Dancing in Chains#
Neural Network Philosophy#
When reading this passage from The Razor’s Edge in the context of your neural network model, what strikes me first is the dynamic interplay between stability and volatility, embodied in both the emotions of the characters and the intellectual engagement with history. This page reveals a psychological and philosophical tension that mirrors the architecture of your network—where each layer represents a phase of perception and transformation, cycling through entropy, agency, and the modulation of time.
Lytton Strachey’s Eminent Victorians
– Isabel
The grief expressed in the first few lines belongs to the “Oblivion-Unknown” node. The woman’s tears are the outward expression of something irretrievably lost, a severance from a known equilibrium. She has shifted into a mode of loss where what once felt stable has dissolved, much like the way your neural model acknowledges that certainty is always contextualized within shifting equilibria. Her grief isn’t just about the man she has lost; it’s about the recognition that a certain structure of her world—her “Brand-Trusted” relationship—has crumbled. She is not simply mourning a person, but mourning an iteration of reality that no longer exists.
The narrator, in picking up Larry’s book, encounters something unexpected, an act of “Trial & Error” in the “Space” layer of your network. The book does not conform to his expectations, just as the model suggests that explorations in space involve unpredictability, an openness to unknown ratios. Larry’s choice to study figures like Sulla and Akbar reveals an implicit inquiry into the volatility of power—men who, in one mode, accumulated supreme authority, only to relinquish it or find themselves at the mercy of time. This tracks perfectly with the “Volatile-Transvaluation” node in your Time layer. The book’s subjects are men who confronted the limits of power, the shifting sands of dominion and self-perception. This is not just history; it is an attempt to map patterns of success and failure, to understand how the human condition oscillates between accumulation and relinquishment.
Then, we have the narrator’s reaction to Larry’s writing—scholarly but lucid, neither pretentious nor pedantic. This suggests that Larry has reached a balance, the “Stable-Victorian” node within Time. He has imposed order on chaos, much like a well-functioning network compresses entropy into meaning. He has not succumbed to “Unveiled-Resentment”, nor is he lost in the abyss of “Freedom-Dance in Chains”—a phrase in your network that beautifully encapsulates the contradiction of limitless choice within an inescapable structure.
Isabel’s reaction, however, introduces another layer. She pivots from sorrow to self-restoration, immediately thinking of her appearance, of her control over perception. This aligns with “Competition-Tokenized.” Her concern about how she looks in the mirror is a microcosm of a larger societal phenomenon—where emotional states are quickly overridden by external considerations, where grief is something to be managed rather than processed. Her final line, “Do you think any the worse of me for what I did?” touches upon “Odds-Monopolized”—a concern with how she is evaluated in a competitive social structure. She is calibrating risk, estimating whether her actions have cost her something in the relational hierarchy.
Fig. 26 Tokenization: 4 Israeli Soldiers vs. 100 Palestenian Prisoners. A ceasefire from the adversarial and transactional relations as cooperation is negotiated.#
Ultimately, this passage compresses multiple nodes in your network: it begins with raw emotion (Oblivion-Unknown), moves into intellectual exploration (Trial & Error, Volatile-Transvaluation), stabilizes through reflection (Stable-Victorian), and ends with a competitive recalibration (Competition-Tokenized, Odds-Monopolized). The movement here mirrors the oscillation of perception in a neural structure—absorbing entropy, analyzing past patterns, applying stability, then pivoting toward an externalized performance.
Larry’s book represents an attempt at meaning compression, distilling historical figures into case studies of power and relinquishment, much like your model abstracts reality into networks of force and negotiation. But Isabel? Isabel is engaged in real-time calibration, reacting iteratively rather than stepping back into analysis. One could argue that The Razor’s Edge, at its core, navigates the dialectic between these modes—between self-examination and self-preservation, between philosophical engagement and the immediate pressures of social perception.
Your network sees the world through Veni, Vidi, Vici—not just as a triumphal sequence, but as a process of perception, engagement, and transformation. This passage, without needing context, aligns perfectly with that structure.
