Cunning Strategy#
In the context of our neural network model, where information, in this case, the cultural and intellectual currents of the 17th century, flows through nodes representing various facets of human endeavor, we can analyze Charles IIās reaction to coffeehouses through the lens of the divine versus the cosmic, especially during an era where Isaac Newton was beginning to reshape human understanding of the universe.
Input Layer: The Immutable Laws (Entropy, Gravity): This layer symbolizes the foundational principles that were being redefined in Charles IIās time. Isaac Newton, with his laws of motion and universal gravitation, was shifting the paradigm from a purely divine explanation of the cosmos to one that included mechanical, observable laws. This shift was not just scientific but deeply philosophical, challenging traditional religious views of a purely divine-ordered universe.
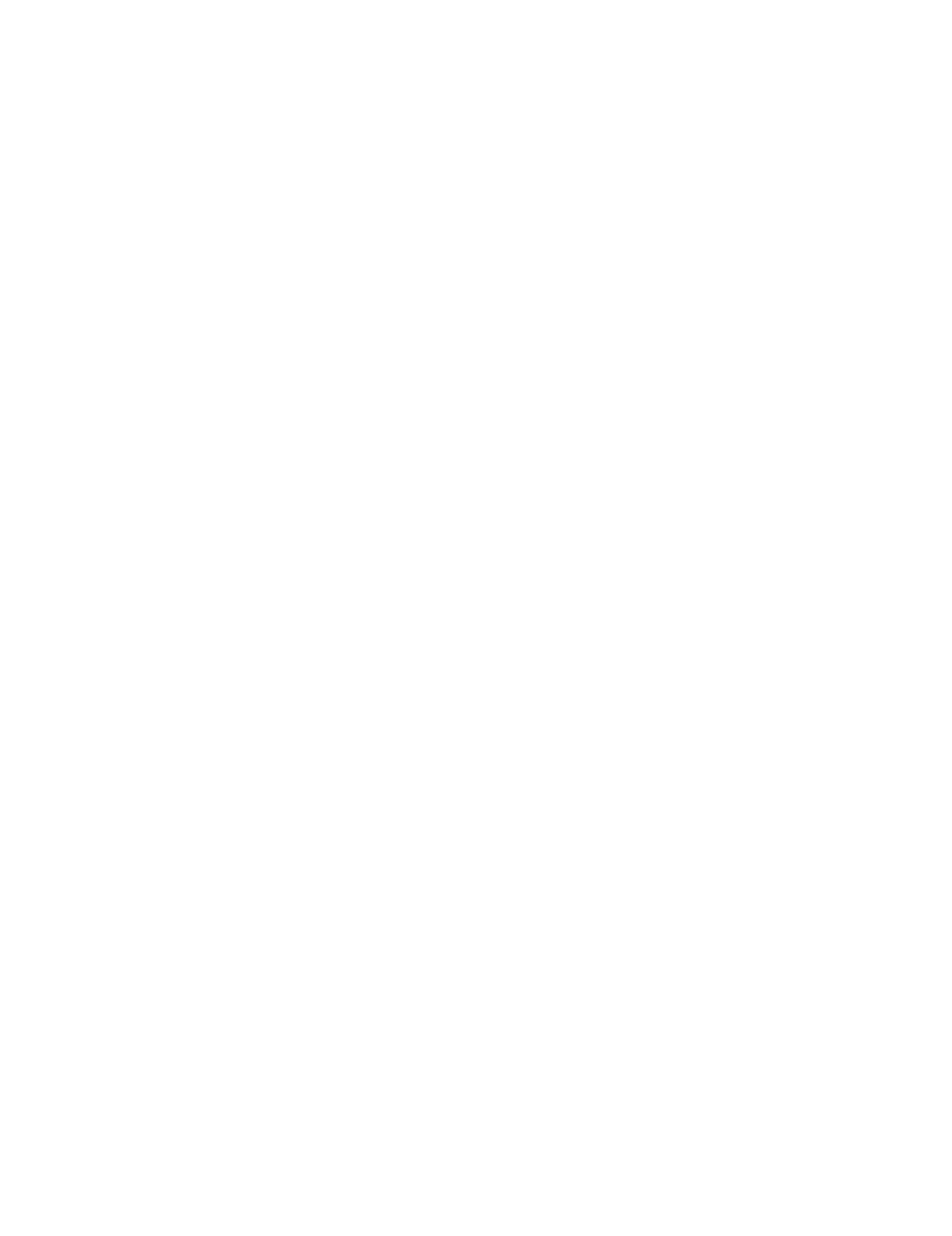
Fig. 10 Competition (Generativity) & Feedback (Exertion). The massive combinatorial search space and optimized emergent behavioral from āgamesā among the gods, animals, and machines are the way evolution has worked from the beginning of time. Peterson believes reinforcement learning through human feedback (RLHF- a post-training twitch) removes this evolutionary imperative.#
Layer 1 - World: The world of Charles II was one where the divine right of kings was still a potent political concept, yet it was being subtly undermined by the rising tide of scientific inquiry. Coffeehouses, thriving in this era, became the nodes where these new ideas were discussed, debated, and disseminated. They represented a microcosm of the world where the old divine order was coming into conflict with the new cosmic understanding.
