Born to Etiquette#
Frailty in older age, as conceptualized by Linda Fried, can be understood as the loss of this very fractal complexity that underpins biological intelligence. The human body, like any intelligent system, is optimized for efficiency, with biological structures—capillaries, bronchioles, dendritic networks—acting as conduits of function that maximize exchange while minimizing energy expenditure. These systems are inherently fractal, meaning they maintain efficiency across multiple scales, from the microscopic to the macroscopic. However, as aging progresses, this fractal architecture begins to degrade, leading to inefficiencies that manifest as frailty.
Frailty is not merely the result of isolated system failures; rather, it is the breakdown of the body’s capacity to self-optimize across multiple domains. The vascular system, once a masterclass in last-mile delivery, loses its microcirculatory finesse, leading to impaired perfusion. The neural dendrites, once richly arborized for maximal synaptic plasticity, retract, reducing cognitive resilience and motor control. The musculoskeletal system, which once dynamically adapted to shifting demands, begins to lose its capacity for rapid repair and load redistribution, leading to sarcopenia and increased fall risk. These losses are not random but systematic, reflecting a progressive unraveling of the fractal complexity that once allowed the body to function with near-perfect efficiency.
See also
Fractal loss in aging is particularly devastating because it disrupts not only structure but also adaptability. The body’s ability to respond to stressors—be it an infection, a sudden drop in blood pressure, or even the act of walking across uneven terrain—depends on dynamic adjustments at multiple levels. In a younger, more complex system, small perturbations can be absorbed and compensated for without significant consequences. In an aging, less complex system, these same perturbations propagate unchecked, leading to cascading failures. This explains why frailty is often triggered by minor stressors: a simple urinary tract infection can precipitate delirium, a short hospital stay can result in loss of independence, and a mild fall can spiral into long-term immobility.
From an optimization standpoint, frailty can be seen as a failure to maintain an adaptive search space. The young body operates within a broad combinatorial space, with multiple solutions available to solve the same physiological problem—alternative metabolic pathways, compensatory neuromuscular adjustments, and immune redundancies. Aging, however, restricts this space. The body’s solutions become fewer, less efficient, and more fragile. Just as an AI model trained on a diminishing dataset loses its generalization capacity, the aging body, deprived of its structural and functional redundancies, becomes increasingly vulnerable to perturbations it once handled effortlessly.
Yet, the most striking parallel between frailty and AI failure is in energy efficiency. A fractal system distributes energy optimally, ensuring that resources reach the most critical areas with minimal waste. Aging, however, introduces inefficiencies: metabolic dysregulation leads to insulin resistance, mitochondrial dysfunction diminishes ATP production, and chronic inflammation saps resources from regenerative processes. What was once a tightly regulated energy landscape becomes an entropic system, with more resources spent on damage control than on maintaining function. This energy misallocation is the physiological equivalent of an AI model overfitting to noise—focusing on short-term survival rather than long-term adaptability.
Ultimately, frailty is the final stage of biological intelligence losing its ability to optimize. The fractal complexity that once enabled adaptability, resilience, and efficiency has eroded, leaving behind a system that is rigid, fragile, and prone to collapse under even minor stressors. Understanding frailty through this lens not only highlights the fundamental nature of aging but also suggests new avenues for intervention. If frailty is the loss of complexity, then maintaining or restoring fractal structures—whether through exercise, neural stimulation, or metabolic recalibration—may be the key to prolonging resilience. In the end, intelligence, whether artificial or biological, is only as strong as its ability to navigate complexity. Frailty is simply what happens when that capacity is lost.
