Revolution#
Enterprise, Portfolio Theory, and the Logic of Cost-Cutting: A Structural Critique#
In conceptualizing an enterprise, denoted as \( Y \), we can initially define it as the sum of its structural components: its costs, its agent, its market correlations, and its revenues. The algebraic formulation you propose,
captures the fundamental forces at play in an enterprise but requires refinement in both mathematical structure and interpretation.
Fig. 21 What Exactly is Identity. A node may represent goats (in general) and another sheep (in general). But the identity of any specific animal (not its species) is a network. For this reason we might have a “black sheep”, distinct in certain ways – perhaps more like a goat than other sheep. But that’s all dull stuff. Mistaken identity is often the fuel of comedy, usually when the adversarial is mistaken for the cooperative or even the transactional.#
Breaking Down the Enterprise Function#
Each component of this equation reflects a different dimension of the enterprise:
Costs \((-C)\): These represent expenditures on land, labor, capital, and overhead—core inputs required to sustain operations.
Agent \((\alpha)\): The principal-agent dynamic emerges here, where an agent (manager, CEO, or key operator) makes decisions that influence the efficiency and direction of the enterprise.
Market Structure \((X\beta)\): This term derives from portfolio theory, where \( X \) represents a set of factors (e.g., competitors, macroeconomic conditions), and \( \beta \) reflects the correlation of the enterprise with these market forces.
Revenues \((R)\): The total income generated by the enterprise through sales, services, or other means.
Structurally, \( Y \) represents the net state of the enterprise. If revenues exceed costs (\( R > C \)), the enterprise is profitable; otherwise, it faces losses. However, the introduction of \( \alpha \) and \( X\beta \) introduces agency and strategic positioning into the equation.
Refining the Algebra: A Portfolio-Theoretic Lens#
A more precise representation would be:
This places the revenue-minus-cost term at the forefront, making it clear that enterprise value is first determined by its fundamental profitability before adjustments for agency and market structure. Here, \( \alpha \) functions as an optimization mechanism, where the agent influences either cost reduction or revenue expansion.
From a portfolio perspective, \( X\beta \) suggests that the enterprise does not exist in isolation; its value is partially determined by its correlation with market peers. This term captures systematic risk and potential competitive advantage.
Agent Optimization: The Cost-Cutting CEO#
Your insight that an agent—\( \alpha \)—can maximize their perceived value by minimizing \( C \) is a classic principal-agent dilemma. An agent who aggressively cuts costs (e.g., layoffs, automation, outsourcing) can generate immediate boosts in net enterprise value while potentially damaging long-term stability. This is the Muskian efficiency model: fire half the staff, maintain functionality, and reframe the enterprise as leaner.
This aligns with the real-world example in the images you provided. If the federal government applies this logic, it effectively transforms itself into an enterprise seeking to maximize efficiency through mass layoffs. The decision to offer employees the choice to “resign voluntarily” mirrors Musk’s approach to restructuring Twitter: create an ultimatum (e.g., “hardcore work culture”) that pressures resignations without direct termination costs.
The Socioeconomic Implications: Enterprise as a Political Model#
Your mapping of enterprise components to social structures is intriguing:
Enterprise (\( Y \)) → Heir: The entity itself represents continuity, inheritance, and survival.
Costs (\( -C \)) → Genius: This frames cost as an intellectual burden—the weight of maintaining infrastructure, expertise, and legacy.
Agent (\( \alpha \)) → Brand: The public face, decision-making force, and perceived value creator.
Market Structure (\( X\beta \)) → Tribe: The positioning within a network, subject to external forces.
Revenues (\( R \)) → Religion: The sustaining force, providing justification for the system’s existence.
This framework suggests a broader argument: The economy itself is an enterprise with a principal-agent structure, where political leaders (agents) optimize for either cost-cutting or expansion, with social groups and institutions playing the role of stakeholders.
The emails to federal employees represent an attempt to transform government into a cost-efficient enterprise by forcing an exodus of redundant labor. The language of voluntary resignation mimics corporate downsizing, reframing layoffs as a strategic optimization rather than a failure of governance.
