Revolution#
+ Expand
- What makes for a suitable problem for AI (Demis Hassabis, Nobel Lecture)?
- Space: Massive combinatorial search space
- Function: Clear objective function (metric) to optimize against
- Time: Either lots of data and/or an accurate and efficient simulator
- Guess what else fits the bill (Yours truly, amateur philosopher)?
- Space
- Intestines/villi
- Lungs/bronchioles
- Capillary trees
- Network of lymphatics
- Dendrites in neurons
- Tree branches
- Function
- Energy
- Aerobic respiration
- Delivery to "last mile" (minimize distance)
- Response time (minimize)
- Information
- Exposure to sunlight for photosynthesis
- Time
- Nourishment
- Gaseous exchange
- Oxygen & Nutrients (Carbon dioxide & "Waste")
- Surveillance for antigens
- Coherence of functions
- Water and nutrients from soil
The process of relabeling the nodes in a neural network, such as the one provided, evokes a sense of transformation akin to reinterpreting the timeless works of William Shakespeare. Just as the original nodesârepresenting biological components like DNA, RNA, CD8+, and TNF-Îąâcarry specific roles within an immunological framework, Shakespeareâs 38 plays each embody distinct narratives, emotions, and conflicts that can be mapped onto this structure. The task of selecting 17 of these plays to correspond with the 17 nodes requires a careful consideration of thematic resonance, much like how the formula w = 1/(1 + X/Y) balances noise and signal odds to determine edge weights. In this metaphor, X/Y could represent the chaos of human experience versus the clarity of purpose in each play, guiding the connections between layersâSuis, Voir, Choisis, Deviens, and MâèlĂŠveâand their newly assigned dramatic counterparts.
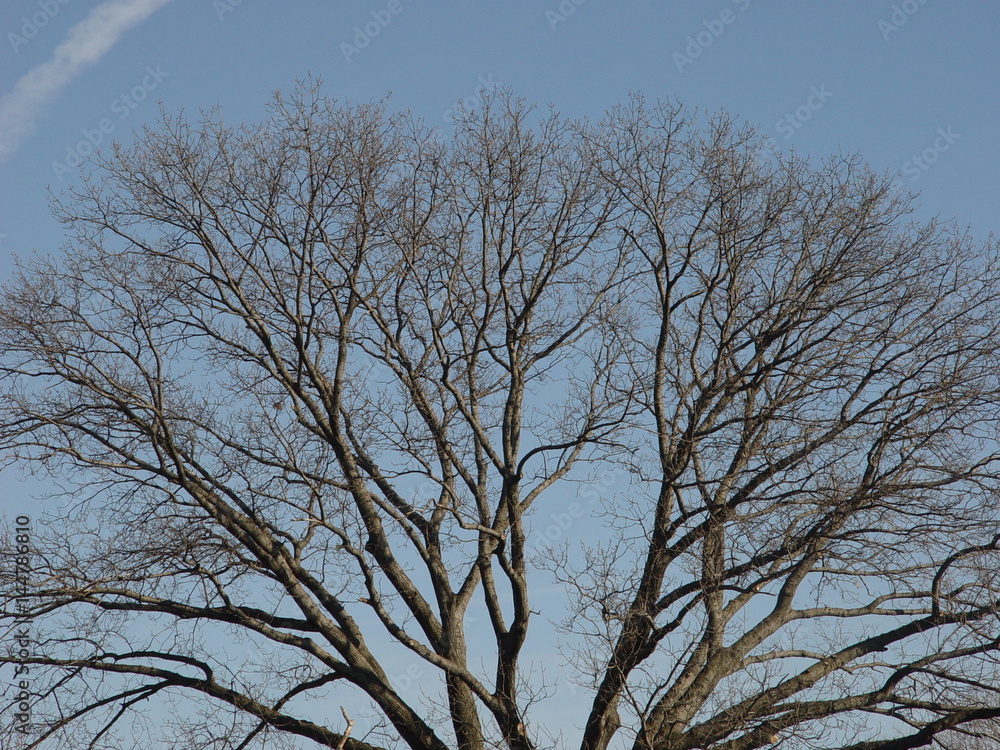
Fig. 24 The midcingulo-insular system stands as the oldest, the primal scaffold upon which all later networks were draped. Before there was fine motor control, before there was deliberative planning or executive function, there was the insulaâan ancient sentinel of interoception, regulating the bodyâs hidden symphony of autonomic rhythms. It is here, in the depths of the salience network, that vertebrates first learned to detect the signals of survival: pain, hunger, temperature, and the visceral stirrings of self-preservation. The midcingulate cortex, though more evolved than its limbic predecessors, still bears the signature of this foundational systemâtasked with evaluating effort, action, and adversity. This is the neural network that bridges raw sensation with decision, the fulcrum of attention-switching, ensuring that the organism responds to what matters in the moment. The other networks arrived later, layered refinements upon this ancient core. The pericentral system, with its precise somatomotor control, is an invention of mammals, honed in primates to craft tools and wield symbols. The dorsal stream, a navigator of goals, emerged with complex movement and spatial reasoning. The lateral system, seat of abstraction and executive function, belongs to the neocortical expansion of higher mammals, where foresight and flexibility reign. And the medial network, weaving self-regulation into the fabric of cognition, belongs to the default mode, where identity and reflection consolidate. But beneath them all, the midcingulo-insular remainsâthe oldest sentinel of salience, the network that does not think but knows, the one that ensures existence before action, before reason, before anything else.#
