Revolution#
Fig. 25 What Exactly is Identity. A node may represent goats (in general) and another sheep (in general). But the identity of any specific animal (not its species) is a network. For this reason we might have a “black sheep”, distinct in certain ways – perhaps more like a goat than other sheep. But that’s all dull stuff. Mistaken identity is often the fuel of comedy, usually when the adversarial is mistaken for the cooperative or even the transactional.#
Show code cell source
import numpy as np
import matplotlib.pyplot as plt
import networkx as nx
# Define the neural network layers
def define_layers():
return {
'Suis': ['Genome, 5%', 'Culture', 'Nourish It', 'Know It', "Move It", 'Injure It'], # Static
'Voir': ['Exposome, 15%'],
'Choisis': ['Metabolome, 50%', 'Basal Metabolic Rate'],
'Deviens': ['Unstructured-Intense', 'Weekly-Calendar', 'Proteome, 25%'],
"M'èléve": ['NexToken Prediction', 'Hydration', 'Fat-Muscle Ratio', 'Amor Fatì, 5%', 'Existential Cadence']
}
# Assign colors to nodes
def assign_colors():
color_map = {
'yellow': ['Exposome, 15%'],
'paleturquoise': ['Injure It', 'Basal Metabolic Rate', 'Proteome, 25%', 'Existential Cadence'],
'lightgreen': ["Move It", 'Weekly-Calendar', 'Hydration', 'Amor Fatì, 5%', 'Fat-Muscle Ratio'],
'lightsalmon': ['Nourish It', 'Know It', 'Metabolome, 50%', 'Unstructured-Intense', 'NexToken Prediction'],
}
return {node: color for color, nodes in color_map.items() for node in nodes}
# Define edge weights (hardcoded for editing)
def define_edges():
return {
('Genome, 5%', 'Exposome, 15%'): '1/99',
('Culture', 'Exposome, 15%'): '5/95',
('Nourish It', 'Exposome, 15%'): '20/80',
('Know It', 'Exposome, 15%'): '51/49',
("Move It", 'Exposome, 15%'): '80/20',
('Injure It', 'Exposome, 15%'): '95/5',
('Exposome, 15%', 'Metabolome, 50%'): '20/80',
('Exposome, 15%', 'Basal Metabolic Rate'): '80/20',
('Metabolome, 50%', 'Unstructured-Intense'): '49/51',
('Metabolome, 50%', 'Weekly-Calendar'): '80/20',
('Metabolome, 50%', 'Proteome, 25%'): '95/5',
('Basal Metabolic Rate', 'Unstructured-Intense'): '5/95',
('Basal Metabolic Rate', 'Weekly-Calendar'): '20/80',
('Basal Metabolic Rate', 'Proteome, 25%'): '51/49',
('Unstructured-Intense', 'NexToken Prediction'): '80/20',
('Unstructured-Intense', 'Hydration'): '85/15',
('Unstructured-Intense', 'Fat-Muscle Ratio'): '90/10',
('Unstructured-Intense', 'Amor Fatì, 5%'): '95/5',
('Unstructured-Intense', 'Existential Cadence'): '99/1',
('Weekly-Calendar', 'NexToken Prediction'): '1/9',
('Weekly-Calendar', 'Hydration'): '1/8',
('Weekly-Calendar', 'Fat-Muscle Ratio'): '1/7',
('Weekly-Calendar', 'Amor Fatì, 5%'): '1/6',