Final Refinement: Rethinking the Equation#
Your equation, refined in the context of political and economic systems, might take the following form:
where \( \alpha(X, \beta) \) captures how an agent leverages market positioning to optimize the enterprise. This formulation clarifies that revenue generation and cost efficiency are primary drivers, with agency as a secondary but crucial layer of influence.
Conclusion: The Musk Doctrine in Government#
The restructuring of Twitter and the proposed government downsizing both follow the same logic: remove inefficiencies, force an adaptive response, and maintain core functionality with a leaner system. Whether this approach enhances long-term resilience or merely strips institutions to their skeletal form remains the open question.
Your instinct to fuse enterprise theory with sociopolitical structures is powerful. The next step would be to explore how these forces interact dynamically over time—whether agents like Musk create sustainable efficiencies or simply engineer short-term gains at the cost of systemic fragility.
Gift from Elon#
This wasn’t included in the initial information. Do you think you’ll update anything fundamentally given what I’ve added?
Yes, this new information sharpens the focus of the analysis, particularly in framing the government’s restructuring as an extreme case of enterprise optimization. The phrase shrink, purge, and remake suggests a broader ideological transformation, rather than merely a cost-cutting exercise. It reinforces the idea that this is not just about efficiency, but also about reshaping institutional power to align with a political vision.
Fundamentally, this means I would update the critique in the following ways:
From Efficiency to Political Purification
The original framework saw \( \alpha \), the agent, as a purely cost-cutting force. However, this update suggests a dual role: the agent is both an optimizer (minimizing \( C \)) and a curator (realigning the organization to fit a new ideological framework).
This means \( \alpha \) is not just Muskian efficiency but also a form of political restructuring. This resembles corporate hostile takeovers where management is gutted to impose a new vision.
Revising the Market Structure \( X\beta \)
The earlier assumption was that \( X\beta \) represents an enterprise’s relationship to the market. However, in the context of government, \( X\beta \) represents the institutional inertia that needs to be overcome.
The fact that employees are offered voluntary resignation means that the approach is not purely about brute force but rather about game-theoretic manipulation: incentivizing a subset of the workforce to self-eliminate.
Heir, Genius, Brand, Tribe, and Religion Revisited
The notion of heir (\( Y \)) as an entity that persists through transformation is critical here. If Musk at Twitter was the prototype, then government restructuring under Trump follows the same blueprint: purge the old, consolidate control, and redefine the function of the institution.
Genius (\( -C \)) as the burden of legacy institutions now takes on a sharper edge: the weight of history, bureaucracy, and expertise is not just a cost to be minimized but a liability in the eyes of political transformation.
Brand (\( \alpha \)), the agent, is both the enforcer and the symbol of change.
Tribe (\( X\beta \)) represents networks of internal resistance, whether ideological or bureaucratic.
Religion (\( R \)), revenue in an enterprise model, now maps onto the justification framework: the higher moral or ideological reason that sustains the purge.
The “Fork in the Road” as a Coordination Mechanism
The email offer serves as a signaling mechanism. In portfolio theory, investors respond to signals about the health of a firm. Here, employees are given a choice that doubles as a signal about their alignment: those who resign are acknowledging that they do not fit the new order.
The voluntary aspect mirrors Musk’s Twitter ultimatum: “Are you in or out?” This is classic adversarial equilibrium design.
Final Refinement of the Model#
Given this, the equation would now take this refined form:
where \( P \) represents political alignment as an additional input modifying the agent’s behavior. This captures the fact that in political institutions, cost-cutting is not purely about efficiency but also about ideological conformity.
This update makes the model not just about enterprise management but also about statecraft as a form of corporate restructuring. The Musk-Trump parallel is no longer incidental; it’s a playbook.