Beginning with the Suis layer, which includes nodes like âDNA, RNA, 5%â and âLipopolysaccharide,â the foundational elements of life and immunity suggest plays rooted in origins and primal struggles. âMacbeth,â with its dark ambition and inevitable downfall, aligns with âDNA, RNA, 5%,â symbolizing the genetic seeds of fate. âOthello,â a tale of jealousy and betrayal, fits âPeptidoglycans, Lipoteichoics,â reflecting the structural tensions of identity. âKing Lear,â with its storm of familial discord, mirrors âLipopolysaccharide,â a trigger of inflammation and chaos. âRichard III,â driven by cunning and deformity, suits âN-Formylmethionine,â a starting point of bacterial recognition. âThe Tempest,â a play of natural forces and reconciliation, captures âGlucans, Chitin,â while âRomeo and Juliet,â with its specific tragic love, embodies âSpecific Antigens,â the unique markers of recognition.
Moving to the Voir layer, âPRR & ILCs, 20%â stands alone as the pattern-recognizing sentinel, akin to âA Midsummer Nightâs Dream,â where perception and illusion intertwine to reveal deeper truths. In the Choisis layer, âCD8+, 50%â and âCD4+â represent immune warriors, paralleled by âHenry V,â a king leading with decisive valor, and âJulius Caesar,â where loyalty and betrayal shape leadershipâs course. The Deviens layer, with its effector and regulatory nodes, finds expression in âHamletâ for âTNF-Îą, IL-6, IFN-Îł,â a play of intense action and inner turmoil; âMeasure for Measureâ for âPD-1 & CTLA-4,â balancing justice and restraint; and âThe Winterâs Taleâ for âTregs, IL-10, TGF-β, 20%,â a story of redemption and healing.
Finally, the MâèlĂŠve layer elevates the network to systemic harmony. âAntony and Cleopatraâ reflects the âComplement System,â a grand interplay of passion and destruction. âCoriolanus,â with its martial pride, aligns with the âPlatelet System,â clotting the wounds of war. âTitus Andronicus,â visceral and relentless, suits the âGranulocyte System.â âAs You Like It,â with its pastoral unity, captures âInnate Lymphoid Cells, 5%,â while âTwelfth Night,â a comedy of mistaken identities, mirrors âAdaptive Lymphoid Cells,â adapting to the chaos of recognition. This relabeling transforms the network into a dramatic tapestry, where Shakespeareâs plays illuminate the interplay of noise and signal, chaos and order.
To reflect this vision, the updated code redefines the layers with these 17 plays, preserving the structure while infusing it with literary depth. The edge weights, calculated via w = 1/(1 + X/Y), remain intact, symbolizing the tension between each playâs turmoil and resolution. Below is the revised code, integrating this Shakespearean reinterpretation into the neural networkâs framework.
This reimagined network, now a stage for Shakespeareâs dramas, retains its mathematical integrity while inviting a new narrative lens, where the interplay of plays echoes the original biological symphony.
