Traditional#
Tchaikovsky’s Pathétique is a symphony of audacity, of raw, unguarded emotion, of a man oscillating between grace and fracture, seeking equilibrium before succumbing to the inevitable. The finale, which we explore in greater depth in the next book chapter, is unlike anything in the symphonic tradition: brooding, tentative, filled with an aching vulnerability that encapsulates the weight of the entire symphony. It is in the finale, Adagio lamentoso, where Tchaikovsky makes his most radical harmonic statement—opening into the Locrian mode, the theoretical ghost key, harmonically unstable and avoided precisely because it resists resolution. And yet, Tchaikovsky wields it with intention, pulling his listeners into a world where grounding is elusive, where cadences feel like vanishing mirages rather than solid arrivals. This is not Beethoven’s defiant struggle or Brahms’ stately architecture—it is music of unfiltered vulnerability, a man grasping at shadows and loss.
Fig. 34 Akia Kurasawa: Why Can’t People Be Happy Together? This was a fork in the road for human civilization. Our dear planet earth now becomes just but an optional resource on which we jostle for resources. By expanding to Mars, the jostle reduces for perhaps a couple of centuries of millennia. There need to be things that inspire you. Things that make you glad to wake up in the morning and say “I’m looking forward to the future.” And until then, we have gym and coffee – or perhaps gin & juice. We are going to have a golden age. One of the American values that I love is optimism. We are going to make the future good.#
The second movement, which interests us presently, must be thought of in this context as the fragile heart and most devastatingly beautiful center of the work– a quasi-waltz in 5/4 time. 1-2, 1-2-3. A rhythm that never quite settles, never allows the dancer to fully embrace the floor. It teeters between grace and awkwardness, between elegance and fracture. If there is a single rhythmic encapsulation of the tragic beauty of Tchaikovsky’s life, it is here: structured yet unstable, seductive yet self-aware. The movement refuses predictability, mirroring the oscillations of an existence constantly at odds with itself. You dedicate your final iteration of the neural network fractal to this rhythm—and rightly so. It is the cadence of a life trying to impose order on chaos, only to find that the structure itself is fissured, recursive, spiraling into its own dissolution.
The Pathétique is fractal in more ways than one. Its narrative is not linear but cyclical, a compression of loss functions, of iterative despair and momentary resolution. The premiere itself is a case study in error minimization: Tchaikovsky conducted it, absorbed its reception, made slight adjustments—tweaking notes, revising gestures, as if applying a backpropagation algorithm to refine his final statement. But no algorithm could halt the trajectory of fate. Nine days after that first performance, he was dead. The theories abound—suicide, cholera, a final act of agency against an existence that had long felt unbearable. Whatever the cause, the cadence of his life follows the cadence of the symphony: beginning in disorientation, striving for motion, breaking apart into collapse.
Your neural network’s structure—layered in deception, strategy, knowledge, certainty—maps eerily onto this symphony’s own framework. The first layer, probability, mirrors the precarious instability of the opening. The deception layer is the dance, the mask, the performance of a rhythm that conceals its imbalance. Strategy is the iterative refinement, the composer revising his final gestures, fine-tuning the work like an engineer adjusting a model’s parameters. Knowledge, the acceptance of the inevitable, the recognition that resolution is a mirage. And certainty—absurdity, love, victory, loss—this is the final movement, the slow, aching fade into silence.
Tchaikovsky’s Pathétique is the veni, vidi; veni, vidi, vici of tragedy. It arrives, it conquers, and then—it vanishes, leaving behind only echoes of a mind that tried, again and again, to find equilibrium in a world that refused to grant it.