Show code cell source
import numpy as np
import matplotlib.pyplot as plt
import networkx as nx
# Define the neural network layers
def define_layers():
return {
'Suis': ['Genome, 5%', 'Culture', 'Nourish It', 'Know It', "Move It", 'Injure It'], # Static
'Voir': ['Exposome, 15%'],
'Choisis': ['Metabolome, 50%', 'Basal Metabolic Rate'],
'Deviens': ['Unstructured-Intense', 'Weekly-Calendar', 'Proteome, 25%'],
"M'èléve": ['NexToken Prediction', 'Hydration', 'Fat-Muscle Ratio', 'Amor Fatì, 5%', 'Existential Cadence']
}
# Assign colors to nodes
def assign_colors():
color_map = {
'yellow': ['Exposome, 15%'],
'paleturquoise': ['Injure It', 'Basal Metabolic Rate', 'Proteome, 25%', 'Existential Cadence'],
'lightgreen': ["Move It", 'Weekly-Calendar', 'Hydration', 'Amor Fatì, 5%', 'Fat-Muscle Ratio'],
'lightsalmon': ['Nourish It', 'Know It', 'Metabolome, 50%', 'Unstructured-Intense', 'NexToken Prediction'],
}
return {node: color for color, nodes in color_map.items() for node in nodes}
# Define edge weights (hardcoded for editing)
def define_edges():
return {
('Genome, 5%', 'Exposome, 15%'): '1/99',
('Culture', 'Exposome, 15%'): '5/95',
('Nourish It', 'Exposome, 15%'): '20/80',
('Know It', 'Exposome, 15%'): '51/49',
("Move It", 'Exposome, 15%'): '80/20',
('Injure It', 'Exposome, 15%'): '95/5',
('Exposome, 15%', 'Metabolome, 50%'): '20/80',
('Exposome, 15%', 'Basal Metabolic Rate'): '80/20',
('Metabolome, 50%', 'Unstructured-Intense'): '49/51',
('Metabolome, 50%', 'Weekly-Calendar'): '80/20',
('Metabolome, 50%', 'Proteome, 25%'): '95/5',
