Orient#
Uncovered Inefficiencies#
Reimagining Research Workflows: A Framework for Efficiency, Accessibility, and Generativity#
In my doctoral journey, I have uncovered inefficiencies in research workflows that impede the speed and accessibility of discovery in academic medicine. These inefficiencies, often deeply rooted in the structural and procedural norms of academia, present barriers that I believe are solvable with the right tools and mindset. To address these challenges, I have developed an app and a framework that streamline the flow of information from raw datasets to actionable insights. This system is not merely a critique of the processes that currently govern researchâit is a constructive solution designed to empower researchers, patients, and institutions alike.
A Network of Inputs and Barriers#
At the input layer of my framework lies the foundational data sources upon which research depends: patient records, public health databases, and cohort studies. These datasets, often generated for regulatory or clinical purposes, are crucial to advancing knowledge. Yet, accessing them is fraught with challenges. Guardians of these datasets impose regulatory barriers, requiring researchers to navigate intricate Institutional Review Board (IRB) processes and gain permissions that are both time-consuming and redundant. Even after gaining access, researchers face technical hurdles in linking disparate datasets, particularly when combining national registries like the US Renal Data System (USRDS) with local electronic health records. These barriers create an environment where the most time-consuming aspects of research are logistical rather than intellectual.
The app I have developed addresses these barriers directly. It provides researchers with pre-built scripts in R or Python, which can be run by collaborators who already possess IRB approval. This approach eliminates the need for redundant IRB applications while ensuring regulatory compliance. The workflow automates the extraction of essential data pointsâsuch as beta coefficients, variance-covariance matrices, and Kaplan-Meier survival estimatesâand integrates them into interactive visualizations. By offering a streamlined pathway from raw data to insight, my framework reweights the inefficiencies of the current system toward generative outputs.
Iterative Pathways for Collaboration and Utility#
The heart of my framework lies in its green nodesâthose iterative pathways that emphasize collaboration and utility without direct confrontation. This approach reframes my critique of the system as an act of enabling rather than opposing. The app is not a rejection of existing workflows but an amplification of their potential. It allows researchers to focus on what truly matters: generating meaningful insights and improving patient outcomes.
For example, my app facilitates faster access to data by enabling linked datasets to be analyzed efficiently. The pre-built scripts reduce the technical expertise required to handle complex data structures, democratizing access to advanced statistical techniques. Interactive visualizations allow not only researchers but also patients, students, and policymakers to engage with the data, fostering a more inclusive approach to knowledge dissemination. These green-node pathways ensure that my critique is generative, creating new possibilities for collaboration and utility.
Respecting the System While Challenging Its Inefficiencies#
While my framework critiques the inefficiencies of the current system, it does so with respect for the foundational structures that underpin academic research. The regulatory oversight of IRBs, the meticulous curation of national datasets, and the rigor of peer review are all invaluable components of the research ecosystem. However, these structures must evolve to meet the demands of a faster, more interconnected world. My framework positions itself as a tool to complement and enhance these systems, reducing their burdens while preserving their integrity.
To this end, I emphasize the collaborative utility of my app. By providing a workflow that integrates seamlessly with existing systems, it reduces the workload for IRB reviewers, dataset guardians, and researchers. It also respects the systemâs goals by maintaining compliance and transparency at every step. This approach allows my critique to be constructive, focusing on opportunities for improvement rather than dwelling on shortcomings.
A Black Box of Mechanisms and Outcomes#
One of the critical insights from my framework is the acknowledgment of gaps in our understanding of mechanisms. While datasets provide a wealth of information on outcomesâsuch as 90-day perioperative mortality, 30-year kidney failure risk, and long-term hospitalization ratesâthey often lack data on the mechanisms driving these outcomes. This âblack boxâ of mechanisms highlights the limitations of current research workflows. My framework does not claim to solve this issue but provides a structure for better navigating it. By streamlining the flow of information to the yellow node, where clinical scenarios are juxtaposed with counterfactuals, my app creates a platform for exploring these gaps more effectively.
