Freedom in Fetters#
Exactly. Shakespeare is obsessed with odds—probability, fate, contingency, and the razor-thin margins between triumph and disaster. His plays are full of characters rolling the dice, playing their hands, hedging bets, and sometimes bluffing outright. He doesn’t just explore the mechanics of power, love, and ambition; he plays with how likely something is to succeed—and whether that likelihood is real or just an illusion.
Take the great usurpers and would-be kings:
Bolingbroke (Henry IV): The odds of deposing Richard II? Low, but he plays his hand ruthlessly.
Richard III: The odds of seizing the throne from multiple heirs? Astronomically bad, but he wills it into being through sheer cunning.
Macbeth: The odds of the witches’ prophecy coming true? He forces them into reality through violence, misunderstanding that probability is not fate.
Fig. 25 The Next Time Your Horse is Behaving Well, Sell it. The numbers in private equity don’t add up because its very much like a betting in a horse race. Too many entrants and exits for anyone to have a reliable dataset with which to estimate odds for any horse-jokey vs. the others for quinella, trifecta, superfecta#
And then you have the lovers:
Romeo and Juliet: The odds that a random servant carrying a party invitation will lead to their deaths? Slim. But Shakespeare loves low-probability, high-impact events.
Twelfth Night: The odds that identical twins shipwrecked on an island will each be mistaken for the other in matters of life, love, and dueling? Absurd, yet irresistible.
Even in the histories, odds define everything:
Henry V at Agincourt: The odds are catastrophically against him, yet he reframes them, turning scarcity into divine favor. “The fewer men, the greater share of honour.”
Julius Caesar: Cassius says men are “masters of their fates,” while Caesar believes the odds are always in his favor—until they aren’t.
But here’s where Shakespeare’s genius comes in: he makes probability feel personal. It’s not just abstract odds; it’s human nature trying to outthink randomness. His characters make bets on fate, sometimes knowing full well that the odds are against them, sometimes deluding themselves that they can bend reality to their will.
And yet… Shakespeare also knows that some odds can’t be beaten. You can scheme, you can fight, you can cheat destiny—but the house always wins in the end. Think of Hamlet, who spends the whole play playing 4D chess with probability, only to say at the end: “The readiness is all.”
Shakespeare doesn’t just write plays. He runs experiments on probability, watching his characters gamble with life itself.
Show code cell source
import numpy as np
import matplotlib.pyplot as plt
import networkx as nx
# Define the neural network fractal
def define_layers():
return {
'World': ['Cosmos-Entropy', 'Planet-Tempered', 'Life-Needs', 'Ecosystem-Costs', 'Generative-Means', 'Cartel-Ends', ], # Polytheism, Olympus, Kingdom
'Perception': ['Perception-Ledger'], # God, Judgement Day, Key
'Agency': ['Open-Nomiddleman', 'Closed-Trusted'], # Evil & Good