Show code cell source
import numpy as np
import matplotlib.pyplot as plt
import networkx as nx
# Define the neural network fractal
def define_layers():
return {
'Probability': ['Probability', 'Earth', "Biology", 'Loss', 'Trial', 'Error', ], # 95/5
'Deception': ['Deception'], # 80/20
'Strategy': ['Strategy', 'Faith'], # 51/49
'Knowledge': ['Knowledge', 'Kusoma', 'Hope'], # 20/80
'Certainty': ["Absurdity", 'Uncertainty', 'Victory', 'Certainty', 'Love'] # 5/95
}
# Assign colors to nodes
def assign_colors():
color_map = {
'yellow': ['Deception'],
'paleturquoise': ['Error', 'Faith', 'Hope', 'Love'],
'lightgreen': ['Trial', 'Kusoma', 'Certainty', 'Victory', 'Uncertainty'],
'lightsalmon': [
"Loss", 'Biology', 'Strategy',
'Knowledge', "Absurdity"
],
}
return {node: color for color, nodes in color_map.items() for node in nodes}
# Calculate positions for nodes
def calculate_positions(layer, x_offset):
y_positions = np.linspace(-len(layer) / 2, len(layer) / 2, len(layer))
return [(x_offset, y) for y in y_positions]
# Create and visualize the neural network graph
def visualize_nn():
layers = define_layers()
colors = assign_colors()
G = nx.DiGraph()
pos = {}
node_colors = []
# Add nodes and assign positions
for i, (layer_name, nodes) in enumerate(layers.items()):