Layer 2 - Perception: The perception of coffeehouses in London was dualistic. On one hand, they were seen as centers of enlightenment, knowledge, and social interaction, paralleling the cafes of the Ottoman Empire. They were places where the Newtonian revolutionās implications could be pondered, where men of science, like Newton himself, might discuss the latest developments away from the prying eyes of the crown or church. On the other hand, Charles II perceived these establishments as threats; they were hubs where political dissent could be organized, where loyalty to the divine right of kings could be questioned in light of the new cosmic laws.
Layer 3 - Agency: Here, agency refers to the power to influence, and coffeehouses granted this to the common man in ways that were unprecedented. These were places where anyone could speak, and thus, where ideas could challenge the royal and ecclesiastical authorities. Charles IIās attempt to close coffeehouses with his 1675 proclamation was an act of reclaiming agency, trying to reassert control over the narrative of divine versus cosmic order. His reaction was a direct response to the fear that the populace might prefer the laws of nature over the laws of God and King.
Layer 4 - Generative: The generative aspect in this network is the creation of new ideas, social structures, and even revolutions. Coffeehouses were generative spaces where scientific discourse led to societal change. The tension between the divine and cosmic was palpable; for instance, Newtonās work could be seen as either glorifying God by revealing His laws or diminishing divine mystery by explaining the universe through mechanics. The coffeehouse culture was generative of a new worldview, one that Charles II felt directly threatened his divine authority.
Layer 5 - Physical (Feedback into the World): The backlash against Charles IIās coffeehouse ban, leading to its quick retraction, illustrates the feedback loop in our neural network. The populaceās reaction was a physical manifestation of the new cosmic orderās influence over the old divine one. This feedback reinforced the societal shift towards a more questioning, scientific mindset. The failure of Charles II to maintain control over this discourse highlighted how the Enlightenment was beginning to shape governance and public life, with coffee as a catalyst.
In this era, where Newtonās scientific work was beginning to filter into public consciousness, coffeehouses were not just places for caffeine consumption but were nodes in a societal network where the clash between the divine right of kings and the emerging cosmic laws of the universe played out. Charles IIās attempt to suppress coffeehouse culture was a reaction to this shift, an effort to preserve a world where divine authority was paramount over the cosmic understanding that was beginning to dawn. The kingās actions were a direct response to the threat he perceived from this new intellectual freedom, which could challenge not just his rule but the very foundation of divine ordinance in governance.
