Prometheus#
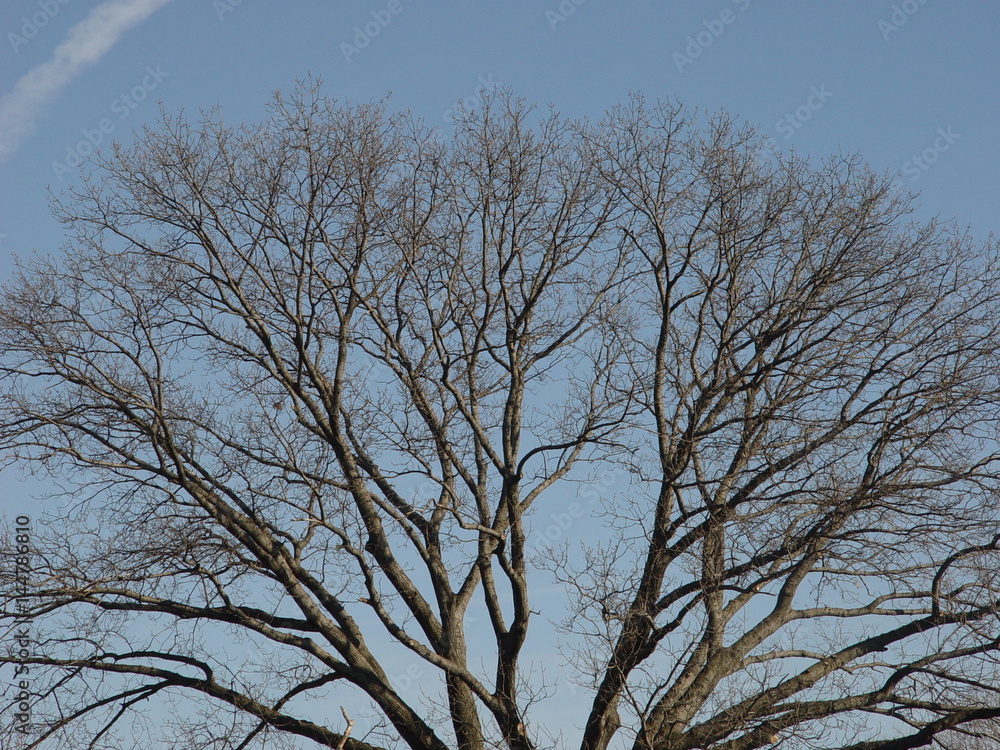
The economic intelligence of nature: a visualization of dendritic structures, evoking parallels with neural networks, tree branches, and respiratory bronchioles.
Entropy: Wisdom (Streets)
Resources: Vigilance (Owl)
Faustian: Noise (Molecule) vs. Signal (Epitope)
Distributed: Self (Helmet), Negotiable (Shield), Nonself (Spear)
Order: Harmony (Lyre)
β Inverted Tree

..evoking parallels with neural networks, tree branches, and Visualization of dendritic structures, evoking parallels with neural networks, tree branches, and respiratory bronchioles.