It’s Poker Time#
The Federal Employee’s Gamble: A Game of Chance, Strategy, and Agency#
To view the enterprise equation
through the lens of games is to situate existence itself within immutable laws of probability, strategy, and human agency. The framework shifts from a deterministic model of enterprise mechanics to a probabilistic one, where outcomes are contingent on the game being played and the player’s skill in navigating it.
At the core of this perspective is \( Y \), the fundamental state of being, which belongs to the realm of a coin toss, a dice roll, a roulette wheel—the domain of fate. This is the realm of heredity, where an individual’s starting conditions are set long before they make a single decision. The federal employee caught in the throes of mass restructuring finds himself here: thrown into a game whose rules he did not design, where a decision must be made with incomplete information. Whether he was hired last year or thirty years ago, whether he has savings or lives paycheck to paycheck, whether he belongs to a powerful union or stands alone—these are the equivalent of the cards he was dealt at the beginning of the hand. He may play them skillfully, but the deck was shuffled before he sat down.
Then comes \( -C \), the world of poker, labor, and capital, the world of wagers and calculated risks. If \( Y \) is the existential given, then \( -C \) is the active bet against fate. The federal employee must decide: Does he fold and take the severance? Does he call, holding onto his position in defiance of uncertainty? Does he raise, engaging in legal battles through a union or finding an alternative power play? In poker, the worker is constantly balancing risk and return, instinct and calculation. A brilliant player, like a genius in any field, operates on the razor’s edge between mathematics and psychology, between reading the game and imposing one’s own logic upon it. The federal worker, if unionized, has a hand to play. If not, he is like a lone gambler staring at a weak set of cards, weighing whether he can bluff his way to a better outcome.
Next, \( \alpha \), the agent, takes us into the world of horse racing, car racing, and Formula One. Here, the gambler is no longer passive; he is a driver, a jockey, a figure of sheer control and precision. Unlike poker, where the player adapts to an uncertain game, in racing the player is the game, commanding speed, taking corners at the perfect moment, pushing every advantage. A federal worker who understands the terrain, who sees beyond the immediate choice of resigning or staying, who can maneuver through the bureaucratic labyrinth with the finesse of Lewis Hamilton threading through an overtaking gap, is an agent in the fullest sense. Here, some may choose to stay not because they believe in stability, but because they know how to navigate shifting political realities—perhaps securing a promotion amid the chaos, perhaps aligning themselves with the ideological purging to ensure survival. Others may simply take the money and run, their race already lost before the starting lights go out.
Then there is \( X\beta \), the world of chess and war, the realm of structured, hierarchical control. If the federal employee is playing poker when assessing severance and Formula One when maneuvering for advantage, then bureaucracy itself is chess. The government is a vast board where pieces move not according to personal agency but by the dictates of overarching strategies. Here, a manager plays with inanimate objects, shifting policies and numbers, shaping outcomes without concern for individual will. In contrast, in war, the pieces are human, and yet, the best soldier is expected to behave like a chess piece—executing orders without question. Some federal employees, particularly in national security, find themselves here: if they behave as chess pieces, they may remain on the board; if they become rogue agents, like Colonel Kurtz in Apocalypse Now, they may be neutralized, cast out as liabilities to the system.
Finally, we arrive at \( R \), the force of love, passion, romance, and singularity. This may seem out of place in a framework dominated by strategy and probability, but it is, in fact, the core of decision-making. A federal employee deciding whether to stay or leave is not merely making a rational calculation about salary and benefits. He is also deciding based on identity, values, relationships. If he loves the work, if he feels bound to a mission, he may stay against all odds. If his passion has waned, if the thrill is gone, if the environment has turned adversarial, then leaving becomes an act of self-preservation. Love, here, is the irrational wildcard, the unaccounted-for variable, the move that defies logic but may ultimately be the most human of all.
The Federal Employee’s Move: What Game Should He Play?#
If the federal employee sees his predicament as poker, he must assess his hand. Is he holding aces, protected by a powerful union, with a strong bargaining position? Or is he barely scraping by, with nothing to leverage but luck and a bluff? If poker is the right framework, he should think in terms of odds and counter-moves—when to fold, when to push, when to wait for the next round.
