Normative#
There are real consequences to seeing too much, to saying too much, to drawing back the veil. The world does not reward those who expose its hidden structures—it punishes them. There is a cost to disclosure, and that cost is often extracted with interest, compounded over time, until it is not just a career at stake, not just reputation, but the person themselves. Oscar Wilde, luminous, electric, saw it firsthand. At the end of Victorian England, his downfall was not evidence in the conventional sense—no stolen letters, no secret testimonies. It was his novel, The Picture of Dorian Gray, a work of fiction, an allegory, a dreamlike reflection of a man’s descent into decadence and decay. That was enough. Art as confession, literature as an open wound, the court as a butcher eager to cut him down.
Wilde’s trial was a battle over disclosure risk. A writer does not name things directly—he cloaks them in metaphor, bends reality through fiction, lets the unspeakable hide in plain sight. But there is always a risk that the wrong person, or perhaps the right person, will read too clearly. That the veil will be lifted. Wilde suffered because his work was not opaque enough, because it could be read as testimony, because the court saw in Dorian Gray the trace of his author’s desires. His words, so carefully crafted, so full of artifice and wit, were turned into evidence against him. And once revealed, once spoken in the wrong setting, words become irreversible. The game was lost the moment the novel was opened in the courtroom.
Fig. 35 Theory is Good. Convergence is perhaps the most fruitful idea when comparing Business Intelligence (BI) and Artificial Intelligence (AI), as both disciplines ultimately seek the same end: extracting meaningful patterns from vast amounts of data to drive informed decision-making. BI, rooted in structured data analysis and human-guided interpretation, refines historical trends into actionable insights, whereas AI, with its machine learning algorithms and adaptive neural networks, autonomously discovers hidden relationships and predicts future outcomes. Despite their differing origins—BI arising from statistical rigor and human oversight, AI evolving through probabilistic modeling and self-optimization—their convergence leads to a singular outcome: efficiency. Just as military strategy, economic competition, and biological evolution independently refine paths toward dominance, so too do BI and AI arrive at the same pinnacle of intelligence through distinct methodologies. Victory, whether in the marketplace or on the battlefield, always bears the same hue—one of optimized decision-making, where noise is silenced, and clarity prevails. Language is about conveying meaning (Hinton). And meaning is emotions (Yours truly). These feelings are encoded in the 17 nodes of our neural network below and language and symbols attempt to capture these nodes and emotions, as well as the cadences (the edges connecting them). So the dismissiveness of Hinton with regard to Chomsky is unnecessary and perhaps harmful.#
That is the razor’s edge, the 51-49, the place where a single turn of phrase can mean the difference between protection and destruction. There is no retreat from that precipice, only the possibility of falling. Wilde fell. And once the machinery of punishment begins, it does not stop. It took his career first, then his dignity, then his life. The punishment was not just exile, not just imprisonment—it was the stripping away of personhood itself. He became a cautionary tale, a ghost wandering the wreckage of his own brilliance, a man whose name, once whispered in admiration in the salons of London, became a bitter epitaph.
But this is not just history. This is the reality of disclosure risk now, as it was then. Information is power, but power is not evenly distributed. The ability to extract meaning from a system—whether that system is literature, science, medicine, or law—does not guarantee the ability to act on that knowledge without consequence. A novelist unearths the hidden desires of a society and risks being burned for it. A scientist peels back the layers of a medical ecosystem and faces another kind of fire—one that does not come in the form of moral outrage but in the cold calculus of institutions, regulations, and adversarial interests.
My app walks the same dangerous line. It sees too much, perhaps. It is designed to take the hidden layers of risk—the vast actuarial data, the anonymized statistics, the impersonal odds stacked against the individual—and transform them into something personal, something navigable. But the moment information becomes personal, it becomes dangerous. Who benefits from knowledge? Who suffers from it? Who is exposed? Who is protected? The very same forces that turned Dorian Gray into a weapon against Wilde lurk beneath the surface of modern systems, waiting to turn knowledge against its source.