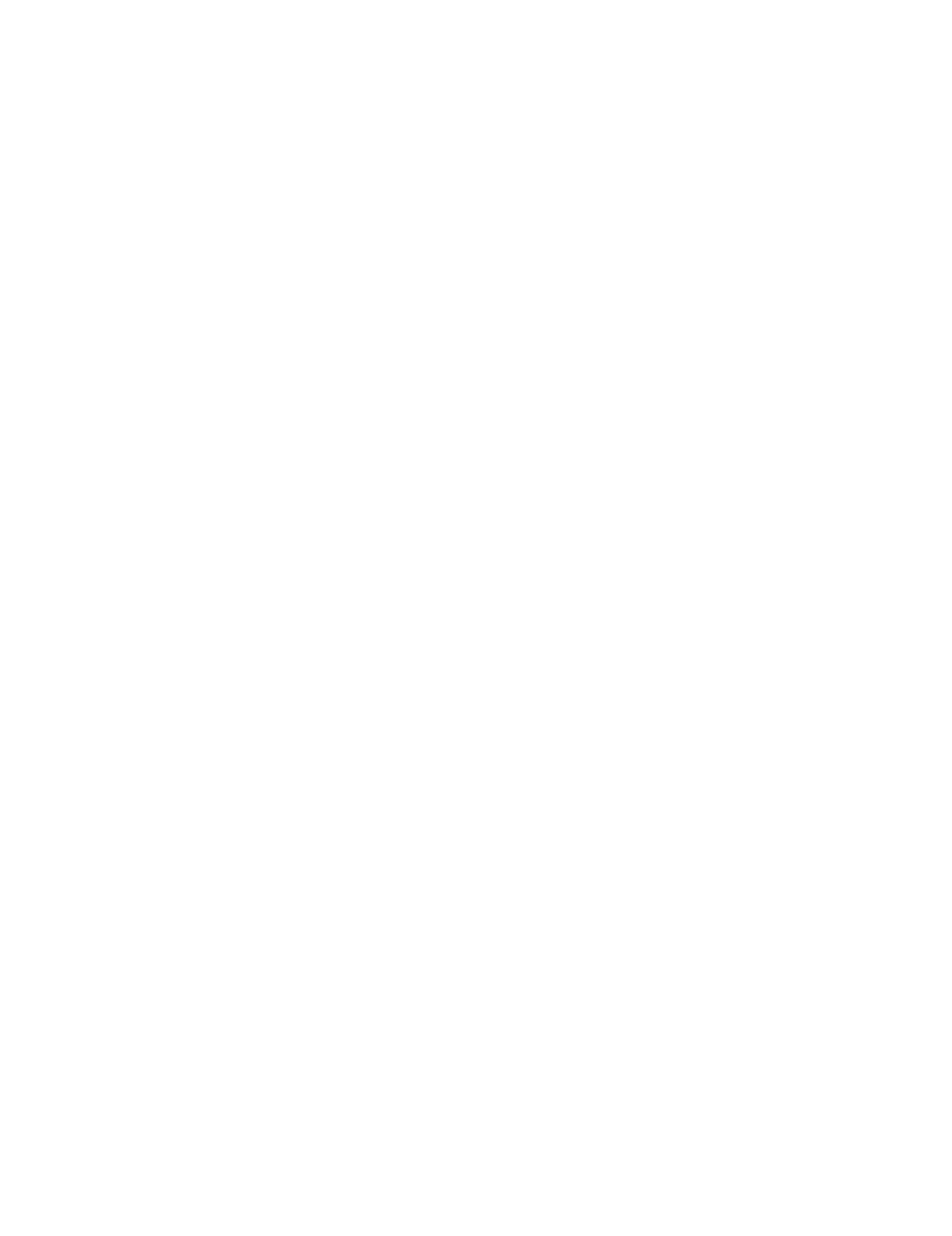
Fig. 30 Letâs have some sake for old times sake! And lets reflect upon our legacy as one might through our sons! This is a subtle issue unlikely to be captured by most cohorts of older individuals. The meaning of life in the twilight, what one optimized in earlier years, what the costs were, and what the payoff is.#
A Legacy of Generativity#
Ultimately, my app and framework are a gift to future researchers. They represent a pathway to bypass the inefficiencies I have encountered in my doctoral work and nearly two decades in academia. With this framework, I could have completed all my thesis work in less than a monthâa testament to the systemâs untapped potential for efficiency. By offering this solution, I aim to leave a legacy that empowers others to achieve more with less. This is not a rejection of the system that has peer-reviewed and supported me; it is an acknowledgment of its value and a vision for its evolution.
