Freedom in Fetters#
The shifting landscape of European aid presents a critical challenge to my app’s original positioning. With funding priorities moving decisively from aid to defense, the traditional avenues for institutional support are eroding. This realignment is not merely a shift in discretionary spending but a fundamental restructuring of European priorities in response to geopolitical instability. The consequence is an increasingly hostile environment for academic and research-driven projects, particularly those reliant on public or philanthropic funding streams that once prioritized global health and development. Where academic institutions and public health departments would have been my lowest-hanging fruit, they are now compromised by shrinking budgets and political recalibration. In light of this, my app must pivot toward funding sources that remain robust and, more importantly, aligned with the new realities of financial patronage.

Fig. 29 The five networks described in the essay, mapped to Uganda’s and Africa’s identity negotiation, unfold as follows: First, the Pericentral network (sensory-motor) governs reflexive responses, reacting to “nonself” threats like colonialism with immediate, physical action. Second, the Dorsal Frontoparietal network (goal-directed attention) focuses on detecting and prioritizing nonself entities, potentially faltering in Africa’s blurred boundaries with foreign influence. Third, the Lateral Frontoparietal network (flexible decision-making) navigates ambiguity, reflecting the continent’s struggle to balance tribal diversity and imposed systems. Fourth, the Medial Frontoparietal network (self-referential identity) turns inward, emphasizing self-coherence over external rejection, perhaps overly so in Africa’s history. Fifth, the Cingulo-Insular network (salience optimization) integrates these, ideally balancing self and nonself for efficiency—a convergence Africa might yet achieve. The order—from reflex to attention, ambiguity, identity, and optimization—mirrors a progression from instinctive reaction to reflective synthesis, suggesting a natural arc of development, though not necessarily a hierarchy; Africa’s “error” may lie in stalling at ambiguity or self-focus, short of full convergence.#
See also
European aid is now moving away from aid to defense
Strategically target Bill & Melinda
Look out for George Bruce Kaiser and his foundation
Vulnerability of my original targets makes these more key
Infrastructure and government might be dead (EY & Danz; Restructure Team; Deloitte mostly consulting; pivoted to tech)
This necessitates a strategic realignment towards entities with both liquidity and an enduring commitment to research and technological innovation. The Bill & Melinda Gates Foundation emerges as an obvious target, not just because of its historical investment in public health but because of its increasing focus on data-driven approaches to healthcare optimization. Gates’ emphasis on scalable, high-impact interventions suggests that a well-pitched alignment between my app’s individualized risk profiling and their ongoing initiatives could unlock substantial funding. The challenge, however, lies in ensuring that my approach distinguishes itself in an ecosystem saturated with health-tech solutions vying for attention. The Gates Foundation is not a passive patron; it seeks transformative potential, and my app’s value proposition must be framed not as an incremental improvement but as a necessary evolution in risk assessment.
Beyond Gates, figures like George Bruce Kaiser and his foundation present an alternative path. Kaiser, with his track record of philanthropic investments, has demonstrated a particular interest in innovative solutions targeting systemic inefficiencies in healthcare and education. If academic institutions are increasingly vulnerable, my pitch must shift towards foundations that recognize the shortcomings of traditional institutional frameworks and are actively looking to circumvent them through direct investment in disruptive technologies. This shift would not only ensure funding resilience but also position my app within a growing movement that seeks to bypass bureaucratic inertia in favor of agile, high-impact solutions.
The vulnerability of my original targets—academic institutions and their associated research departments—now makes this recalibration an imperative rather than a strategic choice. These institutions are facing a perfect storm of financial instability, shifting donor priorities, and an internal bureaucratic inertia that makes them ill-equipped to adapt swiftly. Even where funding still exists, institutions are increasingly risk-averse, unwilling to allocate resources to projects that do not align with their shrinking core mandates. This climate necessitates a move away from dependence on traditional academic structures and towards private philanthropic and strategic partnerships.
