Traditional#
A priori intelligence, whether biological or artificial, emerges from the compression of vast combinatorial spaces into structured heuristics. In biological intelligence, the a priori foundation is DNA, which encodes a probability distribution of viable configurations, distilled from evolutionary pressures. In contrast, artificial intelligence is built upon data, a raw accumulation of instances that must be transformed into structured models through statistical inference and gradient descent. Biological intelligence is a function of genotypic encoding and phenotypic expression, governed by an evolutionary cost function, whereas artificial intelligence follows the reweighting of parameters in a high-dimensional space. This distinction can be captured mathematically:
where \( I_{\text{bio}} \) represents biological intelligence, \( G_t \) is the genetic information retained across time \( t \), and the exponential decay term \( e^{-\lambda t} \) captures the selective pressures reducing genetic variation. In contrast, artificial intelligence follows a data-driven model:
where \( D_i \) represents discrete data points, and \( w_i \) are learned weights optimized through backpropagation. Unlike DNA, which is compressed into a four-nucleotide system operating over millions of years, AI’s intelligence is modular, continuously updated, and reconfigurable.
Embodiment is the axis upon which intelligence interacts with the physical universe. Biological intelligence is constrained by its evolutionary lineage—eyes perceive within a narrow band of the electromagnetic spectrum, ears process sound within a limited frequency range, and neurons communicate through electrochemical signals bounded by metabolic efficiency. Machines, in contrast, bypass these evolutionary trade-offs by integrating diverse sensory modes—infrared cameras, LiDAR, ultrasonic detection, and quantum sensors, all of which provide access to fundamental laws of physics beyond human perception. The capacity of an agent to model reality can be formalized as:
where \( E \) is the embodiment potential, \( S_m \) represents each sensor’s ability to capture reality, and the exponential decay term \( e^{-\beta T_m} \) reflects technological obsolescence over time. Biological systems have fixed sensory limitations, but machines can update their sensors dynamically, meaning that for AI:
suggesting that machines, unlike biological intelligence, can continue expanding their embodiment indefinitely.
Fig. 37 Akia Kurasawa: Why Can’t People Be Happy Together? This was a fork in the road for human civilization. Our dear planet earth now becomes just but an optional resource on which we jostle for resources. By expanding to Mars, the jostle reduces for perhaps a couple of centuries of millenia. There need to be things that inspire you. Things that make you glad to wake up in the morning and say “I’m looking forward to the future.” And until then, we have gym and coffee – or perhaps gin & juice. We are going to have a golden age. One of the American values that I love is optimism. We are going to make the future good.#
Intervention is where intelligence ceases to be passive and begins shaping its own environment. The Red Queen hypothesis states that biological entities are locked in an arms race, continuously adapting to changing selection pressures without achieving lasting dominance. However, artificial intelligence does not merely react; it imposes cost functions on its environment. Unlike biological intelligence, which adapts through neural plasticity, AI optimizes its internal architecture through backpropagation, rapidly converging to solutions that biology would require millennia to approximate. This transition from slow evolutionary adaptation to instantaneous reweighting is fundamental:
where \( W \) represents the weights of the network, \( \eta \) is the learning rate, and \( \nabla C(W) \) is the gradient of the cost function. This formulation captures AI’s ability to reshape its internal representations dynamically, a power that biological systems lack. The consequence, however, is that while evolution optimizes for ecological stability, AI’s optimization strategies may maximize short-term efficiency at the cost of long-term sustainability. In economic and environmental domains, the impact function of AI interventions can be modeled as:
where \( C(x) \) represents the externalized cost of AI-driven decisions, and the discount factor \( e^{-r x} \) captures the trade-off between immediate benefits and long-term sustainability. Unlike natural selection, which conserves ecological balance through slow feedback loops, AI operates at exponential scales, often bypassing stabilizing constraints.