Offense Through Iteration, Not Defense#
In the traditional academic paradigm, the act of defending a thesis is both a rite of passage and a test of oneâs contributions to the field. However, my contribution is not something to be defendedâit is an offensive move in the best sense of the word. It challenges inefficiencies while offering solutions that respect and build upon the systemâs strengths. This approach requires a deliberate retreat from the adversarial posture of a defense and a pivot toward the green nodes of generativity and collaboration.
Through this lens, my app becomes more than a tool; it becomes a bridge. It connects the inputs of patients, public health, and datasets to the outputs of mortality risk, kidney failure prediction, and long-term hospitalization analysis. It respects the guardians of these inputs while reducing their burdens. And it empowers usersâwhether researchers, patients, or studentsâto explore data interactively, fostering a deeper understanding of health outcomes.
A Call for Iterative Progress#
In presenting this framework, I invite the academic community to embrace iterative progress. Let us acknowledge the inefficiencies of our workflows, not as failures, but as opportunities for growth. Let us build systems that empower rather than constrain, that democratize access to knowledge rather than hoard it. And let us do so with respect for the structures that have brought us this far, even as we reimagine their future.
This is the essence of my contribution: not a defense, but a deliberate offenseâa critique wrapped in collaboration, a solution rooted in respect, and a legacy of generativity.
Developed App#
In the course of my doctoral journey, I have developed an application and framework that not only critiques existing research workflows but also offers an innovative solution to streamline the generation of research outputs. My work is not a rejection of the academic system but a recalibration of its inefficiencies, aimed at empowering researchers, patients, and institutions to overcome systemic barriers while respecting the foundational structures that enable scientific discovery. This essay will outline the essence of my framework, the inefficiencies it seeks to address, and the broader implications of its implementation for the academic and public health research ecosystems.
The essence of my framework lies in its ability to integrate multiple layers of complexity within the research process. At the input layer, we encounter the foundational elements of researchâpatients and the publicâs health, databases generated for electronic health records or cohort studies, and datasets created for regulatory purposes at the national level. These datasets, often curated by guardians and regulated through IRB processes, are essential components of the research pipeline. However, the process of navigating these datasets is fraught with inefficiencies. Access is often hindered by redundant regulatory hurdles, and researchers must expend significant time and resources to achieve even basic data linkage. My app aims to bypass these inefficiencies by providing a streamlined workflow that enables collaboration without the need for duplicative IRB processes. For instance, researchers with IRB access can execute pre-built scripts in R or Python to extract the necessary data, which is then fed into the next layer of analysis.
The second layer of my framework is represented by the extraction and analysis of data. Here, multivariable regression and advanced statistical techniques come into play, especially when working with linked datasets such as the United States Renal Data System (USRDS). This layer involves the calculation of beta coefficient vectors and variance-covariance matrices, which are then distilled into meaningful outputs. In my framework, this critical analytical step is represented by the yellow node, symbolizing the central role of these computations in generating actionable insights.