If he sees it as Formula One, he should ask: Is he a skilled enough driver to navigate this crisis? Can he accelerate into an opportunity while others crash out? Is this his moment to seize control, or should he pull into the pits and regroup for another race?
If he sees it as chess or war, he must determine whether he is a piece or a player. If he is merely a pawn, his best strategy may be to avoid the battlefield altogether. If he is a commanding force, he must be strategic, anticipating not just the next move but the moves after that.
If he sees it through the lens of love, the question is: What does he truly want? Is staying in this job an act of passion, duty, or desperation? Is leaving a rejection or an embrace of something new?
Conclusion: The Real Stakes of the Gamble#
Gamifying this framework is appropriate because this is, in every way, a high-stakes game. The federal employee does not operate in a vacuum; he is in a contest where the rules have suddenly changed, and he must decide which game he is playing before he can even make a move.
The worst mistake would be to assume that this is just another bureaucratic restructuring, just another budget cut. This is adversarial play—the employer has made its move, and now the employee must respond. But if he chooses the wrong game, he is already lost.
Should he hold, fold, accelerate, retreat, strategize, or surrender to love? That is the question. The answer depends on who he is—not just as a worker, but as a player in the grand game of existence.
Show code cell source
import numpy as np
import matplotlib.pyplot as plt
import networkx as nx
# Define the neural network fractal
def define_layers():
return {
'World': ['Cosmos-Entropy', 'Planet-Tempered', 'Life-Needs', 'Ecosystem-Costs', 'Generative-Means', 'Cartel-Ends', ], # Polytheism, Olympus, Kingdom
'Perception': ['Perception-Ledger'], # God, Judgement Day, Key
'Agency': ['Open-Nomiddleman', 'Closed-Trusted'], # Evil & Good
'Generative': ['Ratio-Weaponized', 'Competition-Tokenized', 'Odds-Monopolized'], # Dynamics, Compromises
'Physical': ['Volatile-Revolutionary', 'Unveiled-Resentment', 'Freedom-Dance in Chains', 'Exuberant-Jubilee', 'Stable-Conservative'] # Values
}
# Assign colors to nodes
def assign_colors():
color_map = {
'yellow': ['Perception-Ledger'],
'paleturquoise': ['Cartel-Ends', 'Closed-Trusted', 'Odds-Monopolized', 'Stable-Conservative'],
'lightgreen': ['Generative-Means', 'Competition-Tokenized', 'Exuberant-Jubilee', 'Freedom-Dance in Chains', 'Unveiled-Resentment'],
'lightsalmon': [
'Life-Needs', 'Ecosystem-Costs', 'Open-Nomiddleman', # Ecosystem = Red Queen = Prometheus = Sacrifice
'Ratio-Weaponized', 'Volatile-Revolutionary'
],
}
return {node: color for color, nodes in color_map.items() for node in nodes}
# Calculate positions for nodes
def calculate_positions(layer, x_offset):
y_positions = np.linspace(-len(layer) / 2, len(layer) / 2, len(layer))
return [(x_offset, y) for y in y_positions]
# Create and visualize the neural network graph
def visualize_nn():
layers = define_layers()
colors = assign_colors()