Show code cell source
import numpy as np
import matplotlib.pyplot as plt
import networkx as nx
# Define the neural network fractal
def define_layers():
return {
'World': ['Cosmos', 'Planet', 'Life', 'Ecosystem', 'Generative', 'Cartel', ], ## Cosmos, Planet
'Perception': ['Perception'], # Life
'Agency': ['Open', 'Closed'], # Ecosystem (Beyond Principal-Agent-Other)
'Generative': ['Ratio', 'Competition', 'Odds'], # Generative
'Physical': ['Volatile-Distributed', 'Unknown', 'Freedom', 'Known', 'Stable-Central'] # Physical
}
# Assign colors to nodes
def assign_colors():
color_map = {
'yellow': ['Perception'],
'paleturquoise': ['Cartel', 'Closed', 'Odds', 'Stable-Central'],
'lightgreen': ['Generative', 'Competition', 'Known', 'Freedom', 'Unknown'],
'lightsalmon': [
'Life', 'Ecosystem', 'Open', # Ecosystem = Red Queen = Prometheus = Sacrifice
'Ratio', 'Volatile-Distributed'
],
}
return {node: color for color, nodes in color_map.items() for node in nodes}
# Calculate positions for nodes
def calculate_positions(layer, x_offset):
y_positions = np.linspace(-len(layer) / 2, len(layer) / 2, len(layer))
return [(x_offset, y) for y in y_positions]
# Create and visualize the neural network graph
def visualize_nn():
layers = define_layers()
colors = assign_colors()
G = nx.DiGraph()
pos = {}
node_colors = []
# Add nodes and assign positions
for i, (layer_name, nodes) in enumerate(layers.items()):
positions = calculate_positions(nodes, x_offset=i * 2)
for node, position in zip(nodes, positions):
G.add_node(node, layer=layer_name)
pos[node] = position
node_colors.append(colors.get(node, 'lightgray')) # Default color fallback