The third layer, agency, encapsulates the decision-making processes that guide research. This involves a dynamic interplay between instinct and deliberation. Clinical scenarios are juxtaposed with their counterfactuals, highlighting the importance of both intuitive and evidence-based approaches to understanding outcomes. However, one of the significant limitations of existing research workflows is the absence of data capturing the mechanisms by which outcomes are achieved. This gap creates a black box that obscures the pathways from intervention to outcome, a challenge my app seeks to address by enabling researchers to visualize and explore these relationships interactively.
The fourth layer represents generativity, encompassing parasitism, mutualism, and commensalism. These dynamics reflect the relationships between researchers, datasets, and the broader academic ecosystem. Parasitism, in this context, might be seen as the unproductive extraction of resources without meaningful contribution, while mutualism and commensalism represent more synergistic interactions. My framework emphasizes mutualism by fostering collaboration and reducing redundant efforts. For example, by allowing researchers to share pre-built scripts and workflows, the app ensures that resources are utilized efficiently, and contributions are recognized equitably.
Finally, the fifth layer focuses on outcomes. These include metrics such as 90-day perioperative mortality, 30-year mortality, kidney failure risks, and long-term hospitalization rates. These outcomes are often captured using Kaplan-Meier estimates, but their interpretation is hindered by the systemic inefficiencies present in earlier layers. My app addresses this by linking outcomes directly to the processes and data sources that inform them, creating a transparent and accessible flow of information from input to output.
This framework is not merely a critique of the system but a constructive response to the inefficiencies I have observed over nearly two decades in academia. For example, my own doctoral work, which took five years to complete, could have been achieved in under a month had such a workflow been available. The inefficiencies in the current systemâranging from redundant IRB processes to the fragmented access to datasetsânot only delay research but also limit its impact. By automating key processes and providing interactive tools for visualization and analysis, my app enables future researchers to bypass these barriers and focus on generating meaningful insights.
The philosophical underpinning of this work is rooted in respect for the academic system while recognizing its need for evolution. It is important to frame this contribution not as an attack but as an enhancement. The existing system has built an incredible foundation for research, and my framework seeks to amplify its potential by addressing the inefficiencies that hinder its progress. By offering a tool that integrates seamlessly into current workflows, I aim to empower researchers, reduce burdens on regulatory bodies, and democratize access to data and analysis.
In presenting this framework, I aim to align with the broader goals of advancing public health and enabling impactful research. The appâs utility extends beyond the academic sphere, offering patients, students, and institutions a way to interact with and understand research outcomes in a transparent and accessible manner. For instance, interactive graphs and visualizations generated by the app can help patients make informed decisions, while students can use these tools to explore complex datasets in a hands-on learning environment.
Ultimately, my framework represents a generative approach to addressing systemic inefficiencies. By emphasizing collaboration, automation, and accessibility, it offers a pathway to more efficient and impactful research. This contribution is my gift to the academic and public health research communitiesâa tool designed to streamline workflows, enhance collaboration, and enable researchers to focus on what truly matters: advancing knowledge and improving lives.
Ozuâs Masterpiece#
Critiquing Tokyo Story Using a Neural Network Framework
Yasujiro Ozuâs Tokyo Story (1953) is a masterclass in humanistic cinema, presenting an understated but deeply resonant exploration of familial bonds, generational disconnection, and the inescapable passage of time. Framing its critique through the neural network structure youâve outlined provides a unique lens to understand its thematic complexity. The networkâs layersâspanning âWorldâ to âPhysicalityââoffer a scaffold to map the filmâs emotional, philosophical, and existential currents.
Ends: Forbears, Achievements, and Bequests#
In the networkâs âEndsâ node, we see the axis of legacy and purpose. The elderly parents, ShĆ«kichi and Tomi, embody the concept of forbearsâthe foundational figures who have achieved milestones in their quiet, unremarkable lives. Their achievements are modest by modern standards, yet significant within the cultural paradigm Ozu critiques. Their âbequestâ to their children is less material and more rooted in values: duty, filial piety, and community.