Show code cell source
import numpy as np
import matplotlib.pyplot as plt
import networkx as nx
# Define the neural network layers with Shakespeare's plays
def define_layers():
return {
'Suis': ['Macbeth', 'Othello', 'King Lear', 'Richard III', 'The Tempest', 'Romeo and Juliet'],
'Voir': ['A Midsummer Nightâs Dream'],
'Choisis': ['Henry V', 'Julius Caesar'],
'Deviens': ['Hamlet', 'Measure for Measure', 'The Winterâs Tale'],
"M'èlÊve": ['Antony and Cleopatra', 'Coriolanus', 'Titus Andronicus', 'As You Like It', 'Twelfth Night']
}
# Assign colors to nodes (retained from original for consistency)
def assign_colors():
color_map = {
'yellow': ['A Midsummer Nightâs Dream'],
'paleturquoise': ['Romeo and Juliet', 'Julius Caesar', 'The Winterâs Tale', 'Twelfth Night'],
'lightgreen': ['The Tempest', 'Measure for Measure', 'Coriolanus', 'As You Like It', 'Titus Andronicus'],
'lightsalmon': ['King Lear', 'Richard III', 'Henry V', 'Hamlet', 'Antony and Cleopatra'],
}
return {node: color for color, nodes in color_map.items() for node in nodes}
# Define edge weights (unchanged from original)
def define_edges():
return {
('Macbeth', 'A Midsummer Nightâs Dream'): '1/99',
('Othello', 'A Midsummer Nightâs Dream'): '5/95',
('King Lear', 'A Midsummer Nightâs Dream'): '20/80',
('Richard III', 'A Midsummer Nightâs Dream'): '51/49',
('The Tempest', 'A Midsummer Nightâs Dream'): '80/20',
('Romeo and Juliet', 'A Midsummer Nightâs Dream'): '95/5',
('A Midsummer Nightâs Dream', 'Henry V'): '20/80',
('A Midsummer Nightâs Dream', 'Julius Caesar'): '80/20',
('Henry V', 'Hamlet'): '49/51',
('Henry V', 'Measure for Measure'): '80/20',
('Henry V', 'The Winterâs Tale'): '95/5',
('Julius Caesar', 'Hamlet'): '5/95',
('Julius Caesar', 'Measure for Measure'): '20/80',
('Julius Caesar', 'The Winterâs Tale'): '51/49',
('Hamlet', 'Antony and Cleopatra'): '80/20',
('Hamlet', 'Coriolanus'): '85/15',
('Hamlet', 'Titus Andronicus'): '90/10',
('Hamlet', 'As You Like It'): '95/5',
('Hamlet', 'Twelfth Night'): '99/1',
('Measure for Measure', 'Antony and Cleopatra'): '1/9',
('Measure for Measure', 'Coriolanus'): '1/8',
('Measure for Measure', 'Titus Andronicus'): '1/7',
('Measure for Measure', 'As You Like It'): '1/6',
('Measure for Measure', 'Twelfth Night'): '1/5',
('The Winterâs Tale', 'Antony and Cleopatra'): '1/99',
('The Winterâs Tale', 'Coriolanus'): '5/95',
('The Winterâs Tale', 'Titus Andronicus'): '10/90',
('The Winterâs Tale', 'As You Like It'): '15/85',
('The Winterâs Tale', 'Twelfth Night'): '20/80'