('Weekly-Calendar', 'Existential Cadence'): '1/5',
('Proteome, 25%', 'NexToken Prediction'): '1/99',
('Proteome, 25%', 'Hydration'): '5/95',
('Proteome, 25%', 'Fat-Muscle Ratio'): '10/90',
('Proteome, 25%', 'Amor Fatì, 5%'): '15/85',
('Proteome, 25%', 'Existential Cadence'): '20/80'
}
# Calculate positions for nodes
def calculate_positions(layer, x_offset):
y_positions = np.linspace(-len(layer) / 2, len(layer) / 2, len(layer))
return [(x_offset, y) for y in y_positions]
# Create and visualize the neural network graph
def visualize_nn():
layers = define_layers()
colors = assign_colors()
edges = define_edges()
G = nx.DiGraph()
pos = {}
node_colors = []
# Create mapping from original node names to numbered labels
mapping = {}
counter = 1
for layer in layers.values():
for node in layer:
mapping[node] = f"{counter}. {node}"
counter += 1
# Add nodes with new numbered labels and assign positions
for i, (layer_name, nodes) in enumerate(layers.items()):
positions = calculate_positions(nodes, x_offset=i * 2)
for node, position in zip(nodes, positions):
new_node = mapping[node]
G.add_node(new_node, layer=layer_name)
pos[new_node] = position
node_colors.append(colors.get(node, 'lightgray'))
# Add edges with updated node labels
for (source, target), weight in edges.items():
if source in mapping and target in mapping:
new_source = mapping[source]
new_target = mapping[target]
G.add_edge(new_source, new_target, weight=weight)
# Draw the graph
plt.figure(figsize=(12, 8))
edges_labels = {(u, v): d["weight"] for u, v, d in G.edges(data=True)}
nx.draw(
G, pos, with_labels=True, node_color=node_colors, edge_color='gray',
node_size=3000, font_size=9, connectionstyle="arc3,rad=0.2"
)
nx.draw_networkx_edge_labels(G, pos, edge_labels=edges_labels, font_size=8)
plt.title("OPRAH™: Heredity, Lifestyle, Badluck", fontsize=25)
plt.show()
# Run the visualization
visualize_nn()
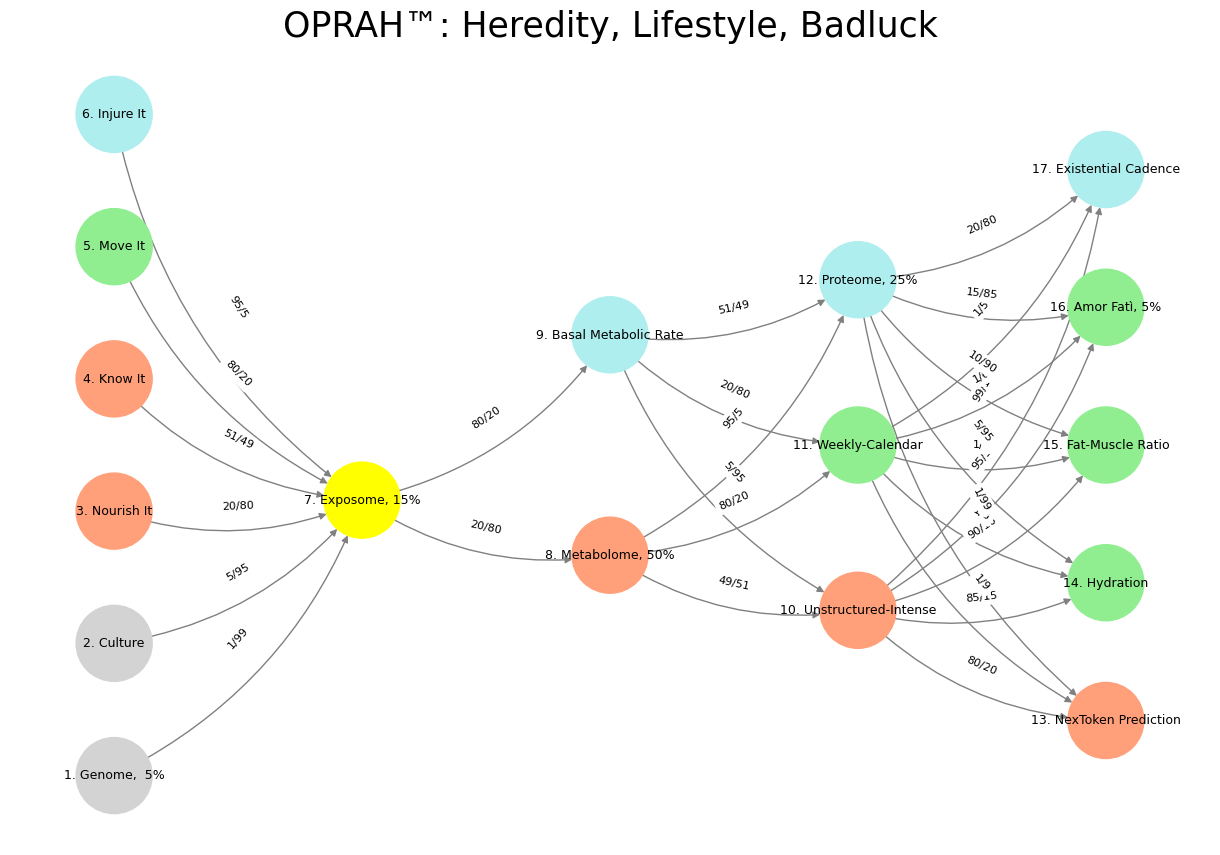

Fig. 26 Francis Bacon. He famously stated “If a man will begin with certainties, he shall end in doubts; but if he will be content to begin with doubts, he shall end in certainties.” This quote is from The Advancement of Learning (1605), where Bacon lays out his vision for empirical science and inductive reasoning. He argues that starting with unquestioned assumptions leads to instability and confusion, whereas a methodical approach that embraces doubt and inquiry leads to true knowledge. This aligns with his broader Novum Organum (1620), where he develops the Baconian method, advocating for systematic observation, experimentation, and the gradual accumulation of knowledge rather than relying on dogma or preconceived notions.#