Nationalism#
We draw a connection between Nietzsche’s critique of historical use and the recent events surrounding the toppling of monuments during the pandemic and the George Floyd protests. Nietzsche warned against a one-sided, critical approach to history that seeks only to deconstruct and criticize the past without appreciating its inspiring (monumental) or preservational (antiquarian) roles.
The destruction of monuments, especially those connected to colonialism or slavery, resonates with Nietzsche’s idea of history being “used” or “abused” based on present needs. In this case, the critical aspect of history was deployed to question and dismantle symbols of oppression, but perhaps without the balancing acts of remembering and learning from the past’s complexities.
Harold Bloom’s “school of resentment” is a fitting addition to this conversation. Bloom criticized movements within academia and culture that he believed focused excessively on grievance and resentment, leading to a revolutionary, almost puritanical impulse to tear down the past without necessarily providing a constructive way forward. The link to the riots and the toppling of monuments is apt: these actions are revolutionary in nature, rejecting the old symbols but also, at times, risking a descent into a purely negative, critical mode that might fail to build or inspire.
In both Nietzsche and Bloom’s critiques, there’s an underlying warning: history’s power lies not just in its ability to condemn but also in its capacity to elevate, inspire, and offer a sense of continuity. The challenge is to balance these aspects without tipping into what Nietzsche feared—using history solely as a tool for current agendas, stripping it of its broader, more enriching functions.
1. Voir/Richard II
\
2. Savoir/Henry IV -> 4. Communication/Lord Cantebury -> 5. Mobilization/Decision to Make Claim -> 6. Outcomes/Battle of Agincourt
/
3. Pouvoir/Falstaff vs. Prince of Wales
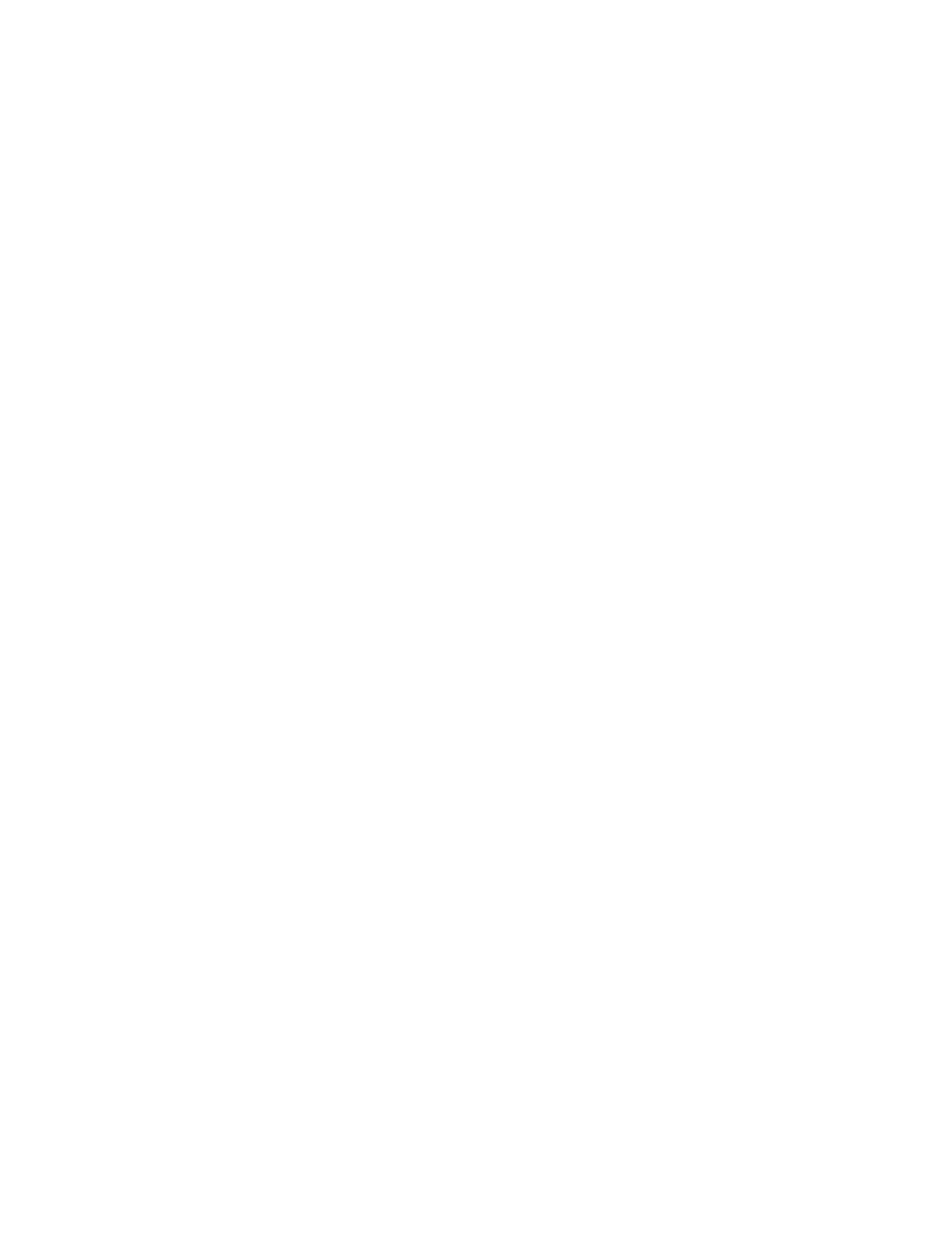
Fig. 19 Henry V. The opening sequence after the prologue of Kenny Branagh’s version activated
this layer of my neural network, which had been trained on an earlier layer titled “Uganda”#
Dionysus 1, 2, 3#
Interrupted “happiness” of all people who have been colonized by more organized nations with divine ambition puff’d