Show code cell source
import numpy as np
import matplotlib.pyplot as plt
import networkx as nx
# Define the neural network layers
def define_layers():
return {
'Tragedy (Pattern Recognition)': ['Cosmology', 'Geology', 'Biology', 'Ecology', "Symbiotology", 'Teleology'],
'History (Resources)': ['Resources'],
'Epic (Negotiated Identity)': ['Faustian Bargain', 'Islamic Finance'],
'Drama (Self vs. Non-Self)': ['Darabah', 'Sharakah', 'Takaful'],
"Comedy (Resolution)": ['Cacophony', 'Outside', 'Ukhuwah', 'Inside', 'Symphony']
}
# Assign colors to nodes
def assign_colors():
color_map = {
'yellow': ['Resources'],
'paleturquoise': ['Teleology', 'Islamic Finance', 'Takaful', 'Symphony'],
'lightgreen': ["Symbiotology", 'Sharakah', 'Outside', 'Inside', 'Ukhuwah'],
'lightsalmon': ['Biology', 'Ecology', 'Faustian Bargain', 'Darabah', 'Cacophony'],
}
return {node: color for color, nodes in color_map.items() for node in nodes}
# Define edges
def define_edges():
return [
('Cosmology', 'Resources'),
('Geology', 'Resources'),
('Biology', 'Resources'),
('Ecology', 'Resources'),
("Symbiotology", 'Resources'),
('Teleology', 'Resources'),
('Resources', 'Faustian Bargain'),
('Resources', 'Islamic Finance'),
('Faustian Bargain', 'Darabah'),
('Faustian Bargain', 'Sharakah'),
('Faustian Bargain', 'Takaful'),
('Islamic Finance', 'Darabah'),
('Islamic Finance', 'Sharakah'),
('Islamic Finance', 'Takaful'),
('Darabah', 'Cacophony'),
('Darabah', 'Outside'),
('Darabah', 'Ukhuwah'),
('Darabah', 'Inside'),
('Darabah', 'Symphony'),
('Sharakah', 'Cacophony'),
('Sharakah', 'Outside'),
('Sharakah', 'Ukhuwah'),
('Sharakah', 'Inside'),
('Sharakah', 'Symphony'),
('Takaful', 'Cacophony'),
('Takaful', 'Outside'),
('Takaful', 'Ukhuwah'),
('Takaful', 'Inside'),
('Takaful', 'Symphony')
]
# Define black edges (1 β 7 β 9 β 11 β [13-17])
black_edges = [
(4, 7), (7, 9), (9, 11), (11, 13), (11, 14), (11, 15), (11, 16), (11, 17)
]
# Calculate node positions
def calculate_positions(layer, x_offset):
y_positions = np.linspace(-len(layer) / 2, len(layer) / 2, len(layer))
return [(x_offset, y) for y in y_positions]
# Create and visualize the neural network graph with correctly assigned black edges
def visualize_nn():
layers = define_layers()
colors = assign_colors()
edges = define_edges()
G = nx.DiGraph()
pos = {}
node_colors = []
# Create mapping from original node names to numbered labels
mapping = {}
counter = 1
for layer in layers.values():