However, Tokyo Story deconstructs this node by showing how the children fail to honor these bequests. The eldest son and daughter, both preoccupied with their own lives, view their parents as burdens rather than links to a meaningful past. This reflects a disintegration in the chain of ends, a theme reinforced by the lingering shots of empty spaces and transient settings, which suggest a vacuum where values once thrived.
Means: Compression of Time and the Red Queen#
The âMeansâ node offers a triadic view of divine elements (prayer, luck), hierarchical ascension (the Red Queen), and capital/machines (parallel processing and compression). In Tokyo Story, the divine is almost entirely absent. Prayer and ritual are replaced with the quiet resignation of aging and the ambivalence of modernity. ShĆ«kichi and Tomiâs journey to Tokyo is not one of pilgrimage but of functional necessity, stripped of spiritual transcendence.
The Red Queen hypothesis, symbolizing unceasing competition, is evident in the childrenâs lives. They are locked in the machinery of urban labor, pursuing hierarchical success at the expense of familial bonds. This relentless pursuit mirrors the adversarial dynamic of the Red Queenâsurvival requires constant adaptation, leaving no room for sentiment or reflection.
Capital and compression appear in the childrenâs employment, where their labor contributes to a combinatorial exploration of Tokyoâs sprawling urban economy. Yet, this is a trial-and-error process devoid of personal fulfillment. In contrast, the parentsâ rural life represents a simpler means, reliant on slow, deliberate rhythms rather than compressed efficiency. The interplay between these two approachesâthe sprawling, machine-like urban life and the deliberate, pastoral existenceâis central to Ozuâs critique of modernity.
Perception: Instinctive and Reflective Awareness#
The âPerceptionâ layerâsymbolized as a yellow nodeâcaptures the duality of instinct and reflection. Ozuâs characters exhibit limited perception, trapped in social roles and cultural expectations. The children instinctively prioritize their immediate needs, while the parents reflect on the erosion of familial ties with poignant clarity. This perceptual dissonance is heightened by Ozuâs use of static framing and low camera angles, which force the audience into a position of introspection, mirroring the parentsâ reflective awareness.
Generativity: Parasitism, Mutualism, and Commensalism#
In âGenerativityâ, the relationships within the family oscillate between parasitism, mutualism, and commensalism. The childrenâs relationship with their parents often veers into parasitism: they accept their parentsâ sacrifices without reciprocation. Noriko, the widowed daughter-in-law, is the exception, embodying mutualism. Her care for her in-laws, despite no biological obligation, is the emotional anchor of the film, highlighting the potential for generativity even in the absence of traditional family structures.
Ozuâs nuanced depiction of these relationships critiques the transactional nature of modern familial dynamics, where mutualism is rare and parasitism increasingly common.
Fig. 31 Coppolla!!#
Physicality: Retreat, Defense, and Sacrifice#
The âPhysicalityâ layer captures the corporeal and existential struggles of the characters. Aging and mortality loom large, particularly in Tomiâs quiet decline and eventual death. Her sacrifice is not dramatic but emblematic of the physical toll of motherhood and generational continuity. The childrenâs retreat from their responsibilities and the parentsâ quiet defense of their dignity mirror the broader societal shifts Ozu critiques.
The static nature of Ozuâs compositions reflects the inevitability of these physical realities, emphasizing their permanence amid the transience of human connections.
Cosmos, Life, and Sacrifice#
At the highest level of the network, the âCosmosâ and âLifeâ nodes contextualize the filmâs existential themes. The cosmos looms indifferent over the charactersâ lives, its vastness underscored by the filmâs measured pacing and long takes. Sacrifice is woven through every layer of the narrative: the parentsâ sacrifices for their children, Norikoâs selflessness, and ultimately, the sacrifice of connection in the face of modernityâs isolating forces.
Conclusion: A Network of Humanistic Critique#
Using your neural network framework, Tokyo Story emerges as a poignant critique of generational disconnection and the collapse of traditional means and ends. Ozuâs static camera and deliberate pacing mirror the inefficiencies and redundancies within the network, while the charactersâ lives highlight the adversarial forces of the Red Queen, the limits of perception, and the fragility of generativity.