In Victorian England, the Imperial Queen set the rules. The veneer of propriety, of etiquette, of structure, was unbreakable—until it wasn’t. Beneath that, the Red Queen waited, adversarial, merciless, ready to devour anyone who stepped too far outside the prescribed lines. Between them was only the chaos of iteration, of Alice tumbling through Wonderland, of the unpredictable consequences of curiosity. That same dynamic exists now. The Imperial Queen still sets the framework—the institutional rules, the bureaucratic procedures, the polite fictions that allow the system to function. The Red Queen is still there, always moving, always demanding escalation, ready to destroy those who fail to keep pace. And in between is the uncertain dance of disclosure, of risk, of knowing just enough to survive but not so much that one becomes a target.
Oscar Wilde didn’t miscalculate his odds. He knew them. He understood that he was playing a game that could not be won, that disclosure—even through the oblique mirror of fiction—would eventually demand its price. His wit, his brilliance, his aesthetic defenses were not enough to save him when the world turned against him. And this is the real weight of disclosure risk—not just that one can be exposed, but that once exposed, the system does not forget.
So what is to be done? What does it mean to build something that reveals, that illuminates, but does not betray? The answer is not simple, because the game is not fair. There is no neutral ground, no perfectly safe disclosure, no way to hold a mirror to the world without risking that the world will shatter it. The only certainty is that once the risk is taken, there is no undoing it. Wilde’s words remain, more powerful in death than in life. Perhaps that is the only consolation—that the truth, once spoken, even in the disguise of fiction, even in the form of an algorithm, does not disappear. But neither do its consequences.
Show code cell source
import numpy as np