positions = calculate_positions(nodes, x_offset=i * 2)
for node, position in zip(nodes, positions):
G.add_node(node, layer=layer_name)
pos[node] = position
node_colors.append(colors.get(node, 'lightgray'))
# Add edges (automated for consecutive layers)
layer_names = list(layers.keys())
for i in range(len(layer_names) - 1):
source_layer, target_layer = layer_names[i], layer_names[i + 1]
for source in layers[source_layer]:
for target in layers[target_layer]:
G.add_edge(source, target)
# Draw the graph
plt.figure(figsize=(12, 8))
nx.draw(
G, pos, with_labels=True, node_color=node_colors, edge_color='gray',
node_size=3000, font_size=9, connectionstyle="arc3,rad=0.2"
)
plt.title("1-2, 1-2-3", fontsize=15)
plt.show()
# Run the visualization
visualize_nn()
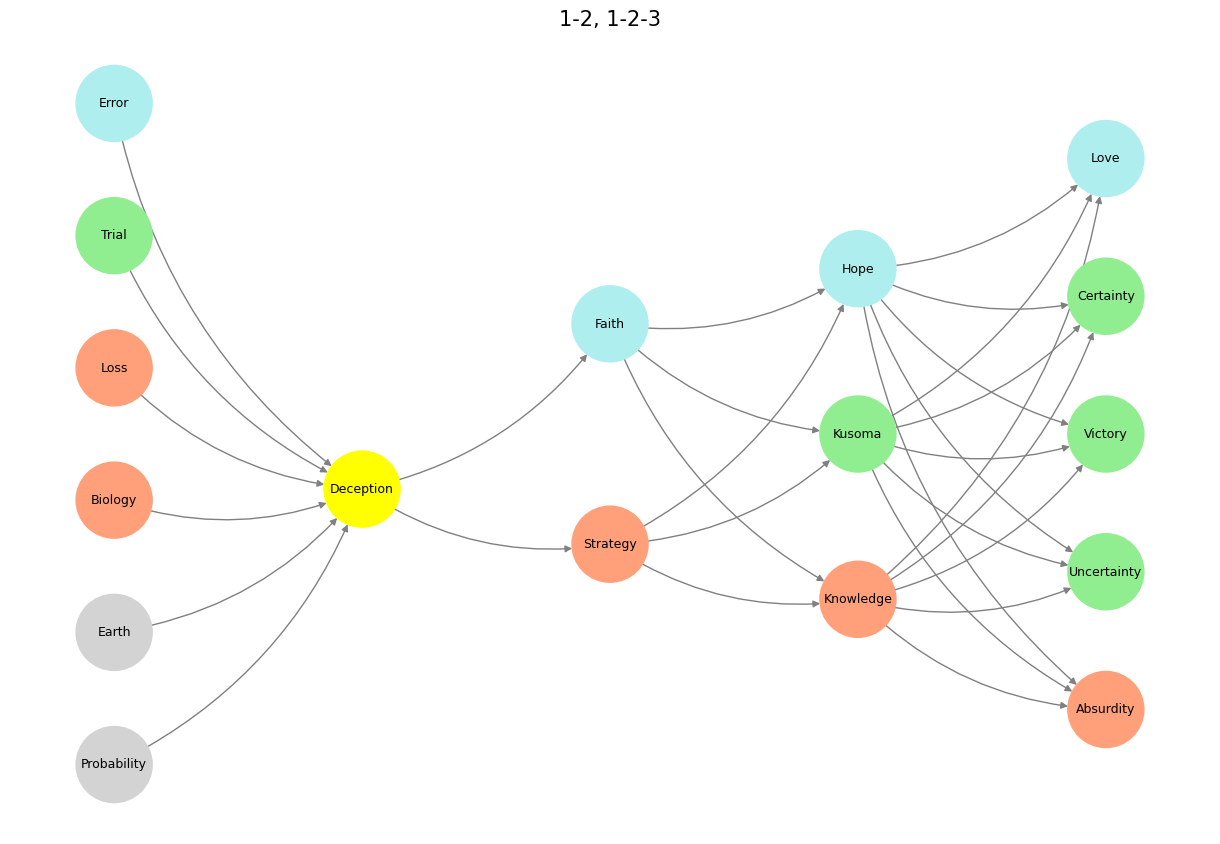
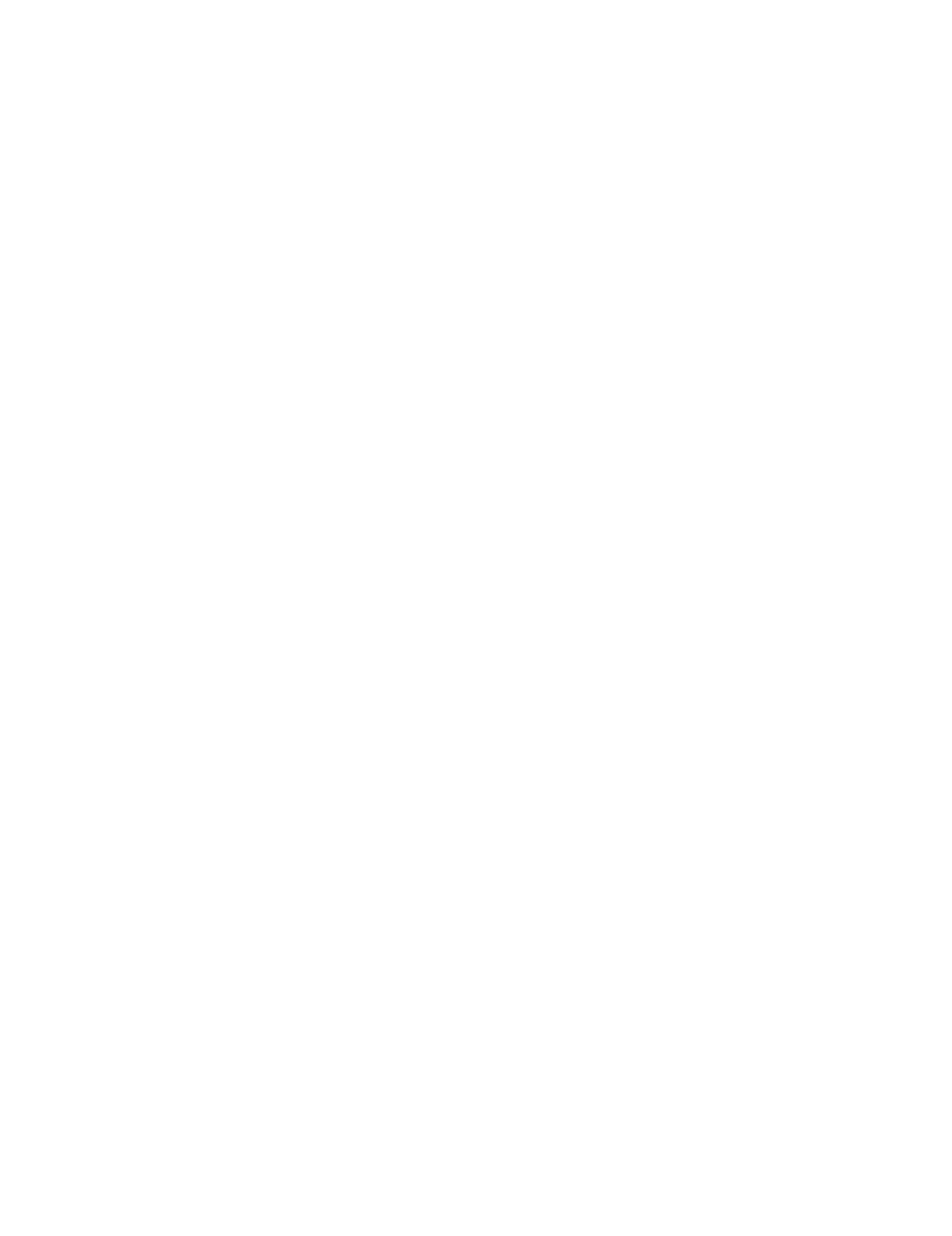
Fig. 35 While neural biology inspired neural networks in machine learning, the realization that scaling laws apply so beautifully to machine learning has led to a divergence in the process of generation of intelligence. Biology is constrained by the Red Queen, whereas mankind is quite open to destroying the Ecosystem-Cost function for the sake of generating the most powerful AI.#