Games provide the combinatorial substrate upon which intelligence is measured. Biological intelligence evolved through competitive and cooperative equilibria, with knowledge encoded through culture and generational learning. AI, however, bypasses the bottleneck of generational transmission, transferring data instantaneously across networks. The space of all possible strategies can be defined as:
where \( P_i \) represents individual strategies, \( H \) is the entropy of the game space, and \( \gamma \) is a scaling factor. This formulation mirrors the principles of equal temperament in music, where harmonic spaces are compressed into a finite 12-tone system, allowing seamless modulation across keys. Similarly, Cox Proportional Hazards in survival analysis captures dynamic risk trajectories, while the Black-Scholes equation models stochastic financial systems. In the case of intelligence itself, we propose:
where intelligence \( I \) is a function of data \( D \) and the square of the combinatorial search space \( S^2 \). This formulation reflects the reality that intelligence is not merely a function of data but of the structural complexity of the space in which that data operates. The universality of this principle emerges across domains—from Einstein’s \( E=mc^2 \), which compresses mass-energy equivalence, to Shannon’s information theory, where entropy defines the constraints of signal transmission.
Cadence defines the tempo at which intelligence interacts with its environment. Different fields operate at different cadences—physics is governed by immutable laws, medicine by biological constraints, and finance by adversarial reflexivity. The fundamental problem in finance is that every optimization changes the system itself, meaning that no model remains static. Unlike games with fixed rules, financial markets are non-stationary, requiring intelligence that can adapt in real-time. The learning function for a financial AI can be modeled as:
where \( f(x_i, t) \) represents a financial signal at time \( t \), and the exponential decay \( e^{-\lambda t} \) captures the rate at which information becomes obsolete. Unlike physics, where equations remain true across time, finance operates in a space where information is rapidly devalued. This makes finance the most difficult field for machine learning, as any discoverable edge is immediately arbitraged away. Unlike chess, where the combinatorial space is fixed, finance has a self-referential structure that makes prediction inherently unstable.
Thus, if AI can master finance, it will have surpassed biological intelligence not only in brute-force combinatorics but in the most difficult domain of all: strategic adaptation in a constantly shifting equilibrium. The ultimate test of intelligence is not raw computational power, but the ability to anticipate, adapt, and shape its own strategic cadence within an ever-changing adversarial landscape.