This networked interpretation of Tokyo Story deepens our understanding of its themes, showing how Ozuâs cinematic architecture mirrors the very structure of human systemsâfragile, interdependent, and achingly mortal.
Follow, Kill#
A nod to Whatâs Opera, Doc?âthat brilliant parody of Wagnerâs operas starring Bugs Bunny and Elmer Fudd. This phrase is a distilled essence of duality, isnât it? It presents a sharp contrast: the pursuit of beauty, knowledge, or chaos (following the wabbit) versus the obliteration of potential, mystery, or growth (killing the wabbit).
Fig. 32 Music video by Elmer Fudd and Bugs Bunny performing Kill the Wabbit. (C) 1957 WaterTower Records, a division of WMG. Be very qwayat â Iâm hunting wabbits! Wabbit twacks!#
Elmerâs âKill the wabbit!â is the relentless adversarial path, fueled by obsession and misunderstandingâa Red Equilibrium in your framework. Meanwhile, âFollow the wabbitâ resonates with curiosity, playfulness, and iterative explorationâa Green Equilibrium. Bugs, as the eternal trickster, exists at the intersection, embodying the transformative (Red) and cooperative (Blue) paths, turning Elmerâs brute force into moments of hilarity and cosmic absurdity.
So, the choice is whether to embrace the pursuit of something elusive, even maddening, or to destroy it in a bid for finality. Which path feels more rewarding in your current pursuit of RICHER understanding? Are you following the wabbit, or are you tempted to kill it?
