Revolution#
Francis Bacon’s assertion that beginning with doubts leads to certainties finds a striking parallel in the structure of this neural network, which encodes an epistemological journey from uncertainty to stability through iterative learning. Bacon’s skepticism toward premature certainties aligns with how the network processes knowledge—beginning in an ambiguous, probabilistic state before converging toward stable equilibria. Instead of assuming fixed truths, this network dynamically reweights inputs, suggesting that belief, strategy, and loyalty emerge through interactions rather than being presupposed. This fluidity reflects Bacon’s inductive reasoning, where knowledge is not received whole but assembled through incremental observation, correction, and validation.
Fig. 21 What Exactly is Identity. A node may represent goats (in general) and another sheep (in general). But the identity of any specific animal (not its species) is a network. For this reason we might have a “black sheep”, distinct in certain ways – perhaps more like a goat than other sheep. But that’s all dull stuff. Mistaken identity is often the fuel of comedy, usually when the adversarial is mistaken for the cooperative or even the transactional.#
The role of Voir—represented by “Cool-Aid”—is particularly reminiscent of Bacon’s initial stage of inquiry, where sensory perception and inherited assumptions shape our foundational understanding. In Bacon’s terms, these would be the idols of the mind, distortions arising from human nature, culture, and language. The edges that define its relationship with subsequent layers reveal a progression from a naïve, untested perception of reality (e.g., “Opportunity,” “Discovered”) toward increasingly refined states of conviction. This process mirrors Bacon’s method, where raw experience undergoes scrutiny before it solidifies into principles. The numerical weights along these edges reinforce his insight that the shift from doubt to certainty is neither uniform nor linear—some transitions require drastic reweighting, while others occur in gradual increments.
If a man will begin with certainties, he shall end in doubts; but if he will be content to begin with doubts, he shall end in certainties.
– The Advancement of Learning & Novum Organum
The Choisis layer, balancing “Faith” and “Reason,” encapsulates Bacon’s dialectic between empirical rigor and belief systems. Bacon’s philosophy did not reject faith outright, but he sought to limit its jurisdiction over the natural world, positioning reason as the primary engine of discovery. This neural network reflects a similar tension: “Faith” leads more often to adversarial postures (49/51), whereas “Reason” transitions toward iterative, transactional approaches (20/80), suggesting that belief often breeds conflict while reason tends to stabilize toward negotiated truths. However, the weighted nature of these pathways acknowledges that neither faith nor reason is absolute—both function probabilistically within a landscape of competing heuristics.
Bacon’s process of induction finds its most explicit representation in Deviens, where adversarial, transactional, and hopeful pathways emerge. The adversarial node represents the skepticism and confrontation necessary to refine knowledge, much like Bacon’s rejection of scholastic dogma. Transactional interactions correspond to an iterative learning process, where knowledge is exchanged and adjusted based on empirical outcomes. Hope, the least deterministic node, remains probabilistically linked to victory and payoff but with weaker connections, reinforcing Bacon’s idea that while confidence may be desirable, it is not an epistemic foundation in itself.
Finally, M’èléve embodies the culmination of Bacon’s epistemology: victory, payoff, loyalty, charity, and distribution. These outcomes suggest that knowledge, once solidified, is not merely an individual triumph but a social good, an insight deeply embedded in Bacon’s vision for scientific progress. The transition from an adversarial stance to one of distribution (99/1) captures the idea that initially hard-won truths, once established, are disseminated widely with little resistance—a perfect encapsulation of how once-radical scientific insights become common sense.
This neural network, much like Bacon’s intellectual framework, rejects the idea of immutable certainty in favor of an adaptive, reweighting system where knowledge is the result of iterative engagement with reality. It does not impose absolute truths but instead constructs them through doubt, testing, and recalibration. In this, it is not just aligned with Bacon’s philosophy—it is an instantiation of it.