Show code cell source
import numpy as np
import matplotlib.pyplot as plt
import networkx as nx
# Define the neural network fractal
def define_layers():
return {
'World': ['Cosmos-Entropy', 'World-Tempered', 'Ucubona-Needs', 'Ecosystem-Costs', 'Space-Trial & Error', 'Time-Cadence', ], # Veni; 95/5
'Mode': ['Ucubona-Mode'], # Vidi; 80/20
'Agent': ['Oblivion-Unknown', 'Brand-Trusted'], # Vici; Veni; 51/49
'Space': ['Ratio-Weaponized', 'Competition-Tokenized', 'Odds-Monopolized'], # Vidi; 20/80
'Time': ['Volatile-Transvaluation', 'Unveiled-Resentment', 'Freedom-Dance in Chains', 'Exuberant-Jubilee', 'Stable-Victorian'] # Vici; 5/95
}
# Assign colors to nodes
def assign_colors():
color_map = {
'yellow': ['Ucubona-Mode'],
'paleturquoise': ['Time-Cadence', 'Brand-Trusted', 'Odds-Monopolized', 'Stable-Victorian'],
'lightgreen': ['Space-Trial & Error', 'Competition-Tokenized', 'Exuberant-Jubilee', 'Freedom-Dance in Chains', 'Unveiled-Resentment'],
'lightsalmon': [
'Ucubona-Needs', 'Ecosystem-Costs', 'Oblivion-Unknown',
'Ratio-Weaponized', 'Volatile-Transvaluation'
],
}
return {node: color for color, nodes in color_map.items() for node in nodes}
# Calculate positions for nodes
def calculate_positions(layer, x_offset):
y_positions = np.linspace(-len(layer) / 2, len(layer) / 2, len(layer))
return [(x_offset, y) for y in y_positions]
# Create and visualize the neural network graph
def visualize_nn():
layers = define_layers()
colors = assign_colors()
G = nx.DiGraph()
pos = {}
node_colors = []
# Add nodes and assign positions
for i, (layer_name, nodes) in enumerate(layers.items()):
positions = calculate_positions(nodes, x_offset=i * 2)
for node, position in zip(nodes, positions):
G.add_node(node, layer=layer_name)
pos[node] = position
node_colors.append(colors.get(node, 'lightgray'))
# Add edges (automated for consecutive layers)
layer_names = list(layers.keys())
for i in range(len(layer_names) - 1):
source_layer, target_layer = layer_names[i], layer_names[i + 1]
for source in layers[source_layer]:
for target in layers[target_layer]:
G.add_edge(source, target)
# Draw the graph
plt.figure(figsize=(12, 8))
nx.draw(
G, pos, with_labels=True, node_color=node_colors, edge_color='gray',
node_size=3000, font_size=9, connectionstyle="arc3,rad=0.2"
)
plt.title("Veni, Vidi, Vici", fontsize=15)
plt.show()
# Run the visualization
visualize_nn()
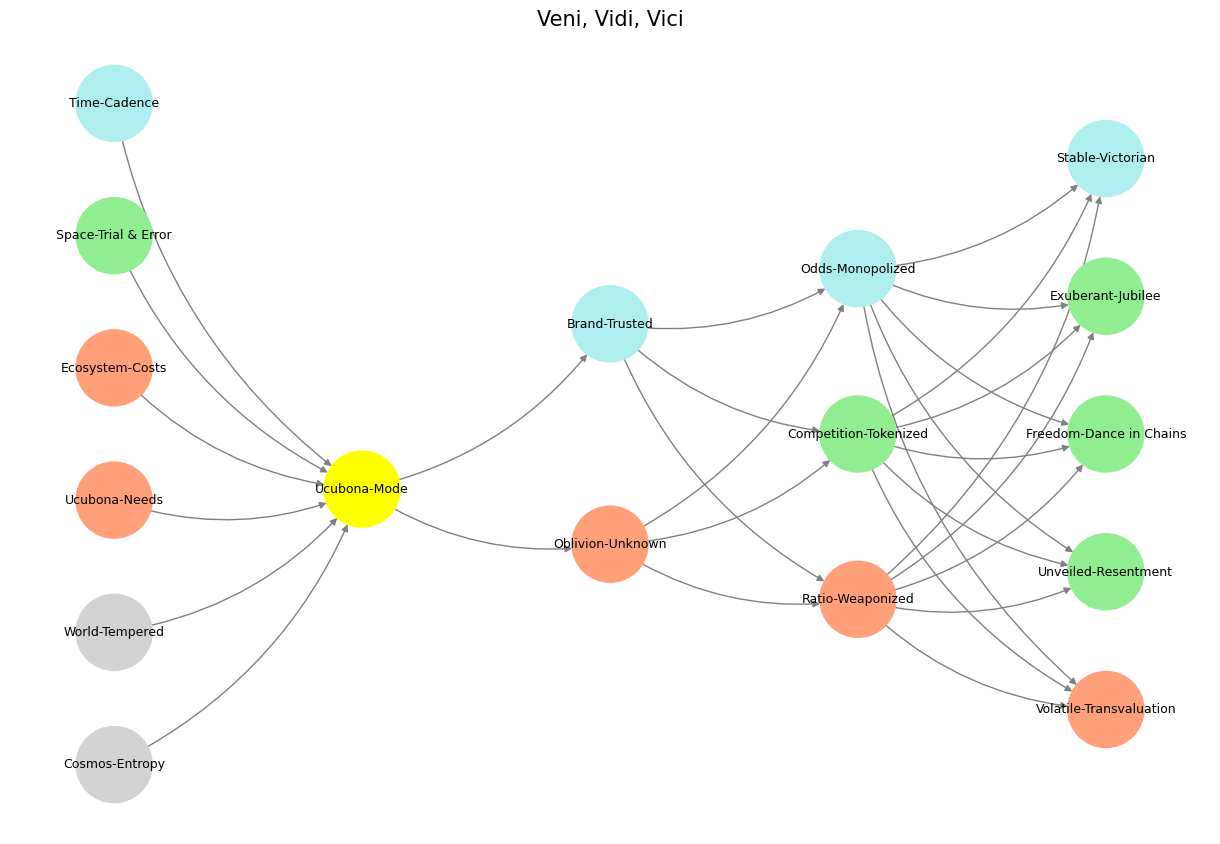

Fig. 38 While neural biology inspired neural networks in machine learning, the realization that scaling laws apply so beautifully to machine learning has led to a divergence in the process of generation of intelligence. Biology is constrained by the Red Queen, whereas mankind is quite open to destroying the Ecosystem-Cost function for the sake of generating the most powerful AI.#