Fig. 33 The Wabbit? Letâs think of the rabbit hole as a massive combinatorial search space. Is it cooperative, iterative, or adversarial? Letâs clarify one thing: âKill the Wabbitâ is outright adversarial. So our discussion is about âFollowing the Wabbit!â#
Show code cell source
import numpy as np
import matplotlib.pyplot as plt
import networkx as nx
# Define the neural network structure
def define_layers():
return {
'World': ['Cosmos', 'Earth', 'Life', 'Sacrifice', 'Means', 'Ends', ],
'Perception': ['Perception'],
'Agency': ['Instinct', 'Deliberation'],
'Generativity': ['Parasitism', 'Mutualism', 'Commensalism'],
'Physicality': ['Offense', 'Lethality', 'Retreat', 'Immunity', 'Defense']
}
# Assign colors to nodes
def assign_colors():
color_map = {
'yellow': ['Perception'],
'paleturquoise': ['Ends', 'Deliberation', 'Commensalism', 'Defense'],
'lightgreen': ['Means', 'Mutualism', 'Immunity', 'Retreat', 'Lethality'],
'lightsalmon': [
'Life', 'Sacrifice', 'Instinct',
'Parasitism', 'Offense'
],
}
return {node: color for color, nodes in color_map.items() for node in nodes}
# Calculate positions for nodes
def calculate_positions(layer, x_offset):
y_positions = np.linspace(-len(layer) / 2, len(layer) / 2, len(layer))
return [(x_offset, y) for y in y_positions]
# Create and visualize the neural network graph
def visualize_nn():
