ESRD#
The phrase “throw a bone to chew on” typically means giving someone something to occupy their attention or to think about. It’s often used metaphorically, derived from the literal action of giving a dog a bone to keep it busy and satisfied. This phrase can be used in various contexts:
Distraction or appeasement: In politics or negotiations, it might mean offering a small concession or minor issue to distract from the more significant matters at hand. For example, a politician might announce a minor policy change to appease the public while avoiding more substantial reforms.
Intellectual stimulation: In discussions or debates, it can refer to providing a challenging idea or topic for someone to ponder or debate. For example, a teacher might present a complex problem to students to stimulate critical thinking and discussion.
Provision of a minor benefit: In the workplace, a manager might give a small perk or benefit to employees to keep them content and focused on their tasks, without making major changes to their workload or working conditions.
Opinions and Thoughts:
Appeasement in politics: This usage often reflects a cynical view of political maneuvering. It suggests that those in power might use minor concessions to pacify dissent or distract from more significant issues. This can be seen as manipulative, and it may eventually backfire if the public perceives the gesture as insincere or insufficient.
Intellectual stimulation: When used in an educational or intellectual context, this phrase has a more positive connotation. Providing challenging problems or ideas can foster growth, creativity, and critical thinking. It shows respect for the intellectual capacities of others and encourages deeper engagement with the material.
Workplace morale: In the context of employee management, the phrase can highlight a tension between short-term appeasement and long-term satisfaction. Small perks might boost morale temporarily, but they need to be part of a broader strategy for employee engagement and well-being. Otherwise, they might be seen as token gestures that don’t address underlying issues.
Ultimately, the effectiveness and ethical implications of “throwing a bone to chew on” depend on the context and the intentions behind the gesture. In all cases, it’s crucial to consider whether the action genuinely addresses the needs and concerns of those involved or if it’s merely a superficial tactic.
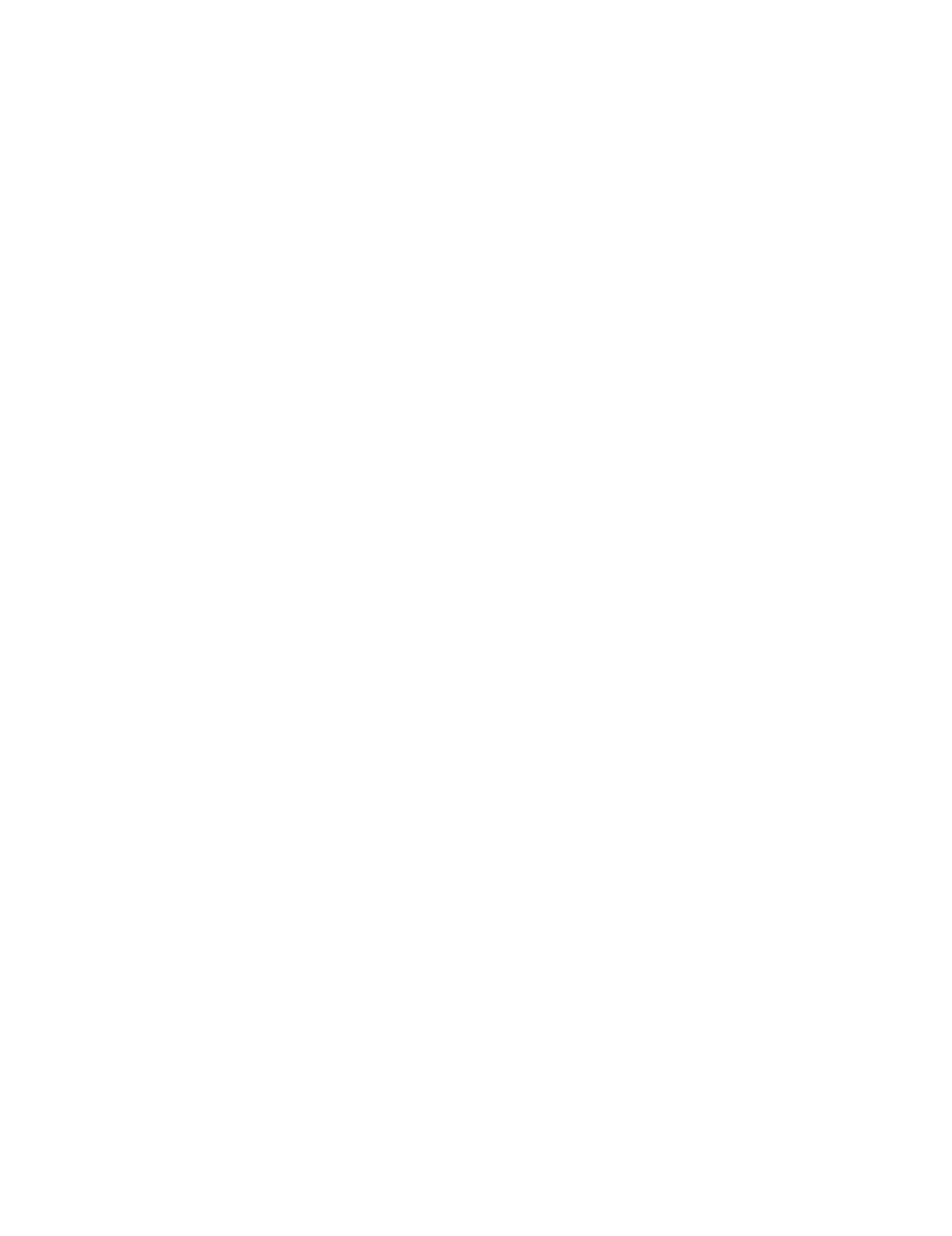
Fig. 2 Pretty Simple Music. I intuitive trust this instructor. He’s developed 5 levels of passing chords
. Any one who has encoded the essence of things knows how to categorize and rank them in a didactic hierarchy#