Traditional#
Fig. 33 Akia Kurasawa: Why Canβt People Be Happy Together? This was a fork in the road for human civilization. Our dear planet earth now becomes just but an optional resource on which we jostle for resources. By expanding to Mars, the jostle reduces for perhaps a couple of centuries of millenia. There need to be things that inspire you. Things that make you glad to wake up in the morning and say βIβm looking forward to the future.β And until then, we have gym and coffee β or perhaps gin & juice. We are going to have a golden age. One of the American values that I love is optimism. We are going to make the future good.#
Show code cell source
import numpy as np
import matplotlib.pyplot as plt
import networkx as nx
# Define the neural network fractal
def define_layers():
return {
'World': ['Cosmos-Entropy', 'World-Tempered', 'Ucubona-Needs', 'Ecosystem-Costs', 'Space-Trial & Error', 'Time-Cadence', ], # Veni; 95/5
'Mode': ['Ucubona-Mode'], # Vidi; 80/20
'Agent': ['Oblivion-Unknown', 'Brand-Trusted'], # Vici; Veni; 51/49
'Space': ['Ratio-Weaponized', 'Competition-Tokenized', 'Odds-Monopolized'], # Vidi; 20/80
'Time': ['Volatile-Transvaluation', 'Unveiled-Resentment', 'Freedom-Dance in Chains', 'Exuberant-Jubilee', 'Stable-Victorian'] # Vici; 5/95
}
# Assign colors to nodes
def assign_colors():
color_map = {
'yellow': ['Ucubona-Mode'],
'paleturquoise': ['Time-Cadence', 'Brand-Trusted', 'Odds-Monopolized', 'Stable-Victorian'],
'lightgreen': ['Space-Trial & Error', 'Competition-Tokenized', 'Exuberant-Jubilee', 'Freedom-Dance in Chains', 'Unveiled-Resentment'],
'lightsalmon': [
'Ucubona-Needs', 'Ecosystem-Costs', 'Oblivion-Unknown',
'Ratio-Weaponized', 'Volatile-Transvaluation'
],
}