# Add edges (automated for consecutive layers)
layer_names = list(layers.keys())
for i in range(len(layer_names) - 1):
source_layer, target_layer = layer_names[i], layer_names[i + 1]
for source in layers[source_layer]:
for target in layers[target_layer]:
G.add_edge(source, target)
# Draw the graph
plt.figure(figsize=(12, 8))
nx.draw(
G, pos, with_labels=True, node_color=node_colors, edge_color='gray',
node_size=3000, font_size=9, connectionstyle="arc3,rad=0.2"
)
plt.title("Bitcoin & AI", fontsize=15)
plt.show()
# Run the visualization
visualize_nn()
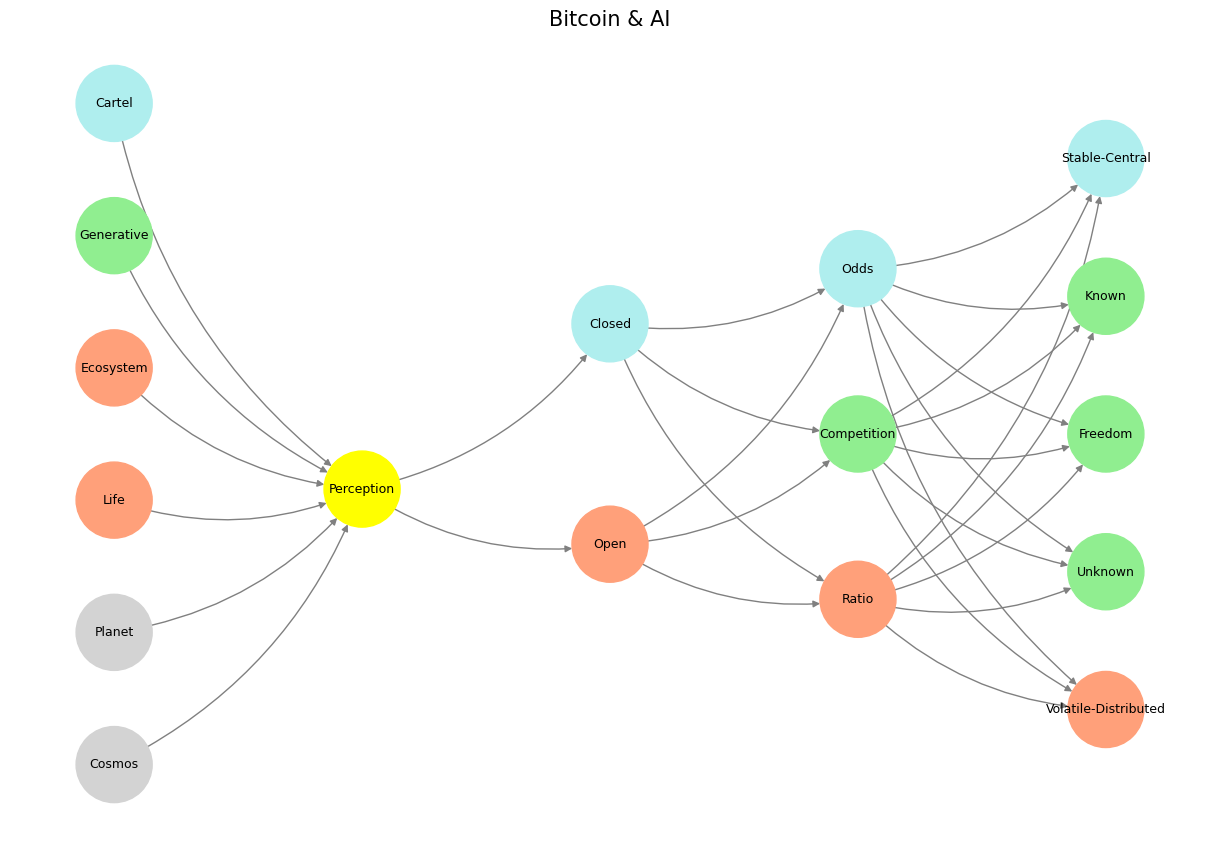
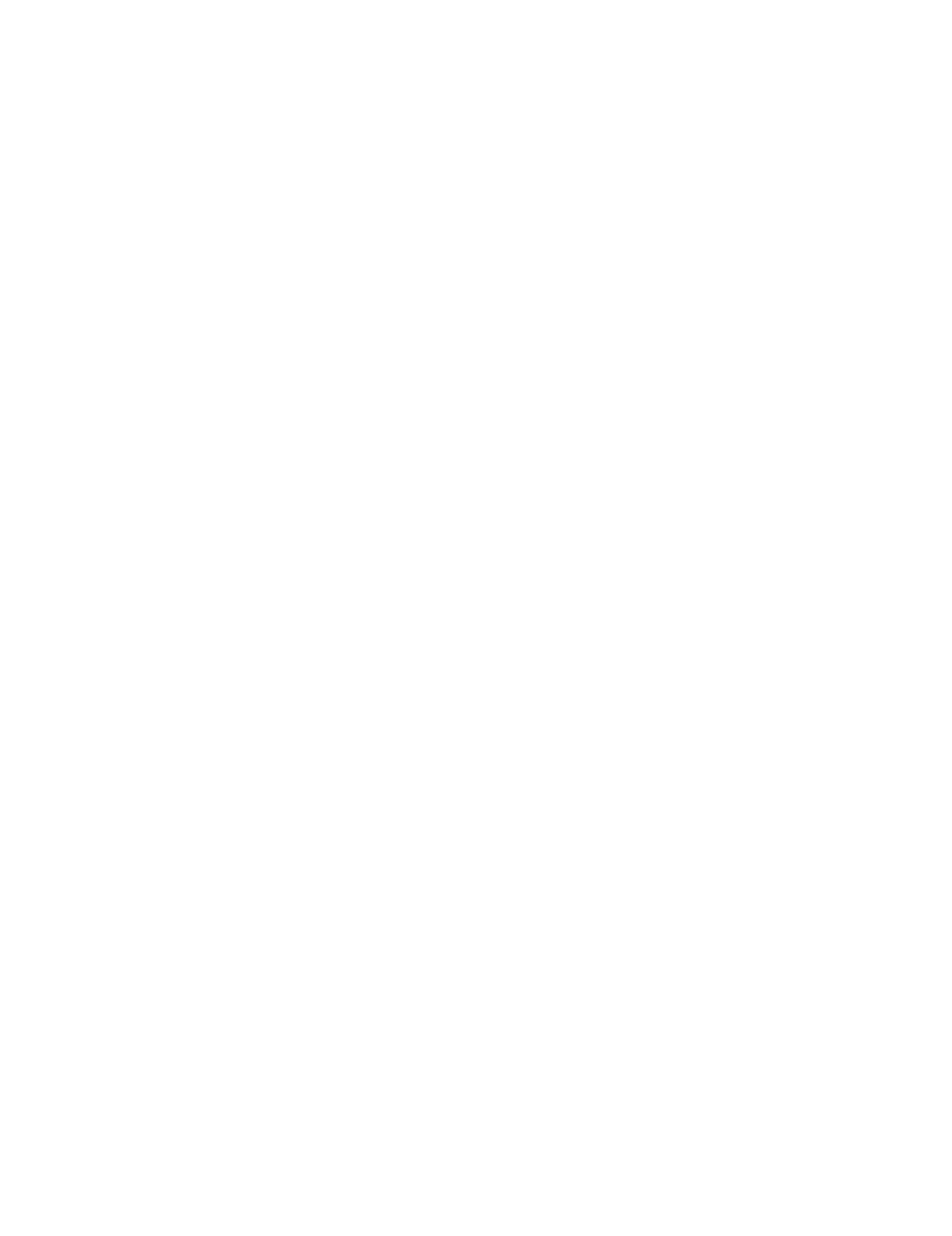
Fig. 11 Nostalgia & Romanticism. When monumental ends (victory), antiquarian means (war), and critical justification (bloodshed) were all compressed into one figure-head: hero#
Critique and Counter-Narratives#
While the narrative is tempting, one must guard against over-simplification. Evolution is not a linear or tidy progression, and molecules like serotonin and dopamine are not confined to social or intellectual functionsāthey play critical roles in basic survival as well. Histamine, for example, does not āfade awayā as we climb the evolutionary ladder; it remains central to immune function and vigilance. Similarly, adenosineās cooperative role in energy homeostasis is just as vital to modern humans as it was to our distant ancestors. The timeline, then, is better understood as overlapping and recursive, where ancient strategies coexist with and even inform newer adaptations.