Note
Gen Z & Millenial are Recession Proof
Podcasts, Blogs, Authorship
Unleashing Identity or Negotiating It
Another dead zone in my original plan appears to be infrastructure and government funding. Once, there was a pathway through partnerships with entities like EY and Danz, whose restructuring teams engaged with public sector projects. However, the prevailing trend among major consulting firms—including Deloitte—has been a pivot towards tech-oriented services, largely at the expense of broader infrastructure and public-sector support. These firms are no longer positioning themselves as intermediaries for government-backed research initiatives; instead, they are aligning with private sector demands for efficiency and automation. This shift leaves little room for a project like mine, which was initially designed to interface with academic institutions and public health structures. Rather than chase dead capital, the more viable route is to align with the technology pivot already underway, embedding my app within the ecosystems of firms that are reshaping digital health rather than trying to persuade legacy institutions to fund something they no longer have the capacity to support.
The necessary conclusion is that my app must undergo a deliberate, high-stakes repivot. The previous assumption that academic institutions, public health departments, and infrastructure-backed consulting firms would provide the most immediate pathways to funding has been rendered obsolete by structural changes in financial and geopolitical priorities. The new strategy must target private foundations and high-net-worth philanthropic entities with both the resources and the ideological commitment to fostering innovation outside traditional academic frameworks. This repivot is not just about survival; it is an opportunity to position my app at the frontier of where funding and innovation are actually converging.
Show code cell source
import numpy as np
import matplotlib.pyplot as plt
import networkx as nx
# Define the neural network layers
def define_layers():
return {
'Suis': ['DNA, RNA, 5%', 'Peptidoglycans, Lipoteichoics', 'Lipopolysaccharide', 'N-Formylmethionine', "Glucans, Chitin", 'Specific Antigens'],
'Voir': ['PRR & ILCs, 20%'],
'Choisis': ['CD8+, 50%', 'CD4+'],
'Deviens': ['TNF-α, IL-6, IFN-γ', 'PD-1 & CTLA-4', 'Tregs, IL-10, TGF-β, 20%'],
"M'èléve": ['Complement System', 'Platelet System', 'Granulocyte System', 'Innate Lymphoid Cells, 5%', 'Adaptive Lymphoid Cells']
}
# Assign colors to nodes
def assign_colors():
color_map = {
'yellow': ['PRR & ILCs, 20%'],
'paleturquoise': ['Specific Antigens', 'CD4+', 'Tregs, IL-10, TGF-β, 20%', 'Adaptive Lymphoid Cells'],