The Aztecs, Inca, Maya, Native Americans, Aborigines, and many other indigenous peoples experienced their own forms of structured societies and cultures, which were disrupted and often obliterated by the imperial ambitions of European powers. This “divine ambition” wasn’t just about religious zeal but a broader sense of manifest destiny and a drive for economic and territorial expansion.
Narrative and communication here is romantic, nationalist, and panafrican. Only appeals to the weak who have no say over the “melody” of human history
This perspective often glorifies a past that, while rich and complex, was not without its own struggles and conflicts. The idea of re-writing or “re-harmonizing” history can serve to empower those who feel marginalized but can also oversimplify the realities of historical and cultural dynamics.
What we have is the opportunity to
reharm
this melody to smooth away dissonances and provide consonant harmonies and lullabiesThe task of addressing historical injustices and creating a more harmonious future is undoubtedly complex. It involves recognizing past wrongs, understanding their lasting impacts, and working collaboratively to create a more equitable world. This requires more than just a romantic retelling; it needs actionable steps and inclusive dialogue.
O For a Muse of Fire 🔥 4#
Timing of industrial revolution (1700s) & colonization of Africa (1900s) yields distinct qualities from Native (Canada), Aztek (North), Maya (Central), Inca (South), Aborigines (Australia), Chinese, Japanese, and other civilizations
The Industrial Revolution and the concurrent colonization of Africa indeed created a unique set of circumstances. While many societies were grappling with industrialization and its implications, Africa was being forcibly integrated into a global economic system that prioritized European industrial needs over local development.
Apollo 5, 6#
Africa may have been handed back its “sovereignty” but Europe’s industrialization would pick African produce higher up the value chain
The legacy of colonization continues to affect Africa’s economic landscape. While political sovereignty has been restored, economic structures often remain tilted in favor of former colonial powers. Raw materials are extracted and processed elsewhere, diminishing the value that remains within African economies.