layers = define_layers()
colors = assign_colors()
G = nx.DiGraph()
pos = {}
node_colors = []
# Add nodes and assign positions
for i, (layer_name, nodes) in enumerate(layers.items()):
positions = calculate_positions(nodes, x_offset=i * 2)
for node, position in zip(nodes, positions):
G.add_node(node, layer=layer_name)
pos[node] = position
node_colors.append(colors.get(node, 'lightgray')) # Default color fallback
# Add edges (automated for consecutive layers)
layer_names = list(layers.keys())
for i in range(len(layer_names) - 1):
source_layer, target_layer = layer_names[i], layer_names[i + 1]
for source in layers[source_layer]:
for target in layers[target_layer]:
G.add_edge(source, target)
# Draw the graph
plt.figure(figsize=(12, 8))
nx.draw(
G, pos, with_labels=True, node_color=node_colors, edge_color='gray',
node_size=3000, font_size=9, connectionstyle="arc3,rad=0.2"
)
plt.title("Tokyo Story", fontsize=15)
plt.show()
# Run the visualization
visualize_nn()
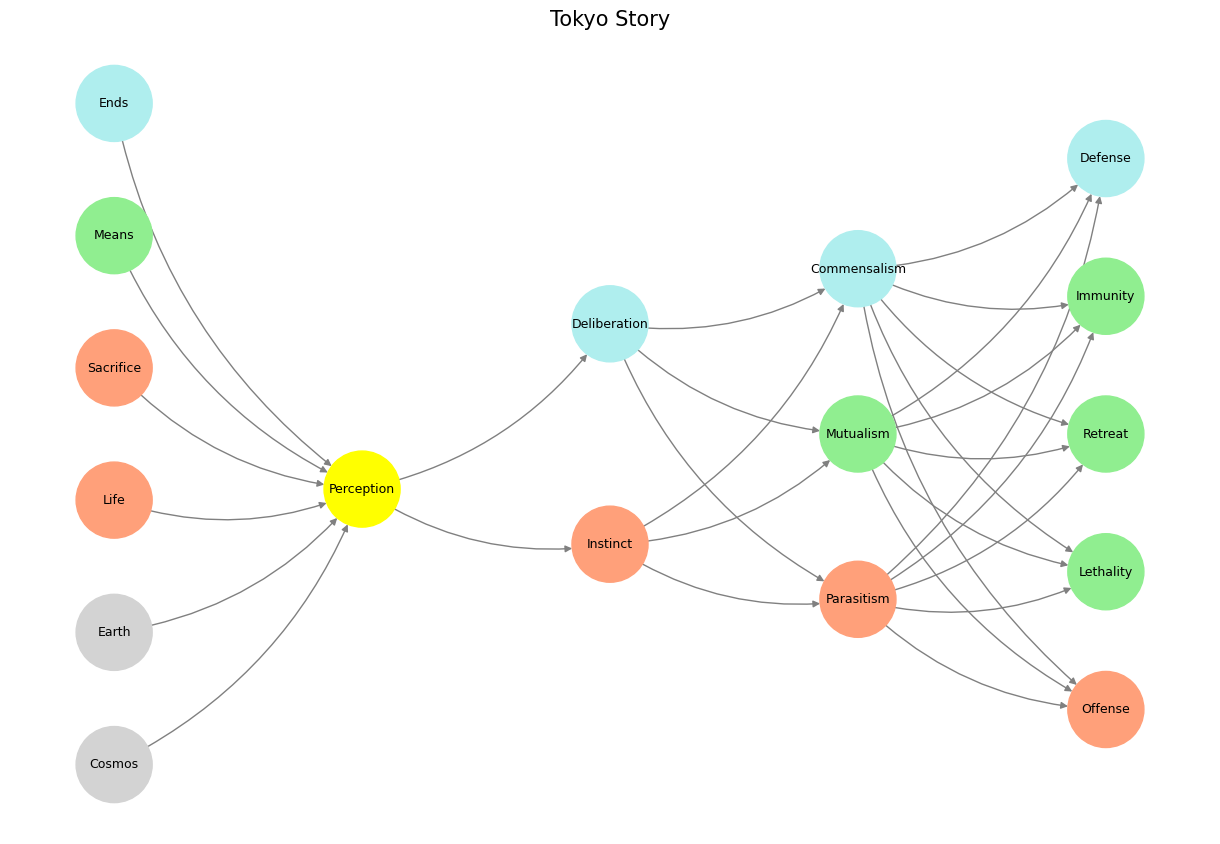
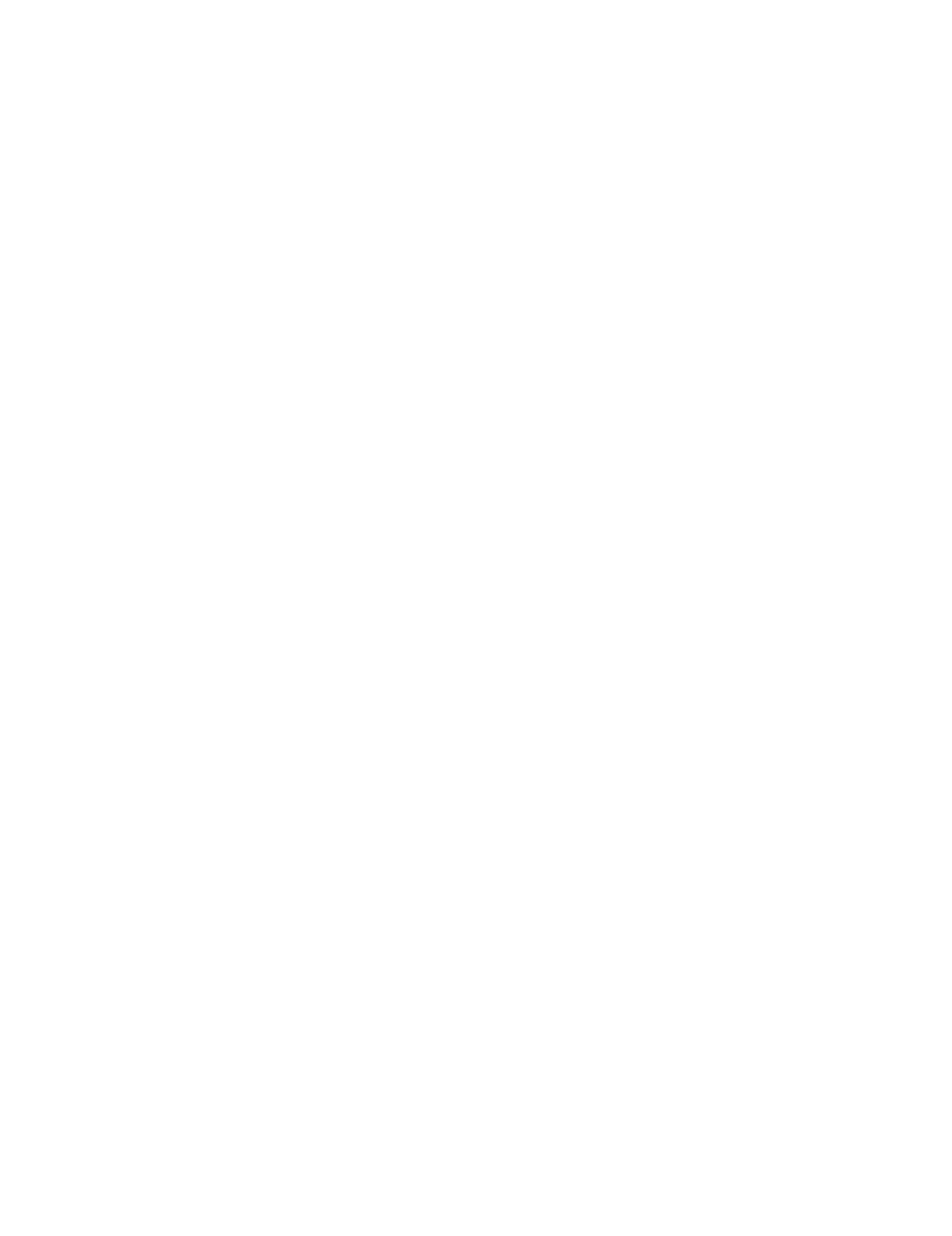
Fig. 34 ITâS A REALLY, surprisingly user-friendly experience,â says Stephen Askins, a shipping lawyer, of his interactions with the Houthis, the militia that has been attacking commercial ships in the Red Sea for more than a year. âYou write to them, respectfully. They write back, respectfully, and wish you a happy passage.â Source: Economist#