'lightgreen': ["Glucans, Chitin", 'PD-1 & CTLA-4', 'Platelet System', 'Innate Lymphoid Cells, 5%', 'Granulocyte System'],
'lightsalmon': ['Lipopolysaccharide', 'N-Formylmethionine', 'CD8+, 50%', 'TNF-α, IL-6, IFN-γ', 'Complement System'],
}
return {node: color for color, nodes in color_map.items() for node in nodes}
# Define edge weights
def define_edges():
return {
('DNA, RNA, 5%', 'PRR & ILCs, 20%'): '1/99',
('Peptidoglycans, Lipoteichoics', 'PRR & ILCs, 20%'): '5/95',
('Lipopolysaccharide', 'PRR & ILCs, 20%'): '20/80',
('N-Formylmethionine', 'PRR & ILCs, 20%'): '51/49',
("Glucans, Chitin", 'PRR & ILCs, 20%'): '80/20',
('Specific Antigens', 'PRR & ILCs, 20%'): '95/5',
('PRR & ILCs, 20%', 'CD8+, 50%'): '20/80',
('PRR & ILCs, 20%', 'CD4+'): '80/20',
('CD8+, 50%', 'TNF-α, IL-6, IFN-γ'): '49/51',
('CD8+, 50%', 'PD-1 & CTLA-4'): '80/20',
('CD8+, 50%', 'Tregs, IL-10, TGF-β, 20%'): '95/5',
('CD4+', 'TNF-α, IL-6, IFN-γ'): '5/95',
('CD4+', 'PD-1 & CTLA-4'): '20/80',
('CD4+', 'Tregs, IL-10, TGF-β, 20%'): '51/49',
('TNF-α, IL-6, IFN-γ', 'Complement System'): '80/20',
('TNF-α, IL-6, IFN-γ', 'Platelet System'): '85/15',
('TNF-α, IL-6, IFN-γ', 'Granulocyte System'): '90/10',
('TNF-α, IL-6, IFN-γ', 'Innate Lymphoid Cells, 5%'): '95/5',
('TNF-α, IL-6, IFN-γ', 'Adaptive Lymphoid Cells'): '99/1',
('PD-1 & CTLA-4', 'Complement System'): '1/9',
('PD-1 & CTLA-4', 'Platelet System'): '1/8',
('PD-1 & CTLA-4', 'Granulocyte System'): '1/7',
('PD-1 & CTLA-4', 'Innate Lymphoid Cells, 5%'): '1/6',
('PD-1 & CTLA-4', 'Adaptive Lymphoid Cells'): '1/5',
('Tregs, IL-10, TGF-β, 20%', 'Complement System'): '1/99',
('Tregs, IL-10, TGF-β, 20%', 'Platelet System'): '5/95',
('Tregs, IL-10, TGF-β, 20%', 'Granulocyte System'): '10/90',
('Tregs, IL-10, TGF-β, 20%', 'Innate Lymphoid Cells, 5%'): '15/85',
('Tregs, IL-10, TGF-β, 20%', 'Adaptive Lymphoid Cells'): '20/80'
}
# Define edges to be highlighted in black
def define_black_edges():
return {
('DNA, RNA, 5%', 'PRR & ILCs, 20%'): '1/99',
('Peptidoglycans, Lipoteichoics', 'PRR & ILCs, 20%'): '5/95',
('Lipopolysaccharide', 'PRR & ILCs, 20%'): '20/80',
('N-Formylmethionine', 'PRR & ILCs, 20%'): '51/49',
("Glucans, Chitin", 'PRR & ILCs, 20%'): '80/20',
('Specific Antigens', 'PRR & ILCs, 20%'): '95/5',
}
# Calculate node positions
def calculate_positions(layer, x_offset):
y_positions = np.linspace(-len(layer) / 2, len(layer) / 2, len(layer))
return [(x_offset, y) for y in y_positions]
# Create and visualize the neural network graph
def visualize_nn():
layers = define_layers()
colors = assign_colors()
edges = define_edges()
black_edges = define_black_edges()
G = nx.DiGraph()
pos = {}
node_colors = []