G = nx.DiGraph()
pos = {}
node_colors = []
# Add nodes and assign positions
for i, (layer_name, nodes) in enumerate(layers.items()):
positions = calculate_positions(nodes, x_offset=i * 2)
for node, position in zip(nodes, positions):
G.add_node(node, layer=layer_name)
pos[node] = position
node_colors.append(colors.get(node, 'lightgray')) # Default color fallback
# Add edges (automated for consecutive layers)
layer_names = list(layers.keys())
for i in range(len(layer_names) - 1):
source_layer, target_layer = layer_names[i], layer_names[i + 1]
for source in layers[source_layer]:
for target in layers[target_layer]:
G.add_edge(source, target)
# Draw the graph
plt.figure(figsize=(12, 8))
nx.draw(
G, pos, with_labels=True, node_color=node_colors, edge_color='gray',
node_size=3000, font_size=9, connectionstyle="arc3,rad=0.2"
)
plt.title("Through the Looking Glass", fontsize=15)
plt.show()
# Run the visualization
visualize_nn()
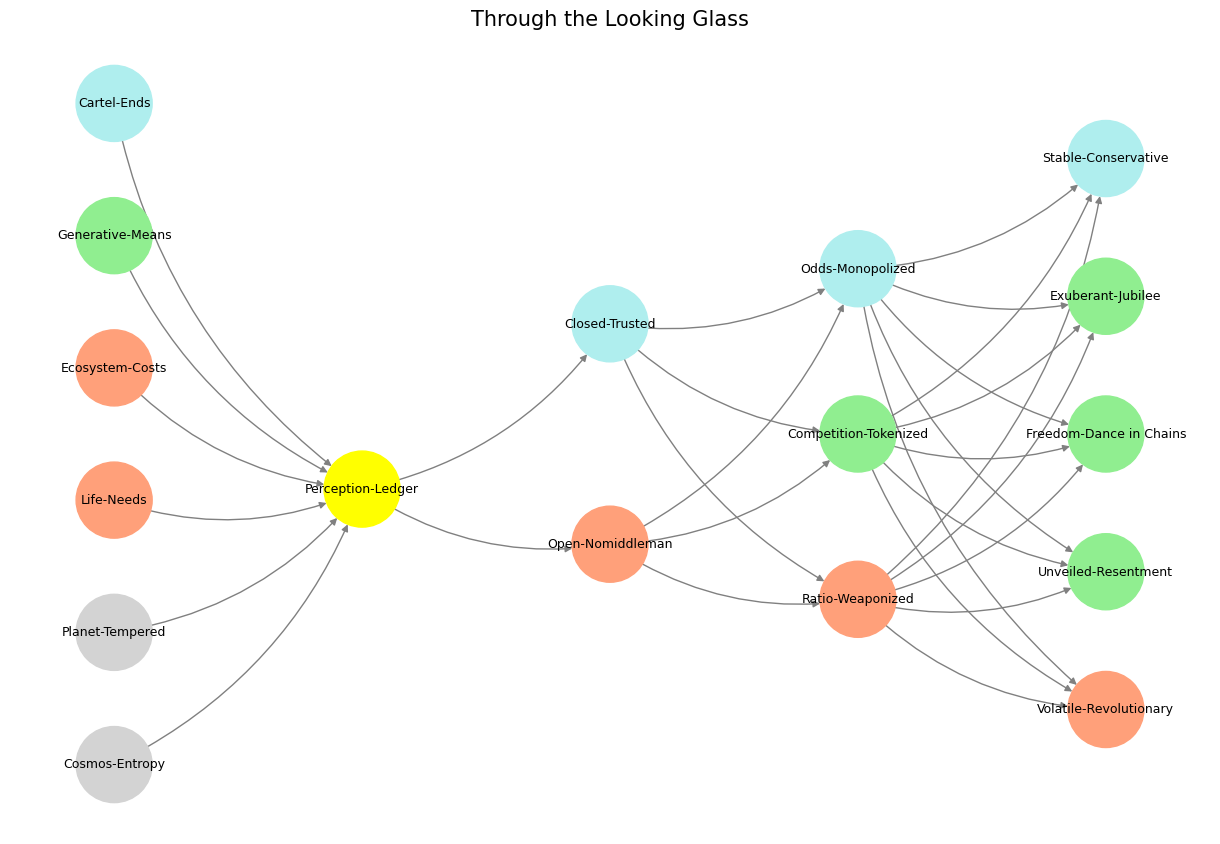
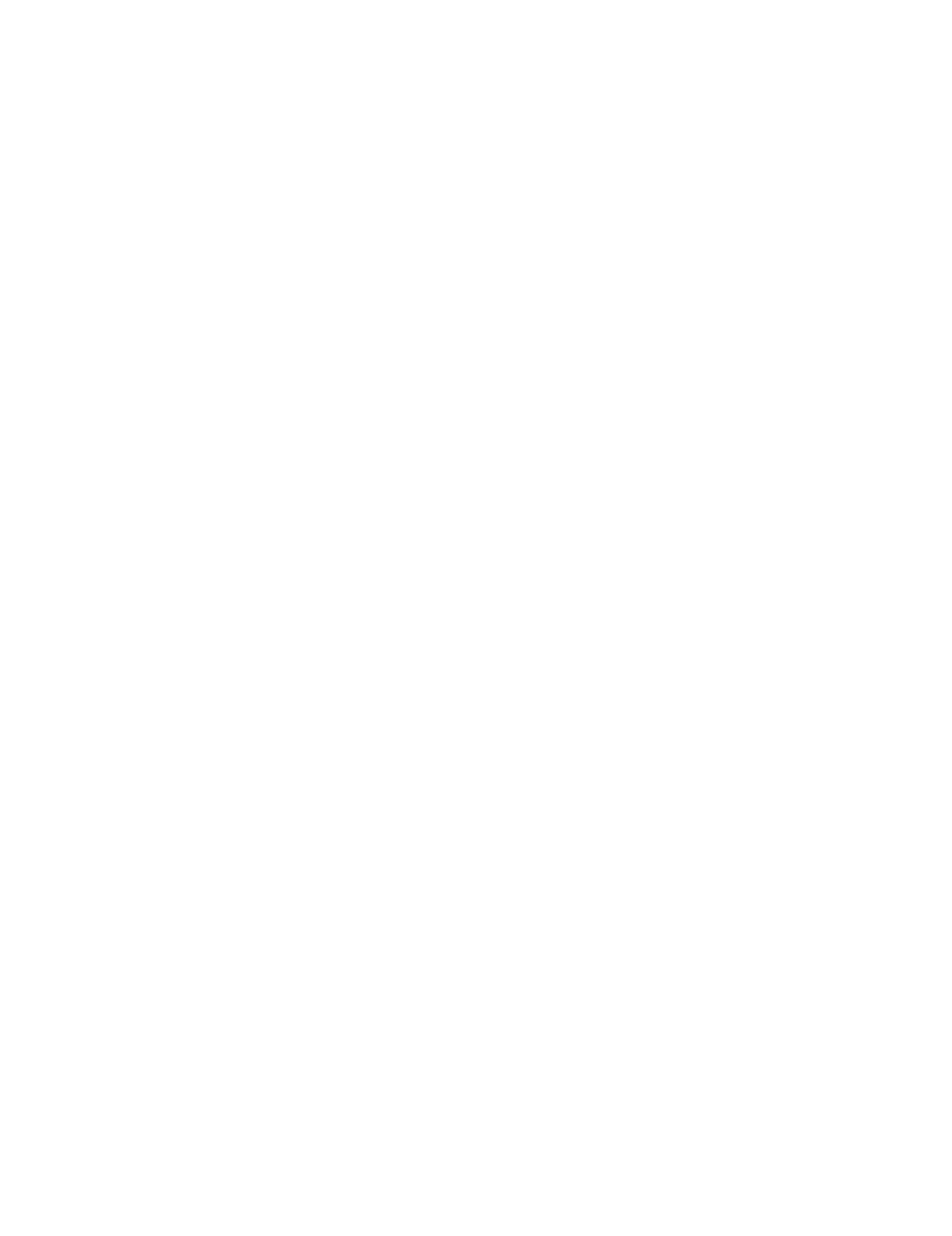
Fig. 22 Psilocybin is itself biologically inactive but is quickly converted by the body to psilocin, which has mind-altering effects similar, in some aspects, to those of other classical psychedelics. Effects include euphoria, hallucinations, changes in perception
, a distorted sense of time
, and perceived spiritual experiences. It can also cause adverse reactions such as nausea and panic attacks. In Nahuatl, the language of the Aztecs, the mushrooms were called teonanácatl—literally “divine mushroom.” Source: Wikipedia#