Show code cell source
import numpy as np
import matplotlib.pyplot as plt
import networkx as nx
# Define the neural network layers
def define_layers():
return {
'Suis': ['Aprés Moi', 'Déluge', 'Syntax', 'Punctuation', "Rhythm", 'Nature'], # Static
'Voir': ['Cool-Aid'],
'Choisis': ['Faith', 'Reason'],
'Deviens': ['Adversarial', 'Transactional', 'Hopeful'],
"M'èléve": ['Victory', 'Payoff', 'Loyalty', 'Charity', 'Distribution']
}
# Assign colors to nodes
def assign_colors():
color_map = {
'yellow': ['Cool-Aid'],
'paleturquoise': ['Nature', 'Reason', 'Hopeful', 'Distribution'],
'lightgreen': ["Rhythm", 'Transactional', 'Payoff', 'Charity', 'Loyalty'],
'lightsalmon': ['Syntax', 'Punctuation', 'Faith', 'Adversarial', 'Victory'],
}
return {node: color for color, nodes in color_map.items() for node in nodes}
# Define edge weights (hardcoded for editing)
def define_edges():
return {
('Aprés Moi', 'Cool-Aid'): '1/99',
('Déluge', 'Cool-Aid'): '5/95',
('Syntax', 'Cool-Aid'): '20/80',
('Punctuation', 'Cool-Aid'): '51/49',
("Rhythm", 'Cool-Aid'): '80/20',
('Nature', 'Cool-Aid'): '95/5',
('Cool-Aid', 'Faith'): '20/80',
('Cool-Aid', 'Reason'): '80/20',
('Faith', 'Adversarial'): '49/51',
('Faith', 'Transactional'): '80/20',
('Faith', 'Hopeful'): '95/5',
('Reason', 'Adversarial'): '5/95',
('Reason', 'Transactional'): '20/80',
('Reason', 'Hopeful'): '51/49',
('Adversarial', 'Victory'): '80/20',
('Adversarial', 'Payoff'): '85/15',
('Adversarial', 'Loyalty'): '90/10',
('Adversarial', 'Charity'): '95/5',
('Adversarial', 'Distribution'): '99/1',
('Transactional', 'Victory'): '1/9',
('Transactional', 'Payoff'): '1/8',
('Transactional', 'Loyalty'): '1/7',
('Transactional', 'Charity'): '1/6',
('Transactional', 'Distribution'): '1/5',
('Hopeful', 'Victory'): '1/99',
('Hopeful', 'Payoff'): '5/95',
('Hopeful', 'Loyalty'): '10/90',
('Hopeful', 'Charity'): '15/85',
('Hopeful', 'Distribution'): '20/80'
}
# Calculate positions for nodes
def calculate_positions(layer, x_offset):