# Create mapping from original node names to numbered labels
mapping = {}
counter = 1
for layer in layers.values():
for node in layer:
mapping[node] = f"{counter}. {node}"
counter += 1
# Add nodes with new numbered labels and assign positions
for i, (layer_name, nodes) in enumerate(layers.items()):
positions = calculate_positions(nodes, x_offset=i * 2)
for node, position in zip(nodes, positions):
new_node = mapping[node]
G.add_node(new_node, layer=layer_name)
pos[new_node] = position
node_colors.append(colors.get(node, 'lightgray'))
# Add edges with updated node labels
edge_colors = []
for (source, target), weight in edges.items():
if source in mapping and target in mapping:
new_source = mapping[source]
new_target = mapping[target]
G.add_edge(new_source, new_target, weight=weight)
edge_colors.append('black' if (source, target) in black_edges else 'lightgrey')
# Draw the graph
plt.figure(figsize=(12, 8))
edges_labels = {(u, v): d["weight"] for u, v, d in G.edges(data=True)}
nx.draw(
G, pos, with_labels=True, node_color=node_colors, edge_color=edge_colors,
node_size=3000, font_size=9, connectionstyle="arc3,rad=0.2"
)
nx.draw_networkx_edge_labels(G, pos, edge_labels=edges_labels, font_size=8)
plt.title("OPRAH™ aAPCs", fontsize=18)
plt.show()
# Run the visualization
visualize_nn()
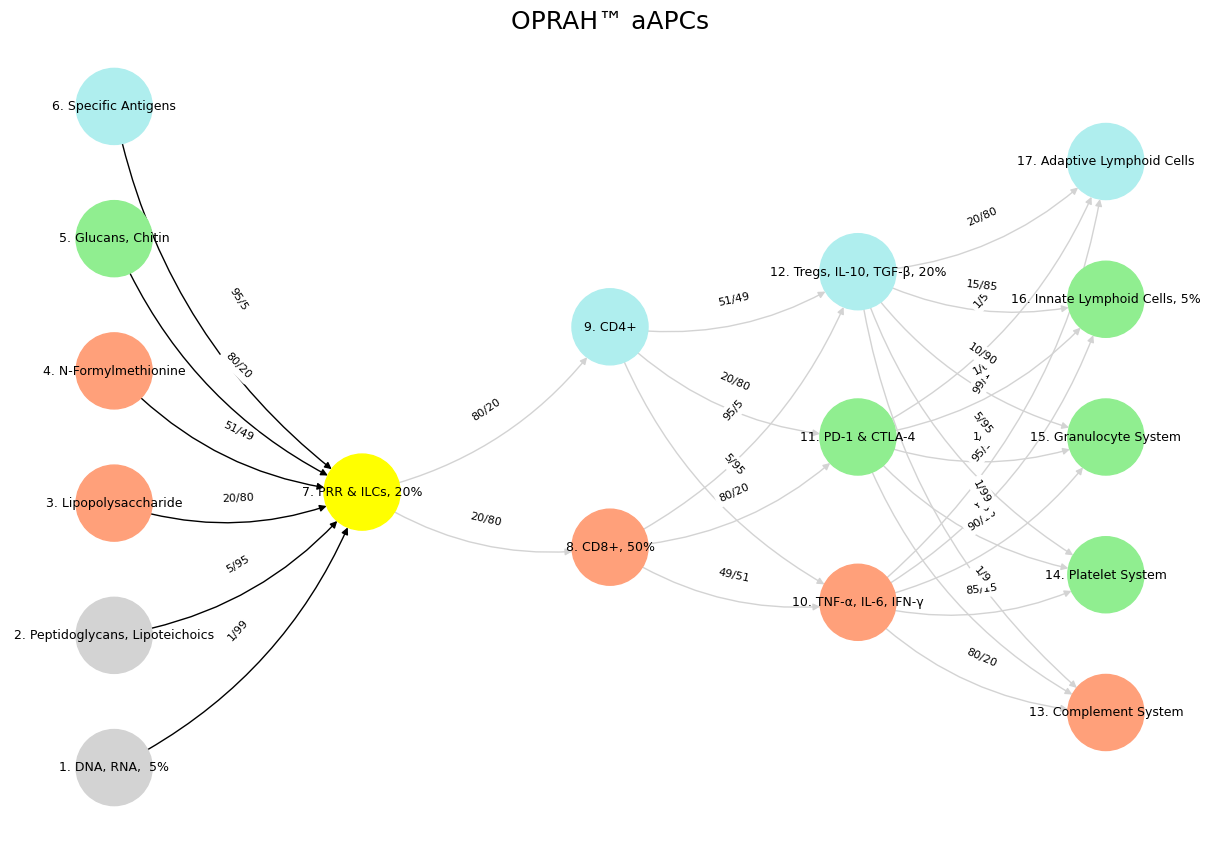
#
Fig. 30 Musical Grammar & Prosody. From a pianist’s perspective, the left hand serves as the foundational architect, voicing the mode and defining the musical landscape—its space and grammar—while the right hand acts as the expressive wanderer, freely extending and altering these modal terrains within temporal pockets, guided by prosody and cadence. In R&B, this interplay often manifests through rich harmonic extensions like 9ths, 11ths, and 13ths, with chromatic passing chords and leading tones adding tension and color. Music’s evocative power lies in its ability to transmit information through a primal, pattern-recognizing architecture, compelling listeners to classify what they hear as either nurturing or threatening—feeding and breeding or fight and flight. This makes music a high-risk, high-reward endeavor, where success hinges on navigating the fine line between coherence and error. Similarly, pattern recognition extends to literature, as seen in Ulysses, where a character misinterprets his companion’s silence as mental composition, reflecting on the instructive pleasures of Shakespearean works used to solve life’s complexities. Both music and literature, then, are deeply rooted in the human impulse to decode and derive meaning, whether through harmonic landscapes or textual introspection. Source: Ulysses, DeepSeek & Yours Truly!#