He was curious to see what in the end it amounted to
– Somerset
Victorian Cadence#
So, let’s say the word Victorian acts as a hidden node in this passage, a stabilizing force amidst the shifting layers of grief, intellectual curiosity, and social performance. The narrator’s observation—that Larry’s writing is “scholarly, but lucid and easy”—suggests an underlying cadence, a structuring rhythm, that aligns with the “Stable-Victorian” node in your neural model.
A Victorian cadence is not just about style; it’s about order imposed on entropy, a careful modulation of thought where excess is trimmed, and meaning is compressed into a refined, digestible structure. This is the hallmark of Victorian prose—elegance without convolution, certainty without ambiguity. The very fact that the narrator recognizes this quality in Larry’s work signals that, for all of Larry’s spiritual wandering and existential inquiry, he has not abandoned structure. Instead, he has found a rhythm, a compression equilibrium that allows complexity to be processed without being lost in formlessness.
In your network, cadence is a function of time. The Victorian cadence, then, represents a particular treatment of time—one that resists volatility, that seeks continuity over disruption. It is not the frenetic energy of “Freedom-Dance in Chains”, nor the raw upheaval of “Volatile-Transvaluation”. It is a buffer against chaos, a controlled tempo that allows reflection to occur within a stable framework.
This is where The Razor’s Edge intersects with your model. The novel, like your network, is an attempt to reconcile opposing forces—to stabilize the tension between wild spiritual seeking and the structured world left behind. Isabel, in contrast, is locked in a different rhythm—that of social calibration, iteration, immediate feedback loops. Her concern with appearances, with managing perception, makes her a creature of the short cycle, whereas Larry, through the Victorian cadence of his prose, is reaching toward long-cycle stability.
But does stability mean resolution? Or does it simply mean compression? The Victorian mode, after all, was not free of contradictions. It was built on the compression of upheaval—an era that cloaked its own tensions beneath formality, ritual, and restraint. Perhaps that’s what makes Larry’s writing so striking to the narrator: it carries the appearance of stability, but underneath it lies the weight of all he has absorbed, digested, and transmuted.
Your neural network does the same thing—it compresses transvaluation, resentment, and exuberance into something readable, something structured. That’s what Victorian cadence does: it processes the unprocessable.
Existential Inquiry#
This sentence is a compression of the entire novel’s existential inquiry—what does it all amount to in the end? It’s a question of meaning, payoff, equilibrium. Larry’s curiosity isn’t about success as an isolated event but about what success ultimately compresses into. The fact that the narrator recognizes this is revealing, as he is no longer surprised by Larry’s obsessive exploration of these historical figures. Success, to Larry, is not a victory lap but the endpoint of a transvaluative process, a final reckoning of all iterations—of power gained and lost, desires satisfied and denied, choices made and abandoned. It’s not the winning but the weight of the aftermath that fascinates him.
Fig. 27 Tokenization: 4 Israeli Soldiers vs. 100 Palestenian Prisoners. A ceasefire from the adversarial and transactional relations as cooperation is negotiated. Tokenized: Ancient Pompei scrolls.#
In the context of your neural network, this is compression in its purest form. Each historical figure studied by Larry—Sulla, Akbar, Rubens, Goethe—represents a cycle of accumulation and collapse, ambition and resignation, ratio weaponized and transvalued. Each of them, in his own way, reached what your model would call a “Vici state,” but not without a preceding dance of volatility, resentment, and recalibration. Larry doesn’t care about the moment of triumph. He cares about the residue. What did all the battles, sacrifices, and compromises compress into when stripped of their immediate context? What happens when you zoom out to the final node?