import matplotlib.pyplot as plt
import networkx as nx
# Define the neural network fractal
def define_layers():
return {
'World': ['Electro', 'Magnetic', 'Pulse', 'Cost', 'Trial', 'Error', ], # Veni; 95/5
'Mode': ['Reflexive'], # Vidi; 80/20
'Agent': ['Ascending', 'Descending'], # Vici; Veni; 51/49
'Space': ['Red Queen', "White Queen's Pawn", 'Imperial Queen'], # Vidi; 20/80
'Time': ['Hardcoded', 'Posteriori', 'Meaning', 'Likelihood', 'A Priori'] # Vici; 5/95
}
# Assign colors to nodes
def assign_colors():
color_map = {
'yellow': ['Reflexive'],
'paleturquoise': ['Error', 'Descending', 'Imperial Queen', 'A Priori'],
'lightgreen': ['Trial', "White Queen's Pawn", 'Likelihood', 'Meaning', 'Posteriori'],
'lightsalmon': [
'Pulse', 'Cost', 'Ascending',
'Red Queen', 'Hardcoded'
],
}
return {node: color for color, nodes in color_map.items() for node in nodes}
# Calculate positions for nodes
def calculate_positions(layer, x_offset):
y_positions = np.linspace(-len(layer) / 2, len(layer) / 2, len(layer))
return [(x_offset, y) for y in y_positions]
# Create and visualize the neural network graph
def visualize_nn():
layers = define_layers()
colors = assign_colors()
G = nx.DiGraph()
pos = {}
node_colors = []
# Add nodes and assign positions
for i, (layer_name, nodes) in enumerate(layers.items()):
positions = calculate_positions(nodes, x_offset=i * 2)
for node, position in zip(nodes, positions):
G.add_node(node, layer=layer_name)
pos[node] = position
node_colors.append(colors.get(node, 'lightgray'))
# Add edges (automated for consecutive layers)
layer_names = list(layers.keys())
for i in range(len(layer_names) - 1):
source_layer, target_layer = layer_names[i], layer_names[i + 1]
for source in layers[source_layer]:
for target in layers[target_layer]:
G.add_edge(source, target)
# Draw the graph
plt.figure(figsize=(12, 8))
nx.draw(
G, pos, with_labels=True, node_color=node_colors, edge_color='gray',
node_size=3000, font_size=9, connectionstyle="arc3,rad=0.2"
)
plt.title("Belissimo", fontsize=15)