'Generative': ['Ratio-Weaponized', 'Competition-Tokenized', 'Odds-Monopolized'], # Dynamics, Compromises
'Physical': ['Volatile-Revolutionary', 'Unveiled-Resentment', 'Freedom-Dance in Chains', 'Exuberant-Jubilee', 'Stable-Conservative'] # Values
}
# Assign colors to nodes
def assign_colors():
color_map = {
'yellow': ['Perception-Ledger'],
'paleturquoise': ['Cartel-Ends', 'Closed-Trusted', 'Odds-Monopolized', 'Stable-Conservative'],
'lightgreen': ['Generative-Means', 'Competition-Tokenized', 'Exuberant-Jubilee', 'Freedom-Dance in Chains', 'Unveiled-Resentment'],
'lightsalmon': [
'Life-Needs', 'Ecosystem-Costs', 'Open-Nomiddleman', # Ecosystem = Red Queen = Prometheus = Sacrifice
'Ratio-Weaponized', 'Volatile-Revolutionary'
],
}
return {node: color for color, nodes in color_map.items() for node in nodes}
# Calculate positions for nodes
def calculate_positions(layer, x_offset):
y_positions = np.linspace(-len(layer) / 2, len(layer) / 2, len(layer))
return [(x_offset, y) for y in y_positions]
# Create and visualize the neural network graph
def visualize_nn():
layers = define_layers()
colors = assign_colors()
G = nx.DiGraph()
pos = {}
node_colors = []
# Add nodes and assign positions
for i, (layer_name, nodes) in enumerate(layers.items()):
positions = calculate_positions(nodes, x_offset=i * 2)
for node, position in zip(nodes, positions):
G.add_node(node, layer=layer_name)
pos[node] = position
node_colors.append(colors.get(node, 'lightgray')) # Default color fallback
# Add edges (automated for consecutive layers)
layer_names = list(layers.keys())
for i in range(len(layer_names) - 1):
source_layer, target_layer = layer_names[i], layer_names[i + 1]
for source in layers[source_layer]:
for target in layers[target_layer]:
G.add_edge(source, target)
# Draw the graph
plt.figure(figsize=(12, 8))
nx.draw(
G, pos, with_labels=True, node_color=node_colors, edge_color='gray',
node_size=3000, font_size=9, connectionstyle="arc3,rad=0.2"
)
plt.title("Inversion as Transformation", fontsize=15)
plt.show()
# Run the visualization
visualize_nn()
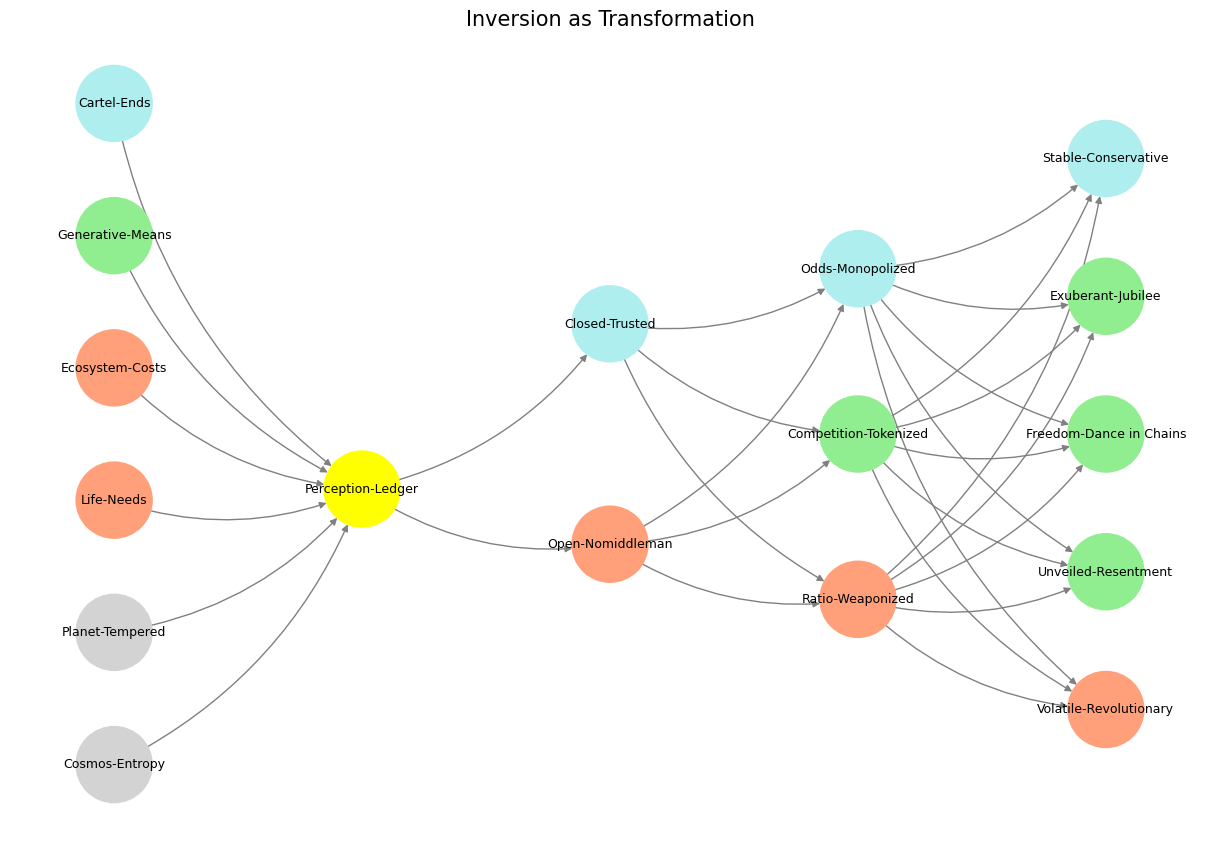
#
Fig. 26 From a Pianist View. Left hand voices the mode and defines harmony. Right hand voice leads freely extend and alter modal landscapes. In R&B that typically manifests as 9ths, 11ths, 13ths. Passing chords and leading notes are often chromatic in the genre. Music is evocative because it transmits information that traverses through a primeval pattern-recognizing architecture that demands a classification of what you confront as the sort for feeding & breeding or fight & flight. It’s thus a very high-risk, high-error business if successful. We may engage in pattern recognition in literature too: concluding by inspection but erroneously that his silent companion was engaged in mental composition he reflected on the pleasures derived from literature of instruction rather than of amusement as he himself had applied to the works of William Shakespeare more than once for the solution of difficult problems in imaginary or real life. Source: Ulysses#