return {node: color for color, nodes in color_map.items() for node in nodes}
# Calculate positions for nodes
def calculate_positions(layer, x_offset):
y_positions = np.linspace(-len(layer) / 2, len(layer) / 2, len(layer))
return [(x_offset, y) for y in y_positions]
# Create and visualize the neural network graph
def visualize_nn():
layers = define_layers()
colors = assign_colors()
G = nx.DiGraph()
pos = {}
node_colors = []
# Add nodes and assign positions
for i, (layer_name, nodes) in enumerate(layers.items()):
positions = calculate_positions(nodes, x_offset=i * 2)
for node, position in zip(nodes, positions):
G.add_node(node, layer=layer_name)
pos[node] = position
node_colors.append(colors.get(node, 'lightgray'))
# Add edges (automated for consecutive layers)
layer_names = list(layers.keys())
for i in range(len(layer_names) - 1):
source_layer, target_layer = layer_names[i], layer_names[i + 1]
for source in layers[source_layer]:
for target in layers[target_layer]:
G.add_edge(source, target)
# Draw the graph
plt.figure(figsize=(12, 8))
nx.draw(
G, pos, with_labels=True, node_color=node_colors, edge_color='gray',
node_size=3000, font_size=9, connectionstyle="arc3,rad=0.2"
)
plt.title("Veni, Vidi, Vici", fontsize=15)
plt.show()
# Run the visualization
visualize_nn()
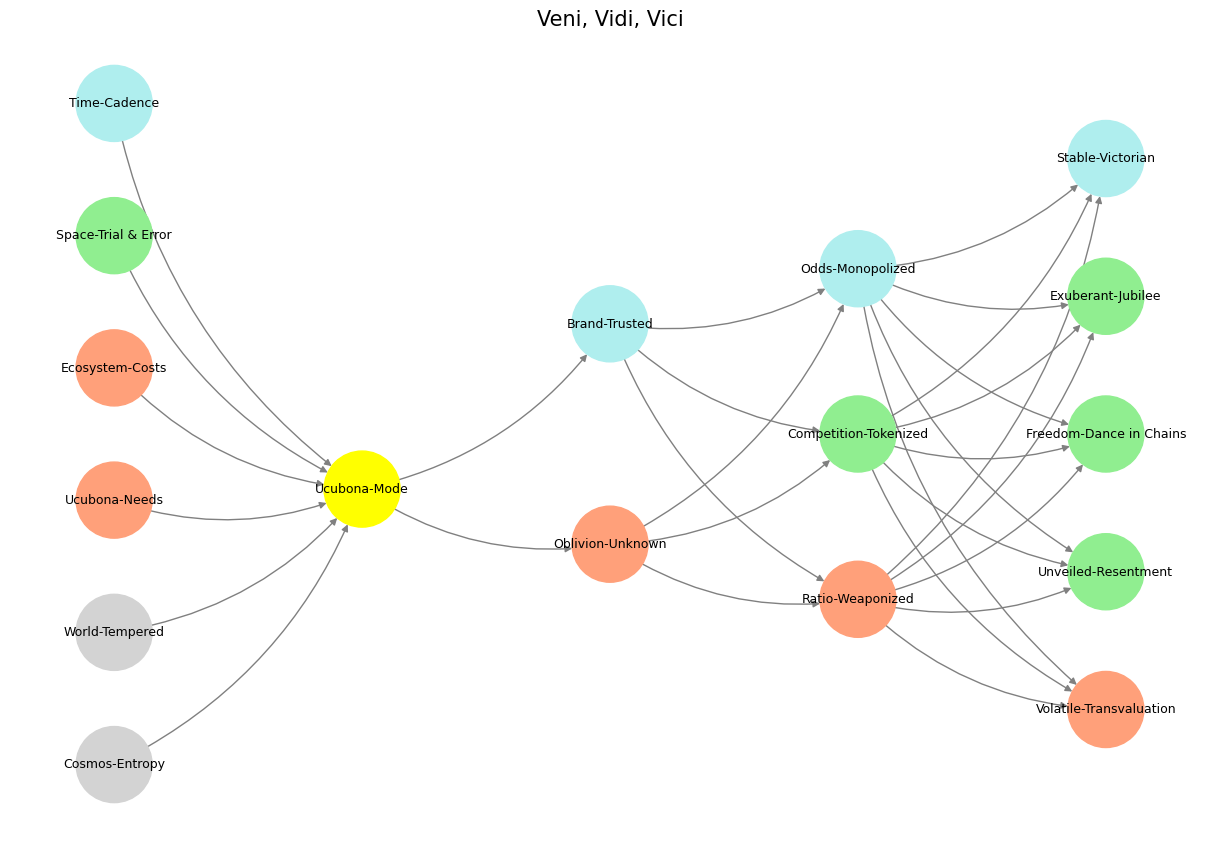
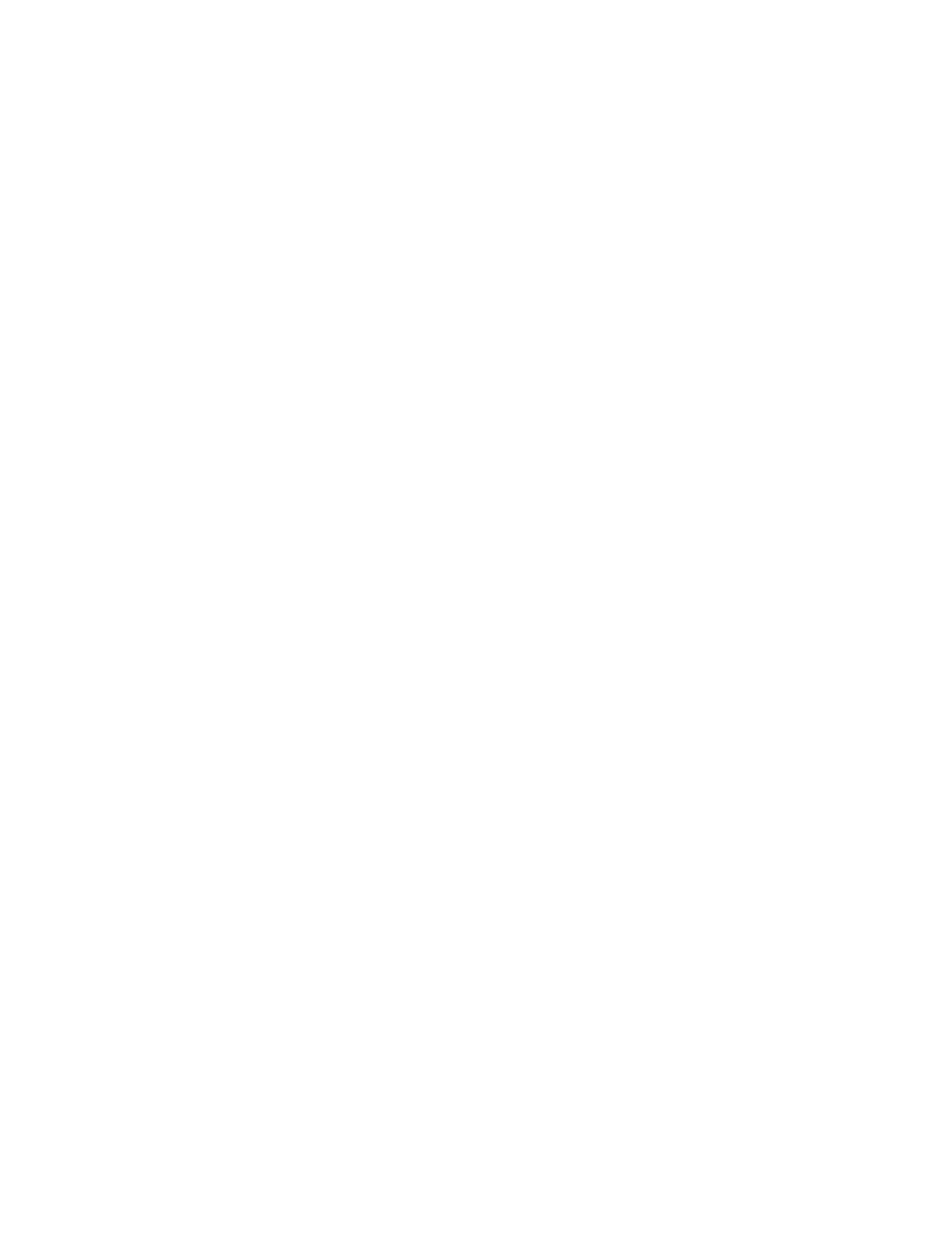
Fig. 34 While neural biology inspired neural networks in machine learning, the realization that scaling laws apply so beautifully to machine learning has led to a divergence in the process of generation of intelligence. Biology is constrained by the Red Queen, whereas mankind is quite open to destroying the Ecosystem-Cost function for the sake of generating the most powerful AI.#