('Basal Metabolic Rate', 'Unstructured-Intense'): '5/95',
('Basal Metabolic Rate', 'Weekly-Calendar'): '20/80',
('Basal Metabolic Rate', 'Proteome, 25%'): '51/49',
('Unstructured-Intense', 'NexToken Prediction'): '80/20',
('Unstructured-Intense', 'Hydration'): '85/15',
('Unstructured-Intense', 'Fat-Muscle Ratio'): '90/10',
('Unstructured-Intense', 'Amor Fatì, 5%'): '95/5',
('Unstructured-Intense', 'Existential Cadence'): '99/1',
('Weekly-Calendar', 'NexToken Prediction'): '1/9',
('Weekly-Calendar', 'Hydration'): '1/8',
('Weekly-Calendar', 'Fat-Muscle Ratio'): '1/7',
('Weekly-Calendar', 'Amor Fatì, 5%'): '1/6',
('Weekly-Calendar', 'Existential Cadence'): '1/5',
('Proteome, 25%', 'NexToken Prediction'): '1/99',
('Proteome, 25%', 'Hydration'): '5/95',
('Proteome, 25%', 'Fat-Muscle Ratio'): '10/90',
('Proteome, 25%', 'Amor Fatì, 5%'): '15/85',
('Proteome, 25%', 'Existential Cadence'): '20/80'
}
# Calculate positions for nodes
def calculate_positions(layer, x_offset):
y_positions = np.linspace(-len(layer) / 2, len(layer) / 2, len(layer))
return [(x_offset, y) for y in y_positions]
# Create and visualize the neural network graph
def visualize_nn():
layers = define_layers()
colors = assign_colors()
edges = define_edges()
G = nx.DiGraph()
pos = {}
node_colors = []
# Create mapping from original node names to numbered labels
mapping = {}
counter = 1
for layer in layers.values():
for node in layer:
mapping[node] = f"{counter}. {node}"
counter += 1
# Add nodes with new numbered labels and assign positions
for i, (layer_name, nodes) in enumerate(layers.items()):
