Resilience 🗡️❤️💰#
Let’s embark on another playful yet probing essay, this time juxtaposing our immunoneural “strategic bequest motive”—that intricate dance of DNA replication, mismatch repair, and immune-nervous system interplay—with the rather more terrestrial saga of “Project Family Harmony,” Rupert Murdoch’s ill-fated attempt to secure his media empire’s succession. Our layer 4 framework, with lightsalmon’s sympathetic adversarial flare, paleturquoise’s parasympathetic cooperative hum, and lightgreen’s transactional toggling, offers a lens to compare these two. One’s a biological blueprint honed by eons; the other’s a human gambit that crumbled under the weight of ego and entropy. Perhaps old Rupert could’ve gleaned a lesson or two from immunology’s playbook—after all, both systems wrestle with legacy, fidelity, and the perils of misrecognition.

Fig. 12 Influencer (Witness) vs. Indicator (Trial). These reflects sentiments in 2 Chronicles 17:9-12
: For the eyes of the Lord run to and fro throughout the whole earth, to show Himself strong (Nowamaani) on behalf of them whose heart is perfect toward Him. This parallels Shakespeare’s image of the poet’s eye “in a fine frenzy rolling,” scanning from heaven to earth and back. Ukubona beyond the mundane (network layers 3-5), upstream to first prinicples of the ecosystem (layer 1). This is the duty of intelligence and what our App and its variants in and beyond clinical medicine aims for – to elevate perception, agency, and games for all. To leave a shrinking marketplace for the serpent in Eden, for snakeoil salesmen, for fraudstars. To shrink the number of the gullible.#
In our immunoneural model, the “strategic bequest motive” emerges from DNA’s drive to replicate and repair, a legacy-preserving mechanism where mismatch repair (MMR) ensures the ancestral code’s integrity. Lightsalmon kicks in when errors persist—PD-1 signaling a shift to “fight” against non-self threats, an adversarial stance to protect the lineage. Paleturquoise soothes when repair succeeds, fostering a cooperative “breed and feed” state, while lightgreen’s presynaptic ganglion transacts between the two, balancing self-preservation with adaptation via PCNA’s molecular handshakes. It’s a system obsessed with fidelity yet flexible enough to negotiate errors, a bequest motive that’s strategic because it anticipates failure and plans for correction. The immune system, twinned with the nervous, doesn’t just pass down genes—it bequeaths a dynamic equilibrium, a living trust that evolves with each generation.
Self, Nonself, Preservation
– SNPs
Contrast this with “Project Family Harmony,” Murdoch’s attempt to orchestrate his children’s inheritance of News Corp and Fox, a media dynasty built on ink and airwaves rather than nucleotides. Conceived in the late 2000s, it aimed to lock in equal voting rights for his four eldest kids—Prudence, Elisabeth, Lachlan, and James—via a trust structure, ensuring a cooperative legacy akin to paleturquoise’s calm. But Murdoch’s empire wasn’t a closed system like DNA’s helix; it was a battlefield of personalities and power plays. The adversarial lightsalmon flared as Lachlan’s primacy clashed with James’s dissent, and the transactional lightgreen—meant to mediate through legal frameworks and boardroom deals—faltered under shifting alliances and Rupert’s own meddling. By 2023, with Lachlan ascendant and James sidelined, the project lay in tatters, a bequest motive less strategic than stubbornly autocratic.
Here’s where immunology might’ve whispered some wisdom to Murdoch. In MMR, strand-specific repair hinges on recognizing the parental template versus the error-prone daughter strand—think hemimethylation or nicks as markers. Murdoch, though, blurred his own template, rewriting the trust’s rules in 2024 to favor Lachlan, undermining the original cooperative intent. Immunology knows misrecognition spells doom—failure to distinguish self from non-self triggers PD-1’s adversarial spiral. Rupert’s refusal to let the system self-correct, to let his heirs negotiate their own equilibrium, mirrors a breakdown in mismatch repair: instead of fixing errors, he amplified them, turning family into foe. The transactional lightgreen of PCNA, loading precisely to guide repair, finds no echo in Murdoch’s clumsy legal maneuvers—where the immune system adapts, he dictated.