for node in layer:
mapping[node] = f"{counter}. {node}"
counter += 1
# Add nodes with new numbered labels and assign positions
for i, (layer_name, nodes) in enumerate(layers.items()):
positions = calculate_positions(nodes, x_offset=i * 2)
for node, position in zip(nodes, positions):
new_node = mapping[node]
G.add_node(new_node, layer=layer_name)
pos[new_node] = position
node_colors.append(colors.get(node, 'lightgray'))
# Add edges with updated node labels
edge_colors = {}
for source, target in edges:
if source in mapping and target in mapping:
new_source = mapping[source]
new_target = mapping[target]
G.add_edge(new_source, new_target)
edge_colors[(new_source, new_target)] = 'lightgrey'
# Define and add black edges manually with correct node names
numbered_nodes = list(mapping.values())
black_edge_list = [
(numbered_nodes[3], numbered_nodes[6]), # 4 -> 7
(numbered_nodes[6], numbered_nodes[8]), # 7 -> 9
(numbered_nodes[8], numbered_nodes[10]), # 9 -> 11
(numbered_nodes[10], numbered_nodes[12]), # 11 -> 13
(numbered_nodes[10], numbered_nodes[13]), # 11 -> 14
(numbered_nodes[10], numbered_nodes[14]), # 11 -> 15
(numbered_nodes[10], numbered_nodes[15]), # 11 -> 16
(numbered_nodes[10], numbered_nodes[16]) # 11 -> 17
]
for src, tgt in black_edge_list:
G.add_edge(src, tgt)
edge_colors[(src, tgt)] = 'black'
# Draw the graph
plt.figure(figsize=(12, 8))
nx.draw(
G, pos, with_labels=True, node_color=node_colors,
edge_color=[edge_colors.get(edge, 'lightgrey') for edge in G.edges],
node_size=3000, font_size=9, connectionstyle="arc3,rad=0.2"
)
plt.title("Self-Similar Micro-Decisions", fontsize=18)
plt.show()
# Run the visualization
visualize_nn()
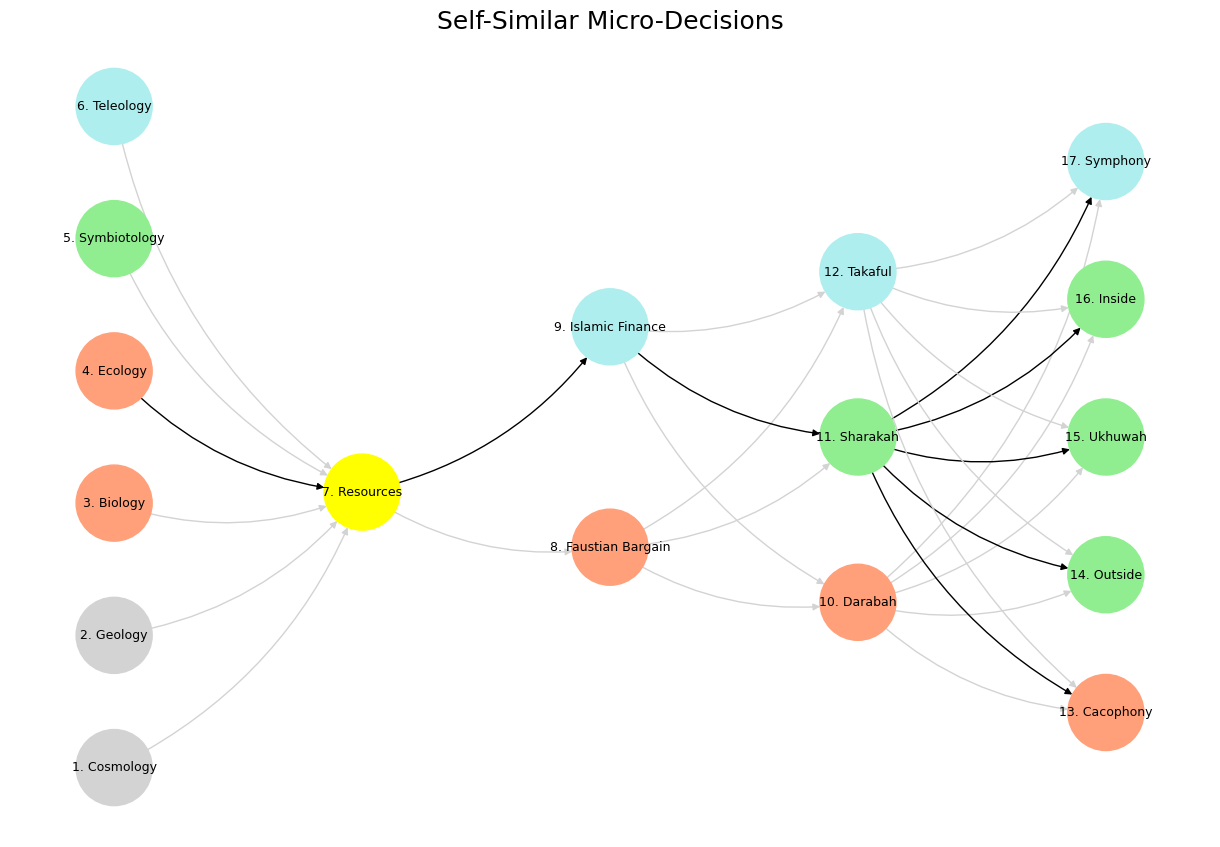
Fig. 8 Across diverse domains of biology and computation, branching structures appear as a universal design principle. From the fractal-like bifurcations of trees to the dendritic networks of neurons, and even the bronchioles within the lungs, nature employs efficient, scalable, and self-similar architectures to optimize function. These structures facilitate rapid information transfer, efficient oxygen distribution, and recursive connectivityβechoing similar principles in artificial neural networks and computational models. This shared geometry underscores the fundamental economy of nature, where optimal design principles are preserved across scales, from micro to macro systems. So, too, with CG-BEST and the Bardβs oeuvre!#