positions = calculate_positions(nodes, x_offset=i * 2)
for node, position in zip(nodes, positions):
new_node = mapping[node]
G.add_node(new_node, layer=layer_name)
pos[new_node] = position
node_colors.append(colors.get(node, 'lightgray'))
# Add edges with updated node labels
for (source, target), weight in edges.items():
if source in mapping and target in mapping:
new_source = mapping[source]
new_target = mapping[target]
G.add_edge(new_source, new_target, weight=weight)
# Draw the graph
plt.figure(figsize=(12, 8))
edges_labels = {(u, v): d["weight"] for u, v, d in G.edges(data=True)}
nx.draw(
G, pos, with_labels=True, node_color=node_colors, edge_color='gray',
node_size=3000, font_size=9, connectionstyle="arc3,rad=0.2"
)
nx.draw_networkx_edge_labels(G, pos, edge_labels=edges_labels, font_size=8)
plt.title("OPRAH™: Frailty, Fractals, Factorials", fontsize=25)
plt.show()
# Run the visualization
visualize_nn()
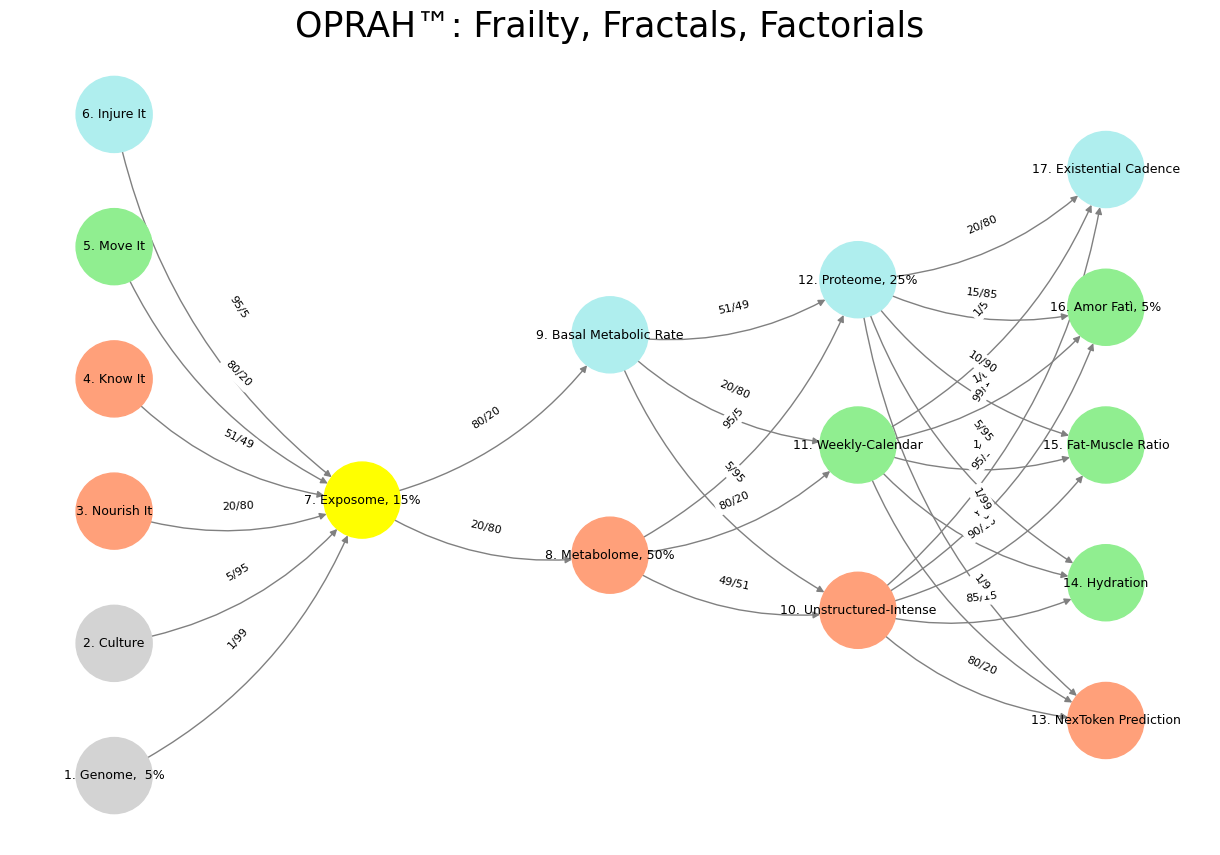
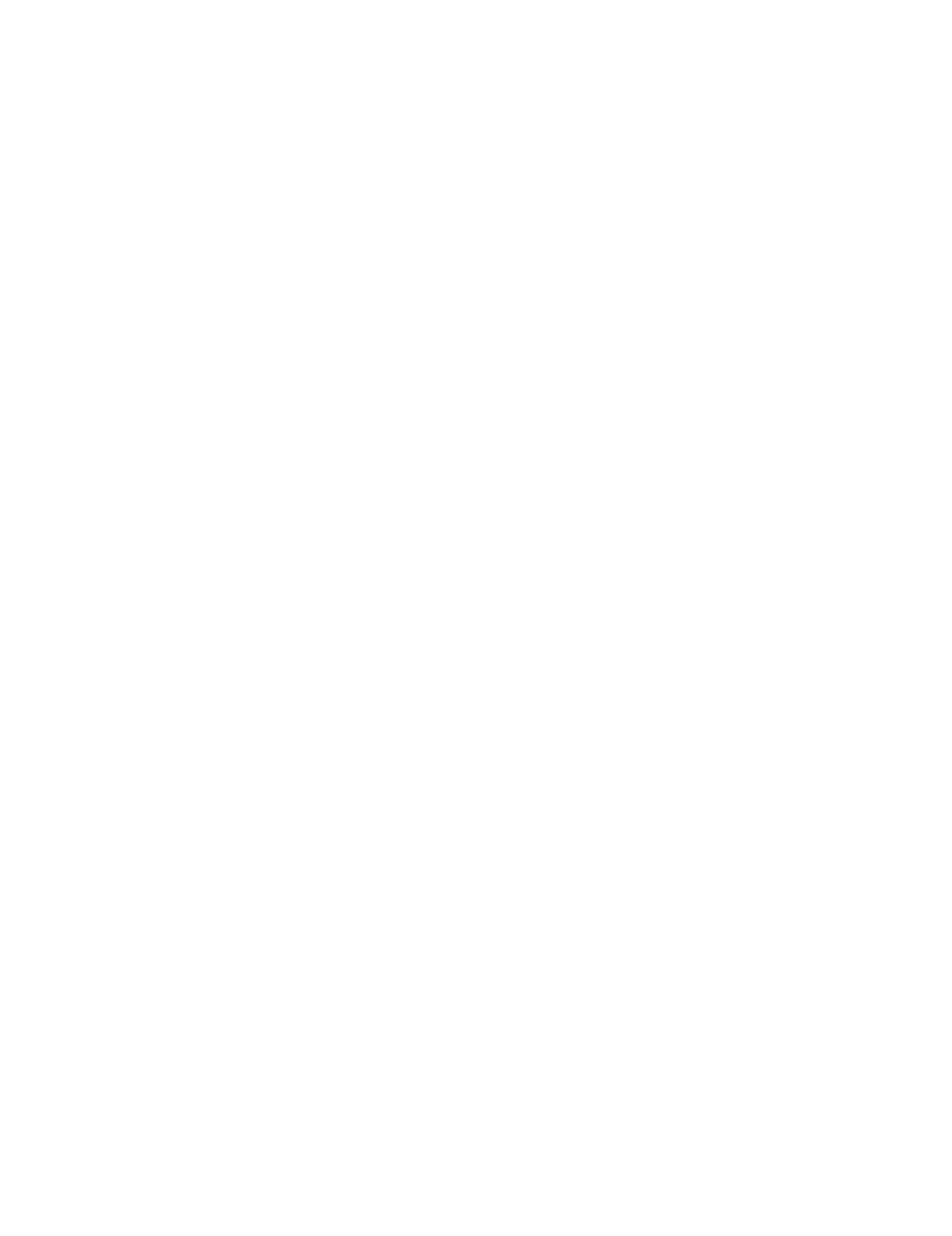
Fig. 36 Glenn Gould and Leonard Bernstein famously disagreed over the tempo and interpretation of Brahms’ First Piano Concerto during a 1962 New York Philharmonic concert, where Bernstein, conducting, publicly distanced himself from Gould’s significantly slower-paced interpretation before the performance began, expressing his disagreement with the unconventional approach while still allowing Gould to perform it as planned; this event is considered one of the most controversial moments in classical music history.#