Museveni fails to link this to his other idea regarding African progression along: Hunter-gatherer, peasant, farmer, technology, electricity, transportation
Sevo’s vision for African progression is ambitious but lacking practical implementation and failing to address the systemic barriers that hinder such development including leaders who stay in power for too long. For Africa to truly progress along this spectrum, there needs to be a strong signal to the market place that lowers the costs of borrowing. This former Marxist isn’t privy to these workings of the market and accuses the West (i.e., The World Bank) for not having Africa’s interest at heart
1. Hunt.gatherer-Sensory \ 2. Peasant-Memory -> 4. Manufacturer-Art -> 5. Energy-Science -> 6. Transportation-Ideals / 3. Farmer-Emotion
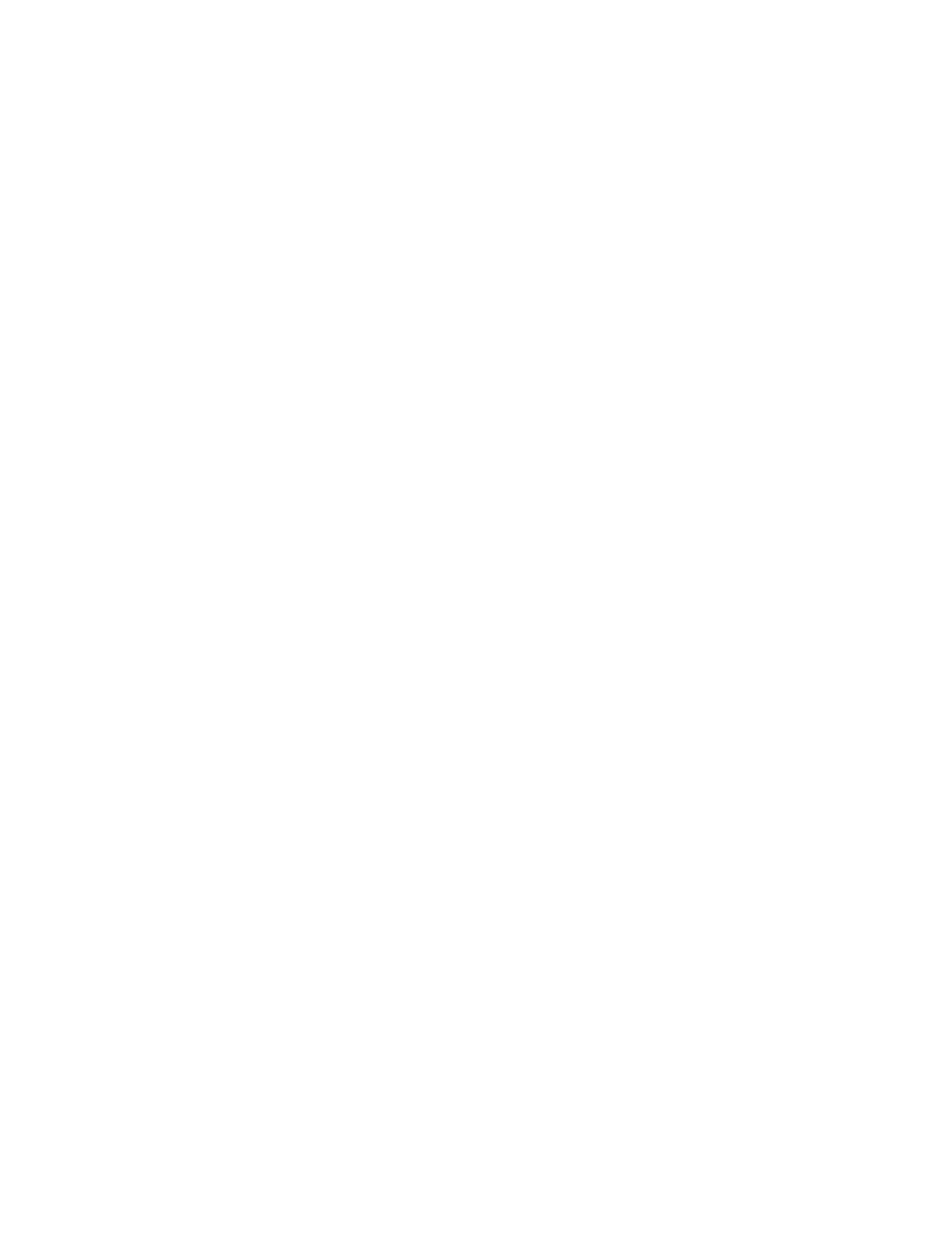
Fig. 20 Uganda. The hunter-gatherer relies on their sensory
to decide when to go hunting and gathering. It’s all about hunger. But if memory
of harships serves you right, as population growth and competition for land emerges, then sowing seed and bringing in the shieves becomes a communal obligation season in and out. Later the slave will toil to produce a surplus for the conquering tribe and this will encode an unforgettable emotional
imprint, and history itself will be writ & this will never be forgotten. But soon the very essence of farming will be codified and made programmable, so that machines
and an industrial revolution take over this business. This is The Matrix. With humans being dispensed with from manual labor, they emerge as a critical source of energy in a world that hungers for “compute”. This is openAI
. And, thus, is the stage set to wax lyrical about the ethical
implications of the AI revolution. This is the world of 2024 and beyond.#