y_positions = np.linspace(-len(layer) / 2, len(layer) / 2, len(layer))
return [(x_offset, y) for y in y_positions]
# Create and visualize the neural network graph
def visualize_nn():
layers = define_layers()
colors = assign_colors()
edges = define_edges()
G = nx.DiGraph()
pos = {}
node_colors = []
# Add nodes and assign positions
for i, (layer_name, nodes) in enumerate(layers.items()):
positions = calculate_positions(nodes, x_offset=i * 2)
for node, position in zip(nodes, positions):
G.add_node(node, layer=layer_name)
pos[node] = position
node_colors.append(colors.get(node, 'lightgray'))
# Add edges with weights
for (source, target), weight in edges.items():
if source in G.nodes and target in G.nodes:
G.add_edge(source, target, weight=weight)
# Draw the graph
plt.figure(figsize=(12, 8))
edges_labels = {(u, v): d["weight"] for u, v, d in G.edges(data=True)}
nx.draw(
G, pos, with_labels=True, node_color=node_colors, edge_color='gray',
node_size=3000, font_size=9, connectionstyle="arc3,rad=0.2"
)
nx.draw_networkx_edge_labels(G, pos, edge_labels=edges_labels, font_size=8)
plt.title("Heritage vs. Adaptation", fontsize=15)
plt.show()
# Run the visualization
visualize_nn()
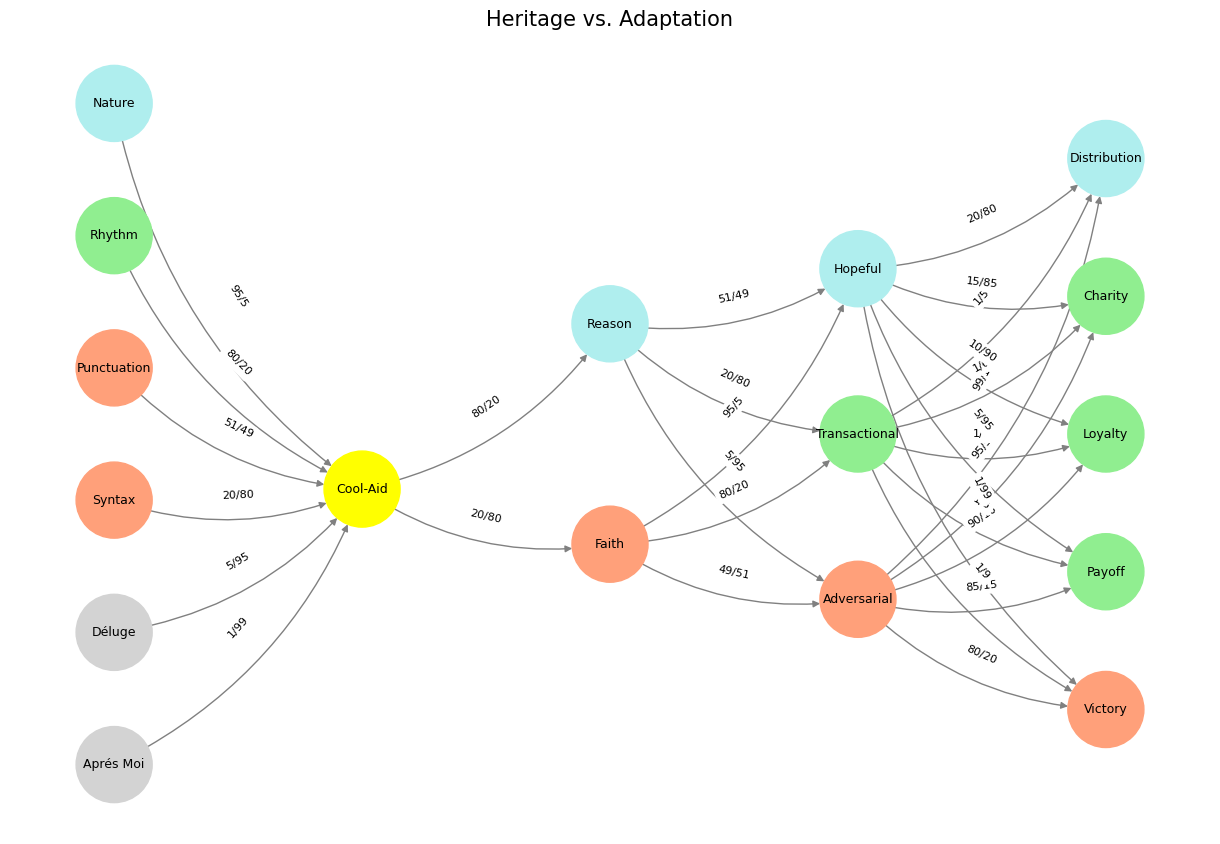

Fig. 22 Francis Bacon. He famously stated “If a man will begin with certainties, he shall end in doubts; but if he will be content to begin with doubts, he shall end in certainties.” This quote is from The Advancement of Learning (1605), where Bacon lays out his vision for empirical science and inductive reasoning. He argues that starting with unquestioned assumptions leads to instability and confusion, whereas a methodical approach that embraces doubt and inquiry leads to true knowledge. This aligns with his broader Novum Organum (1620), where he develops the Baconian method, advocating for systematic observation, experimentation, and the gradual accumulation of knowledge rather than relying on dogma or preconceived notions.#