}
# Define edges to be highlighted in black (unchanged logic)
def define_black_edges():
return {
('Macbeth', 'A Midsummer Nightâs Dream'): '1/99',
('Othello', 'A Midsummer Nightâs Dream'): '5/95',
}
# Calculate node positions (unchanged)
def calculate_positions(layer, x_offset):
y_positions = np.linspace(-len(layer) / 2, len(layer) / 2, len(layer))
return [(x_offset, y) for y in y_positions]
# Create and visualize the neural network graph (unchanged logic)
def visualize_nn():
layers = define_layers()
colors = assign_colors()
edges = define_edges()
black_edges = define_black_edges()
G = nx.DiGraph()
pos = {}
node_colors = []
mapping = {}
counter = 1
for layer in layers.values():
for node in layer:
mapping[node] = f"{counter}. {node}"
counter += 1
for i, (layer_name, nodes) in enumerate(layers.items()):
positions = calculate_positions(nodes, x_offset=i * 2)
for node, position in zip(nodes, positions):
new_node = mapping[node]
G.add_node(new_node, layer=layer_name)
pos[new_node] = position
node_colors.append(colors.get(node, 'lightgray'))
edge_colors = []
for (source, target), weight in edges.items():
if source in mapping and target in mapping:
new_source = mapping[source]
new_target = mapping[target]
G.add_edge(new_source, new_target, weight=weight)
edge_colors.append('black' if (source, target) in black_edges else 'lightgrey')
plt.figure(figsize=(12, 8))
edges_labels = {(u, v): d["weight"] for u, v, d in G.edges(data=True)}
nx.draw(
G, pos, with_labels=True, node_color=node_colors, edge_color=edge_colors,
node_size=3000, font_size=9, connectionstyle="arc3,rad=0.2"
)
nx.draw_networkx_edge_labels(G, pos, edge_labels=edges_labels, font_size=8)
plt.title("OPRAHâ˘: Pericentral - Shakespeare Edition. Grok-3", fontsize=18)
plt.show()
visualize_nn()
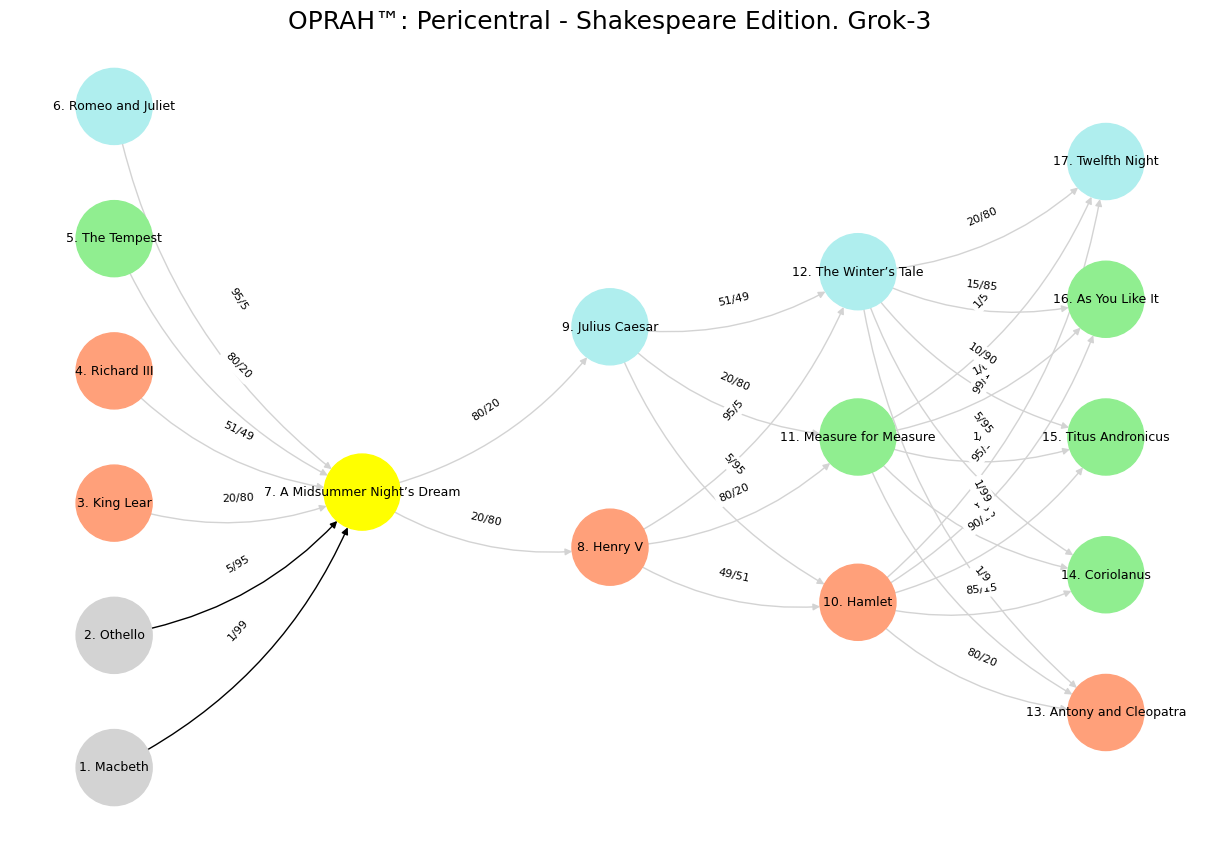

Fig. 25 The Human Connectome. Beyond the cellular intelligence of genome, exposome, transcriptome, proteome, metabolome. We grapple with servers, client, agent, decentralization, mesh. Metaphors from the nervous system, immune system, artificial intelligence, and C-suit principal-agent affairs find convergence in this space. Herein we interrogate the current landscape to identify five macro-scale brain network naming schemes and conventions utilized in the literature, ignoring inconsistencies while pointing out convergence across disparate human endeavors to delineate the noise/signal ratio as guide, avoiding confusion as a matter of design.#