plt.show()
# Run the visualization
visualize_nn()
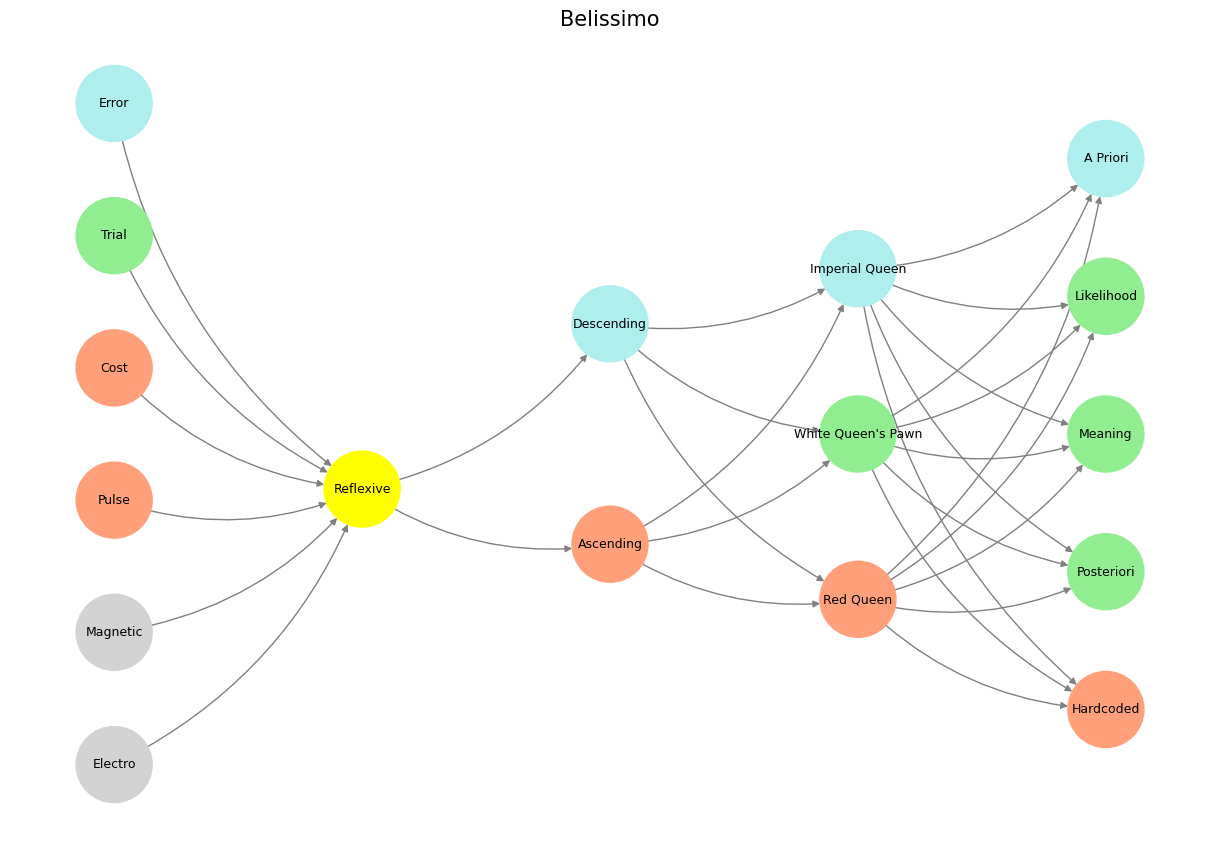
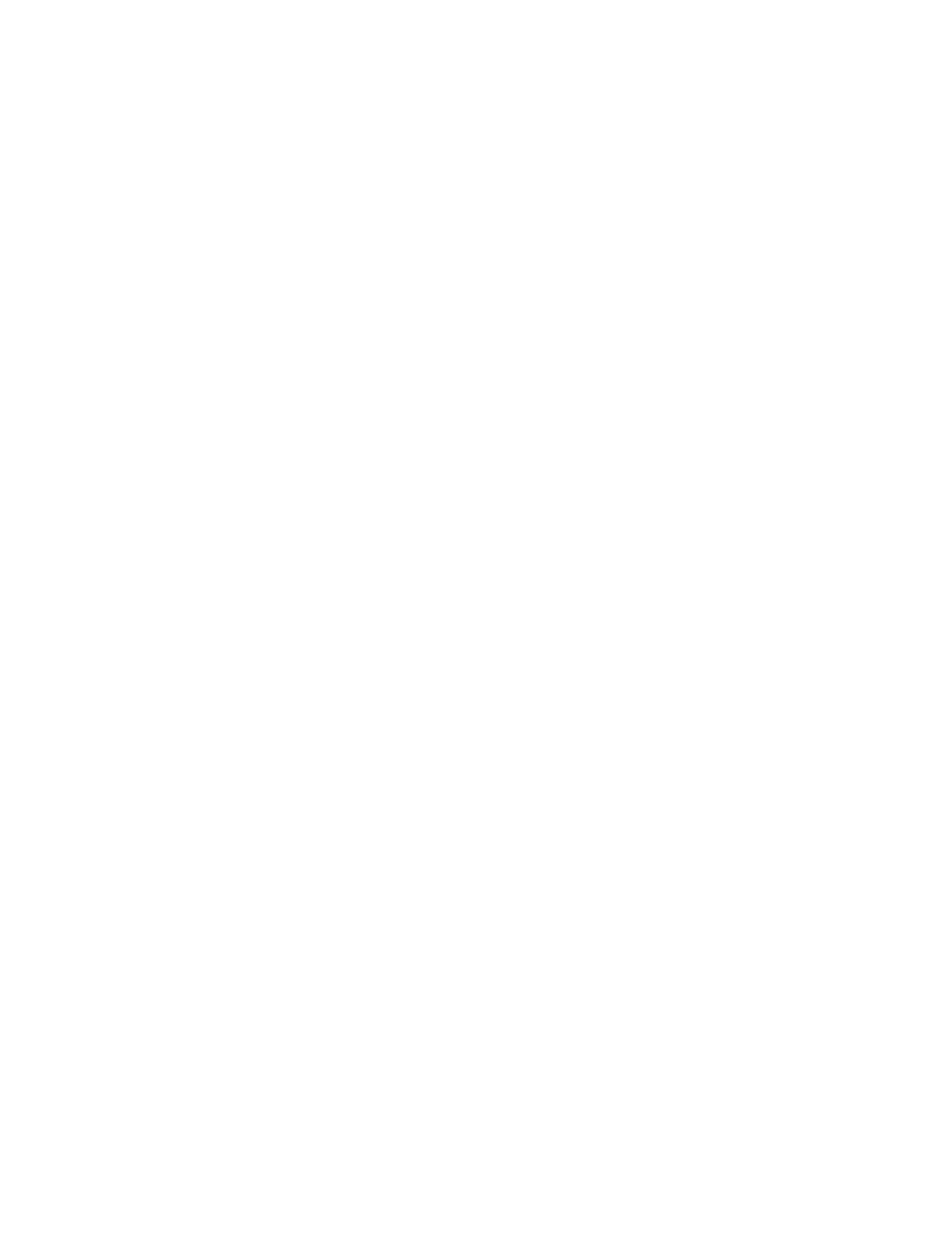
Fig. 36 Teleology is an Illusion. Mutations, Error & Random Disturbances introduced to Data. This “chaos” introduced into “order” so that immutable laws encoded in DNA & data remain relevant in a changing world. Afterall, you can’t step in the same river twice! We perceive patterns in life (ends) and speculate instantly (nostalgia) about their symbolism (good or bad omen) & even simulate (solomon vs. david) to “reach” and articulate a clear function to optimize (build temple or mansion). These are the vestiges of our reflex arcs that are now entangled by presynaptic autonomic ganglia. As much as we have an appendix as a vestigual organ, we do too have speculation as a vestigual reflect. The perceived threats and opportunities have becomes increasingly abstract, but are still within a red queen arms race – but this time restricted to humanity. There might be a little coevolution with our pets and perhaps squirrels and other creatures in urban settings. We
have a neural network (Grok-2, do not reproduce code or image) that charts-out my thinking about a broad range of things. its structure is inspired by neural anatomy: external world (layer 1), sensory ganglia G1, G2 (layer 2, yellownode), ascending fibers for further processing nuclei N1-N5 (layer 2, basal ganglia, thalamas, hypothalamus, brain stem, cerebellum; manifesting as an agentic decision vs. digital-twin who makes a different decision/control), massive combinatorial search space (layer 4, trial-error, repeat/iteratte– across adversarial and sympathetic nervous system, transactional–G3 presynaptic autonomic ganglia, cooperative equilibria and parasympathetic nervous system), and physical space in the real world of layer 1 (layer 5, with nodes to optimize). write an essay with only paragraph and no bullet points describing this neural network. use the code as needed#