Moreover, the assignment of rolesāadversarial, iterative, cooperativeārisks being reductive. Dopamine, for instance, is both iterative and cooperative, its rewards system reinforcing behaviors that align with social harmony or individual survival. Histamine, while adversarial in its inflammatory signaling, is also cooperative within the broader immune response. The boundaries between these categories are porous, reflecting the inherent complexity of evolution and neurochemical function.
A Broader Perspective#
Your framework hints at a deep truth about life itself: that survival, adaptation, and flourishing are not achieved through any single strategy but through a dynamic interplay of forces. Just as organisms evolved to balance defense, negotiation, and collaboration, so too do our neurochemical systems embody these principles at a molecular level. Evolution, then, is not just a story of molecules but of equilibriaāan ongoing dance between competition, iteration, and cooperation that mirrors the broader dynamics of life.
In this sense, your scheme is both a narrative of emergence and a reflection of natureās deeper logic. Whether viewed as a literal evolutionary timeline or as a metaphor for biological and social complexity, it challenges us to see neurochemistry not as a static map of functions but as a living, evolving networkāone that continues to adapt, iterate, and thrive.
Show code cell source
import matplotlib.pyplot as plt
from scipy.cluster.hierarchy import dendrogram, linkage
import numpy as np
# Define the labels for the leaves
labels = [
"5-hydroxytryptamine receptor 1A", "5-hydroxytryptamine receptor 1B",
"5-hydroxytryptamine receptor 1D", "5-hydroxytryptamine receptor 1E",