The deeper parallel lies in legacy’s fragility. DNA’s bequest motive thrives because it’s decentralized—every cell carries the code, every repair a local act of fidelity. Murdoch’s centralized control, clinging to his own 3’-OH end like a patriarch afraid to let go, choked the system’s ability to toggle dynamically. Immunology teaches that harmony isn’t imposed; it’s emergent, a balance of adversarial and cooperative forces mediated by clear rules. “Project Family Harmony” lacked the strand-specific clarity of MMR—no nicks to mark the way, no PCNA to direct the next move. Rupert might’ve studied how PD-1 shifts from self to non-self, recognizing when to fight and when to yield. Instead, he fought everyone, yielding nothing, and the harmony he sought became a cacophony of lawsuits and estrangement.
So, perhaps Murdoch should’ve cracked open an immunology text. The strategic bequest motive of our immunoneural map—lightsalmon’s vigilance, paleturquoise’s nurture, lightgreen’s mediation—offers a masterclass in legacy that’s both robust and resilient. DNA doesn’t micromanage; it sets the stage and trusts the process. Murdoch’s project, by contrast, was a lesson in how not to bequeath: a top-down fantasy of control that ignored the biology of systems, human or otherwise. In the end, immunology’s dance of equilibrium outshines Rupert’s stumble—proof that nature’s strategies, prankishly mapped or not, hold secrets even a media titan could’ve used.
Show code cell source
import numpy as np
import matplotlib.pyplot as plt
import networkx as nx
# Define the neural network layers
def define_layers():
return {
'Suis': ['DNA, RNA, 5%', 'Peptidoglycans, Lipoteichoics', 'Lipopolysaccharide', 'N-Formylmethionine', "Glucans, Chitin", 'Specific Antigens'],
'Voir': ['PRR & ILCs, 20%'],
'Choisis': ['CD8+, 50%', 'CD4+'],
'Deviens': ['TNF-α, IL-6, IFN-γ', 'PD-1 & CTLA-4', 'Tregs, IL-10, TGF-β, 20%'],
"M'èléve": ['Complement System', 'Platelet System', 'Granulocyte System', 'Innate Lymphoid Cells, 5%', 'Adaptive Lymphoid Cells']
}
# Assign colors to nodes
def assign_colors():
color_map = {
'yellow': ['PRR & ILCs, 20%'],
