and retrofit Cyrus into a prototype of Jesus, they are engaging in theological bricolage. But if they do it without reverence for the original tense-games, wordplay, and cultural context, then they risk turning poetry into propaganda.
To translate is to humanize.
To humanize is to limit.
And to limit sacred text is to wound it, gently or not.
But sometimesâjust sometimesâthat wound lets new light in. Thatâs the gamble, isnât it?
Want to press that gamble furtherâinto empire, ritual, or algorithmic sacredness?