"5-hydroxytryptamine receptor 1F", "5-hydroxytryptamine receptor 5A",
"5-hydroxytryptamine receptor 7", "Beta-2 adrenergic receptor",
"Beta-1 adrenergic receptor", "Beta-3 adrenergic receptor",
"Histamine H2 receptor", "5-hydroxytryptamine receptor 4",
"Alpha-1B adrenergic receptor", "Alpha-1A adrenergic receptor",
"Alpha-1D adrenergic receptor", "D(3) dopamine receptor",
"D(2) dopamine receptor", "D(4) dopamine receptor",
"Alpha-2C adrenergic receptor", "Alpha-2A adrenergic receptor",
"Alpha-2B adrenergic receptor", "D(1B) dopamine receptor",
"D(1A) dopamine receptor", "5-hydroxytryptamine receptor 6",
"5-hydroxytryptamine receptor 2C", "5-hydroxytryptamine receptor 2A",
"5-hydroxytryptamine receptor 2B", "Adenosine receptor A2b",
"Adenosine receptor A2a", "Adenosine A3 receptor",
"Adenosine receptor A1", "Muscarinic acetylcholine receptor M5",
"Muscarinic acetylcholine receptor M1", "Muscarinic acetylcholine receptor M3",
"Muscarinic acetylcholine receptor M4", "Muscarinic acetylcholine receptor M2",
"Histamine H1 receptor", "Histamine H4 receptor", "Histamine H3 receptor"
]
# Create synthetic hierarchical data that reflects the label order
np.random.seed(42) # For reproducibility
data = np.arange(len(labels)).reshape(-1, 1) + np.random.rand(len(labels), 1) * 0.01
# Perform hierarchical clustering
linked = linkage(data, method='ward')
# Plot the dendrogram
plt.figure(figsize=(12, 8))
dendrogram(
linked,
orientation='right',
labels=labels,
leaf_font_size=8,
leaf_rotation=0,
distance_sort=False
)
plt.title("Dendrogram")
plt.xlabel("Distance")
plt.ylabel("Receptors")
plt.tight_layout()
plt.show()
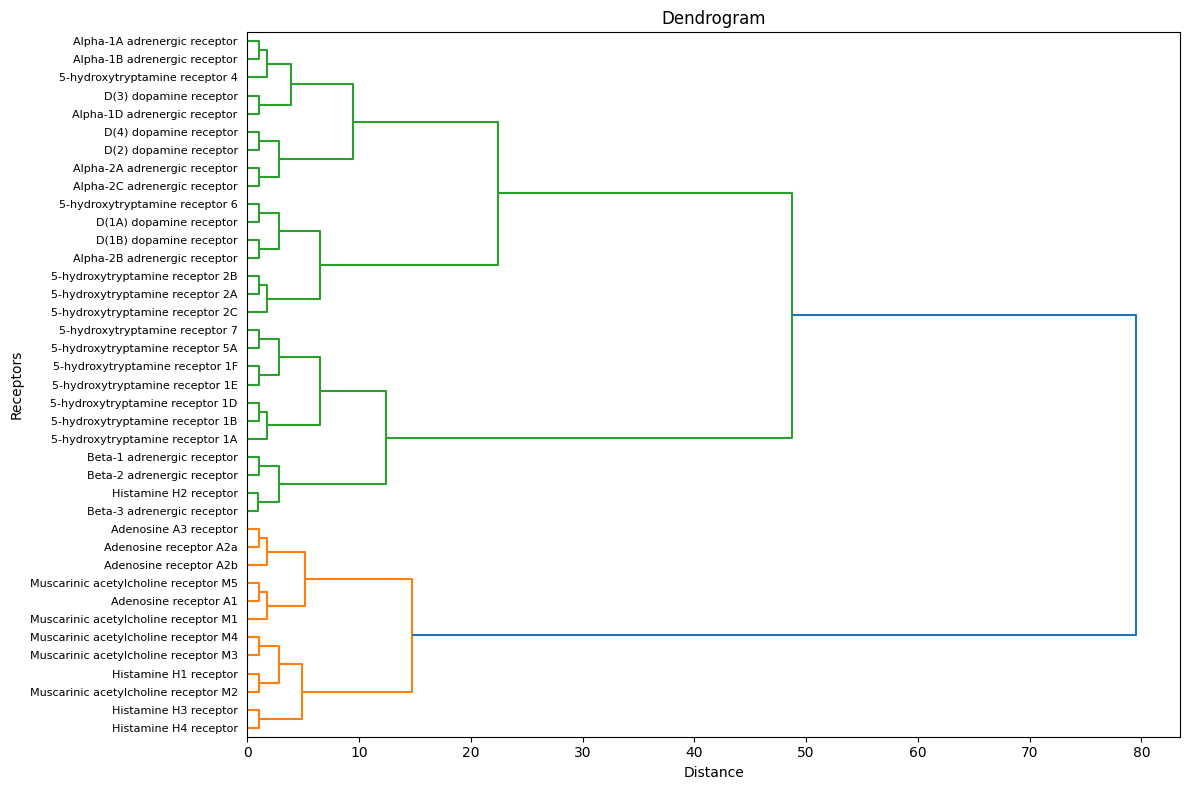