'paleturquoise': ['Specific Antigens', 'CD4+', 'Tregs, IL-10, TGF-β, 20%', 'Adaptive Lymphoid Cells'],
'lightgreen': ["Glucans, Chitin", 'PD-1 & CTLA-4', 'Platelet System', 'Innate Lymphoid Cells, 5%', 'Granulocyte System'],
'lightsalmon': ['Lipopolysaccharide', 'N-Formylmethionine', 'CD8+, 50%', 'TNF-α, IL-6, IFN-γ', 'Complement System'],
}
return {node: color for color, nodes in color_map.items() for node in nodes}
# Define edge weights
def define_edges():
return {
('DNA, RNA, 5%', 'PRR & ILCs, 20%'): '1/99',
('Peptidoglycans, Lipoteichoics', 'PRR & ILCs, 20%'): '5/95',
('Lipopolysaccharide', 'PRR & ILCs, 20%'): '20/80',
('N-Formylmethionine', 'PRR & ILCs, 20%'): '51/49',
("Glucans, Chitin", 'PRR & ILCs, 20%'): '80/20',
('Specific Antigens', 'PRR & ILCs, 20%'): '95/5',
('PRR & ILCs, 20%', 'CD8+, 50%'): '20/80',
('PRR & ILCs, 20%', 'CD4+'): '80/20',
('CD8+, 50%', 'TNF-α, IL-6, IFN-γ'): '49/51',
('CD8+, 50%', 'PD-1 & CTLA-4'): '80/20',
('CD8+, 50%', 'Tregs, IL-10, TGF-β, 20%'): '95/5',
('CD4+', 'TNF-α, IL-6, IFN-γ'): '5/95',
('CD4+', 'PD-1 & CTLA-4'): '20/80',
('CD4+', 'Tregs, IL-10, TGF-β, 20%'): '51/49',
('TNF-α, IL-6, IFN-γ', 'Complement System'): '80/20',
('TNF-α, IL-6, IFN-γ', 'Platelet System'): '85/15',
('TNF-α, IL-6, IFN-γ', 'Granulocyte System'): '90/10',
('TNF-α, IL-6, IFN-γ', 'Innate Lymphoid Cells, 5%'): '95/5',
('TNF-α, IL-6, IFN-γ', 'Adaptive Lymphoid Cells'): '99/1',
('PD-1 & CTLA-4', 'Complement System'): '1/9',
('PD-1 & CTLA-4', 'Platelet System'): '1/8',
('PD-1 & CTLA-4', 'Granulocyte System'): '1/7',
('PD-1 & CTLA-4', 'Innate Lymphoid Cells, 5%'): '1/6',
('PD-1 & CTLA-4', 'Adaptive Lymphoid Cells'): '1/5',
('Tregs, IL-10, TGF-β, 20%', 'Complement System'): '1/99',
('Tregs, IL-10, TGF-β, 20%', 'Platelet System'): '5/95',
('Tregs, IL-10, TGF-β, 20%', 'Granulocyte System'): '10/90',
('Tregs, IL-10, TGF-β, 20%', 'Innate Lymphoid Cells, 5%'): '15/85',
('Tregs, IL-10, TGF-β, 20%', 'Adaptive Lymphoid Cells'): '20/80'
}
# Define edges to be highlighted in black
def define_black_edges():
return {
('Tregs, IL-10, TGF-β, 20%', 'Complement System'): '1/99',
('Tregs, IL-10, TGF-β, 20%', 'Platelet System'): '5/95',
('Tregs, IL-10, TGF-β, 20%', 'Granulocyte System'): '10/90',