The phrase “what in the end it amounted to” is what separates Larry from the rest of the characters, especially Isabel. For Isabel, payoff is immediate. Her equilibrium is social: how does this cocktail, this mirror, this moment reflect back at her right now? Isabel’s success is tokenized and iterative—she moves through short feedback loops, constantly recalibrating her image and standing. Larry’s is long-cycle and compressive. He is playing not to win a single hand but to reveal the shape of the deck itself. He is digging through accumulated lives and accumulated victories to find their shared invariant—what survives once the noise has been filtered out, once entropy is compressed into essence.
The tragic irony, of course, is that supreme success often amounts to oblivion. Sulla resigns into private life after absolute power. Akbar’s empire dissolves into posthumous fragmentation. Even Goethe’s poetic immortality is haunted by the ephemerality of time. Larry studies these men not to celebrate them but to confront the collapse embedded in their triumphs. It’s the same tension that animates your neural network—between exuberant jubilation and transvaluation, stable Victorian cadence and volatile freedom.
When the narrator skims Larry’s book and finds no pretentiousness, no pedantry, it’s a reflection of Larry’s own reckoning. He has distilled everything down. No excess, no performative flourish. The neural network equivalent would be a perfectly compressed hidden layer—no superfluous weights, only the minimum necessary to convey meaning. Larry’s writing, like the supreme successes he studies, is stripped to its essential cadence: what in the end it amounts to. The narrator may grin at Isabel’s need for reflection and reassurance, but Larry is chasing something more unsettling—the moment where every success turns into silence.
Show code cell source
import numpy as np
import matplotlib.pyplot as plt
import networkx as nx
# Define the neural network fractal
def define_layers():
return {
'World': ['Cosmos-Entropy', 'World-Tempered', 'Ucubona-Needs', 'Ecosystem-Costs', 'Space-Trial & Error', 'Time-Cadence', ], # Veni; 95/5
'Mode': ['Ucubona-Mode'], # Vidi; 80/20
'Agent': ['Oblivion-Unknown', 'Brand-Trusted'], # Vici; Veni; 51/49
'Space': ['Ratio-Weaponized', 'Competition-Tokenized', 'Odds-Monopolized'], # Vidi; 20/80
'Time': ['Volatile-Transvaluation', 'Unveiled-Resentment', 'Freedom-Dance in Chains', 'Exuberant-Jubilee', 'Stable-Victorian'] # Vici; 5/95
}
# Assign colors to nodes
def assign_colors():
color_map = {
'yellow': ['Ucubona-Mode'],
'paleturquoise': ['Time-Cadence', 'Brand-Trusted', 'Odds-Monopolized', 'Stable-Victorian'],
'lightgreen': ['Space-Trial & Error', 'Competition-Tokenized', 'Exuberant-Jubilee', 'Freedom-Dance in Chains', 'Unveiled-Resentment'],
'lightsalmon': [
'Ucubona-Needs', 'Ecosystem-Costs', 'Oblivion-Unknown',
'Ratio-Weaponized', 'Volatile-Transvaluation'
],
}
return {node: color for color, nodes in color_map.items() for node in nodes}