('Tregs, IL-10, TGF-β, 20%', 'Innate Lymphoid Cells, 5%'): '15/85',
('Tregs, IL-10, TGF-β, 20%', 'Adaptive Lymphoid Cells'): '20/80'
}
# Calculate node positions
def calculate_positions(layer, x_offset):
y_positions = np.linspace(-len(layer) / 2, len(layer) / 2, len(layer))
return [(x_offset, y) for y in y_positions]
# Create and visualize the neural network graph
def visualize_nn():
layers = define_layers()
colors = assign_colors()
edges = define_edges()
black_edges = define_black_edges()
G = nx.DiGraph()
pos = {}
node_colors = []
# Create mapping from original node names to numbered labels
mapping = {}
counter = 1
for layer in layers.values():
for node in layer:
mapping[node] = f"{counter}. {node}"
counter += 1
# Add nodes with new numbered labels and assign positions
for i, (layer_name, nodes) in enumerate(layers.items()):
positions = calculate_positions(nodes, x_offset=i * 2)
for node, position in zip(nodes, positions):
new_node = mapping[node]
G.add_node(new_node, layer=layer_name)
pos[new_node] = position
node_colors.append(colors.get(node, 'lightgray'))
# Add edges with updated node labels
edge_colors = []
for (source, target), weight in edges.items():
if source in mapping and target in mapping:
new_source = mapping[source]
new_target = mapping[target]
G.add_edge(new_source, new_target, weight=weight)
edge_colors.append('black' if (source, target) in black_edges else 'lightgrey')
# Draw the graph
plt.figure(figsize=(12, 8))
edges_labels = {(u, v): d["weight"] for u, v, d in G.edges(data=True)}
nx.draw(
G, pos, with_labels=True, node_color=node_colors, edge_color=edge_colors,
node_size=3000, font_size=9, connectionstyle="arc3,rad=0.2"
)
nx.draw_networkx_edge_labels(G, pos, edge_labels=edges_labels, font_size=8)
plt.title("OPRAH™: Default-Mode Network", fontsize=18)
plt.show()
# Run the visualization
visualize_nn()
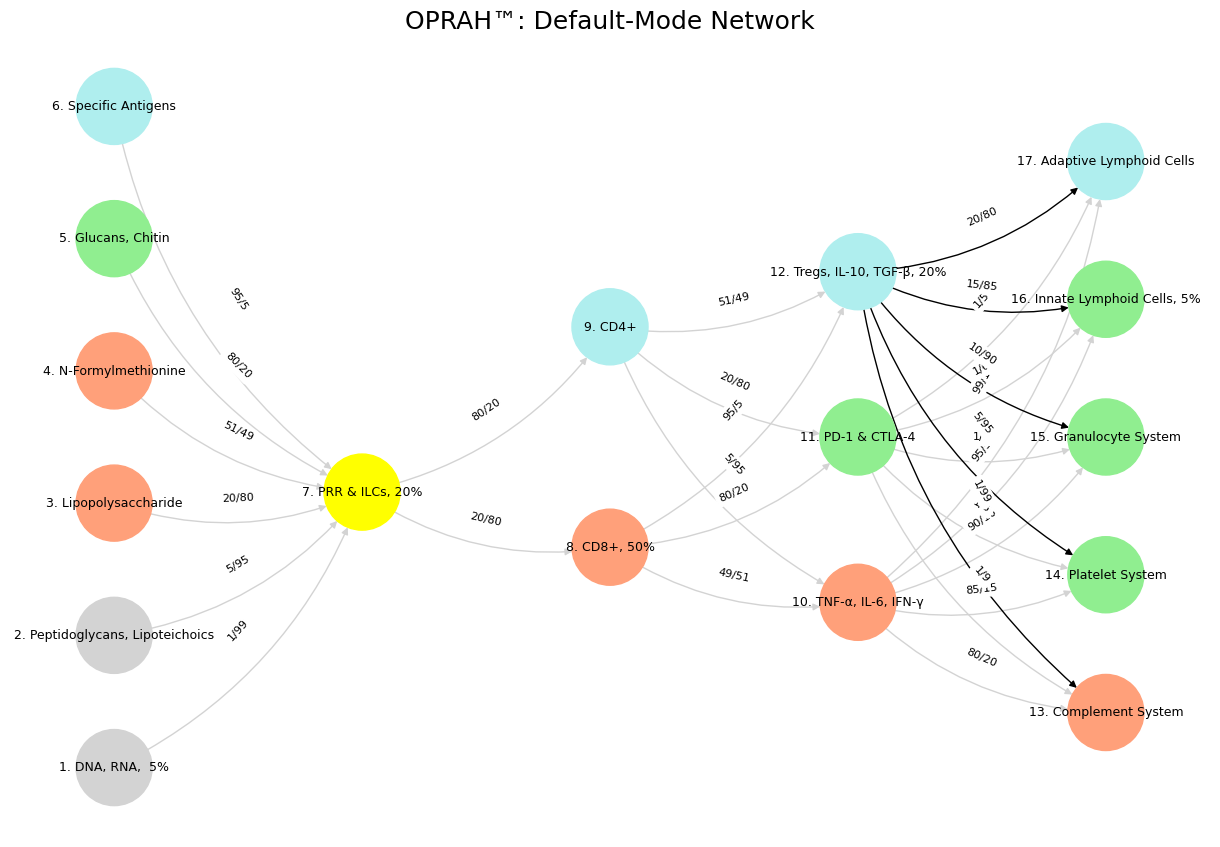
Fig. 13 Resources, Needs, Costs, Means, Ends. This is an updated version of the script with annotations tying the neural network layers, colors, and nodes to specific moments in Vita è Bella, enhancing the connection to the film’s narrative and themes:#