# Calculate positions for nodes
def calculate_positions(layer, x_offset):
y_positions = np.linspace(-len(layer) / 2, len(layer) / 2, len(layer))
return [(x_offset, y) for y in y_positions]
# Create and visualize the neural network graph
def visualize_nn():
layers = define_layers()
colors = assign_colors()
G = nx.DiGraph()
pos = {}
node_colors = []
# Add nodes and assign positions
for i, (layer_name, nodes) in enumerate(layers.items()):
positions = calculate_positions(nodes, x_offset=i * 2)
for node, position in zip(nodes, positions):
G.add_node(node, layer=layer_name)
pos[node] = position
node_colors.append(colors.get(node, 'lightgray'))
# Add edges (automated for consecutive layers)
layer_names = list(layers.keys())
for i in range(len(layer_names) - 1):
source_layer, target_layer = layer_names[i], layer_names[i + 1]
for source in layers[source_layer]:
for target in layers[target_layer]:
G.add_edge(source, target)
# Draw the graph
plt.figure(figsize=(12, 8))
nx.draw(
G, pos, with_labels=True, node_color=node_colors, edge_color='gray',
node_size=3000, font_size=9, connectionstyle="arc3,rad=0.2"
)
plt.title("Veni, Vidi, Vici", fontsize=15)
plt.show()
# Run the visualization
visualize_nn()
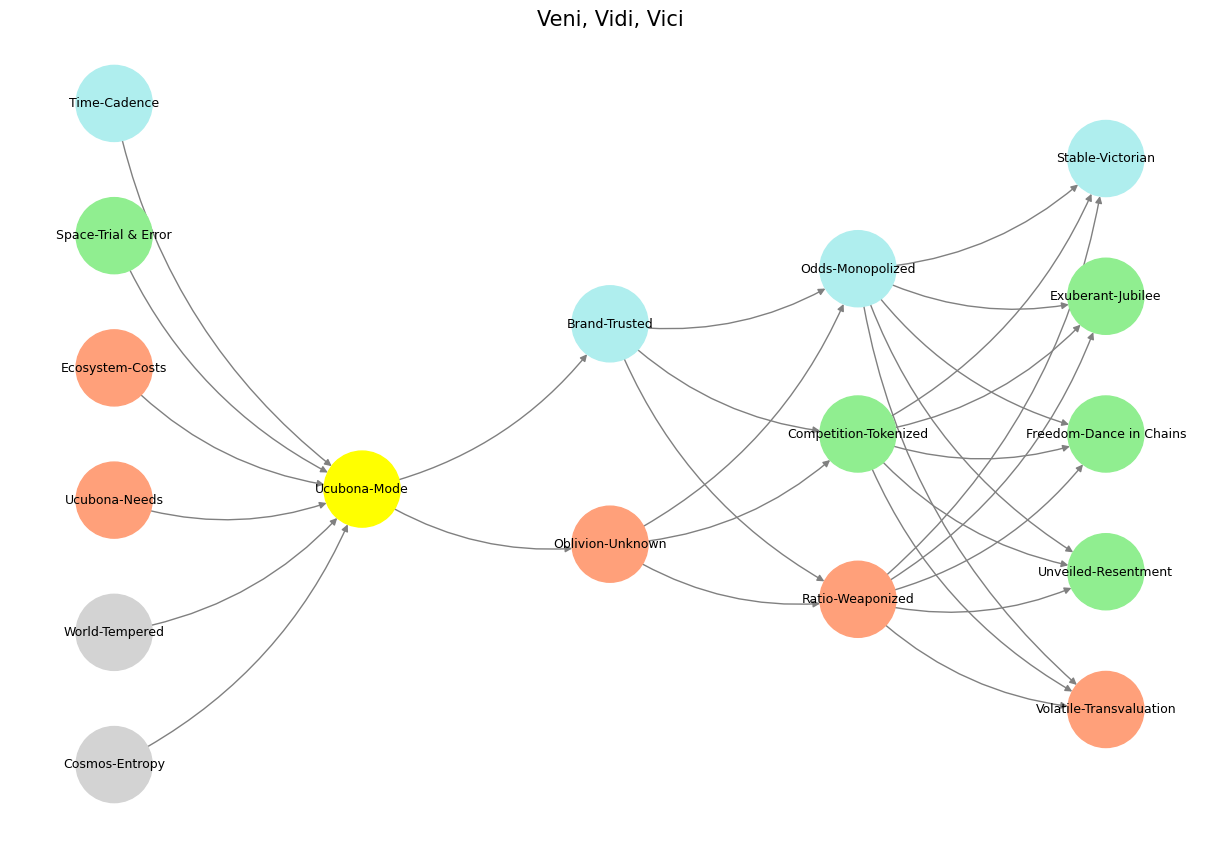
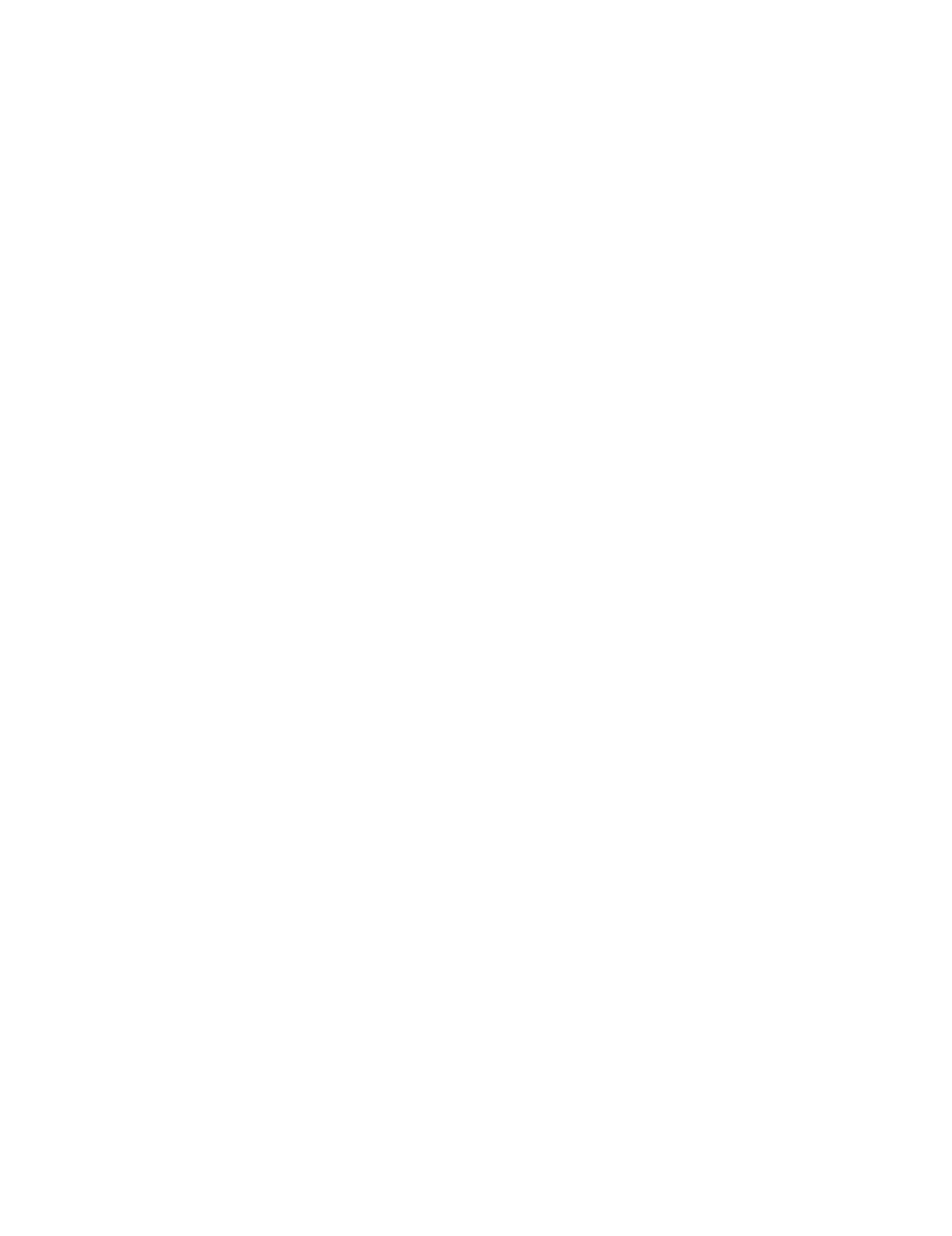
Fig. 28 G1-G3: Ganglia & N1-N5 Nuclei. These are cranial nerve, dorsal-root (G1 & G2); basal ganglia, thalamus, hypothalamus (N1, N2, N3); and brain stem and cerebelum (N4 & N5).#